Meta-Learning-based Cross-Dataset Motor Imagery Brain-Computer Interface.
International Winter Conference on Brain-Computer Interface(2024)
摘要
Motor Imagery Brain-Computer Interface (MI-BCI) facilitates human to communicate with computers or machines using brain signals, such as electroencephalography (EEG), induced by the imagination of body movements. However, acquiring sufficient data for training reliable classification model is often time-consuming and impractical. Consequently, recent studies have shifted focus to subject-independent EEG classification, leveraging data from other subjects by using methodologies like transfer learning or meta-learning. However, most of the studies exploit the subjects within the same dataset, which might raise challenges especially when data from other subjects are scarce or inaccessible. To address this issue, we propose a meta-learning-based cross-dataset transfer learning for MI EEG classification. We first extract informative knowledge from the source dataset based on the meta-learning framework. We then leverage the extracted knowledge (or meta-parameters) to enhance the classification performance of the target dataset. This method leverages the BCI Competition IV-2a dataset as the source and the KU and GIST datasets as the target dataset, respectively. Our experimental results indicate that the proposed method enhances MI EEG classification performance compared to conventional subject-dependent scenarios.
更多查看译文
AI 理解论文
溯源树
样例
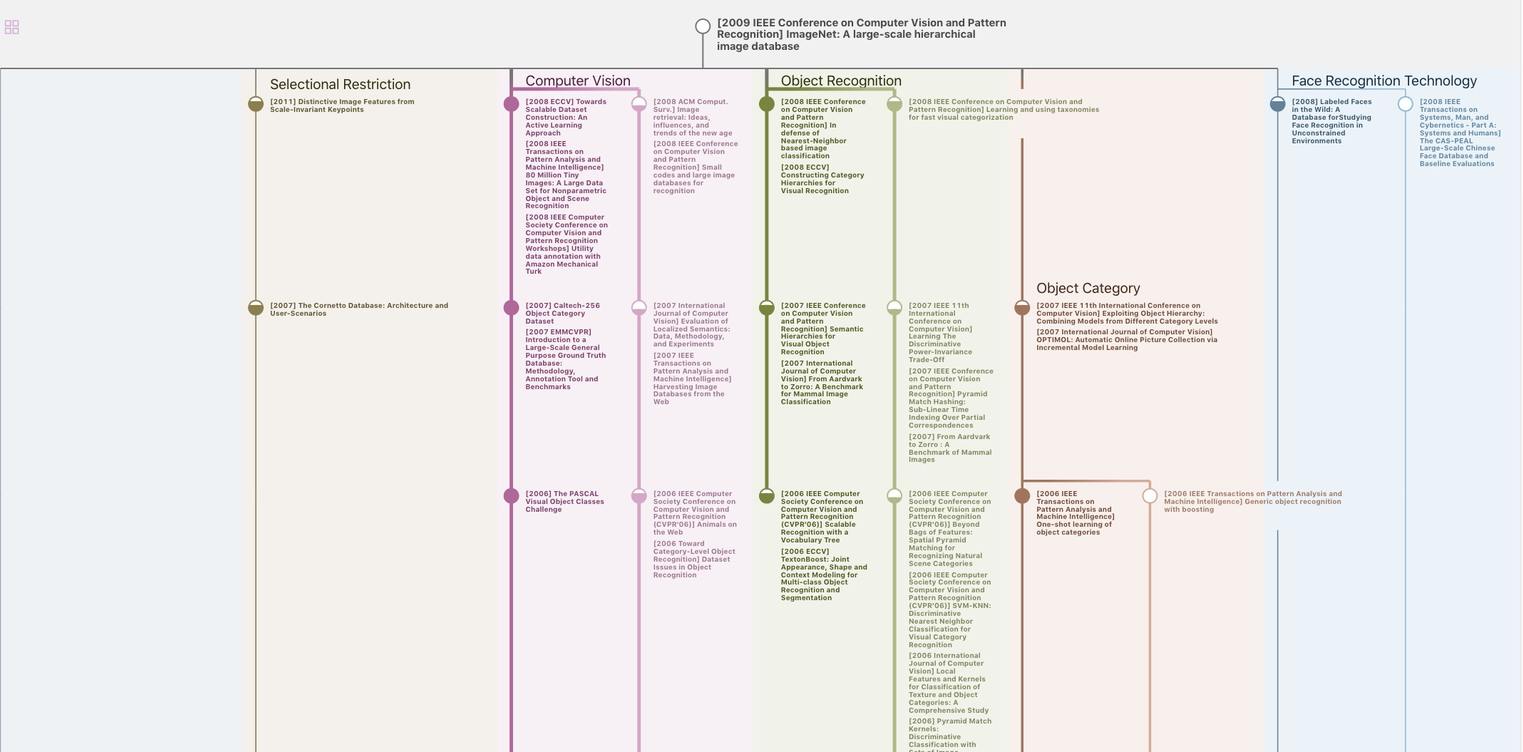
生成溯源树,研究论文发展脉络
Chat Paper
正在生成论文摘要