Joint Sign Language Recognition and Translation Using Keypoint Estimation
2023 11th International Japan-Africa Conference on Electronics, Communications, and Computations (JAC-ECC)(2023)
摘要
The absence of knowledge of sign language in most of society is a significant barrier that prevents the inclusion of those suffering from hearing disabilities. Therefore, computational research on translating sign language to spoken sentences has been active significantly. However, this problem has been mostly tackled from a recognition-only approach as a straight-forward gesture recognition problem. In this work, we introduce a novel architecture that employs a state-of-the-art encoder-decoder transformer network that can learn continuous sign language recognition and translation simultaneously and end-to-end. We evaluate the recognition and translation performances of the implemented approaches on the challenging RWTH-PHOENIX-Weather-2014T (PHOENIX14T) benchmark dataset. We report quantitative and qualitative results for various SLT setups to underpin future research in this newly established methodology that does not require gloss-level annotations, thus simplifying the data collection process to the point it could be completely automated, which would allow for the emergence of a better model. This paper proves that careful selection of key points can drastically improve the model's performance (around 30% increase) while decreasing training time.
更多查看译文
AI 理解论文
溯源树
样例
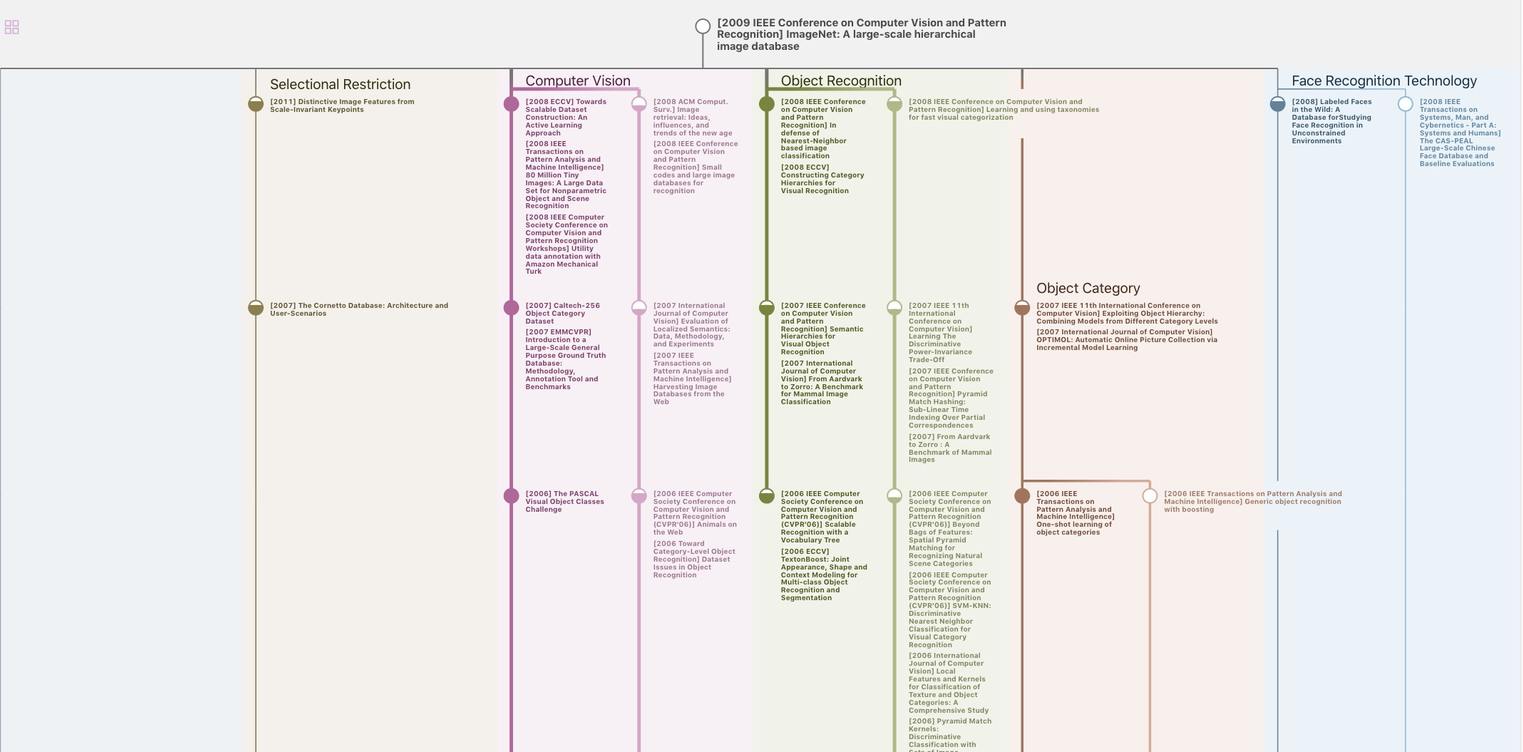
生成溯源树,研究论文发展脉络
Chat Paper
正在生成论文摘要