Battery prognostics using statistical features from partial voltage information
MECHANICAL SYSTEMS AND SIGNAL PROCESSING(2024)
摘要
Accurately estimating battery health status is crucial to ensure safe battery operation. The datadriven estimation methods proposed in current literature typically require complete sets of voltage, current, temperature data throughout battery charge/discharge process. However, obtaining such comprehensive data in real-world applications is often impractical. In this paper, we propose to estimate the health status of commercial lithium-ion batteries based on statistical features derived from voltage data within a specific voltage interval. Our emphasis on voltage data is grounded in the wealth of information voltage curves usually provide for battery prognostics. To implement our approach, the time-sampled battery data is first converted into voltagesampled data series via resampling using cubic splines. Then, a set of statistical features is constructed based on Delta Q(V) statistics and incremental capacity analysis, aiming to capture more effective voltage information from both cycle and discharge time dimensions. The extracted features are then studied considering battery degradation mechanisms, different battery materials, and the choice of voltage interval. The proposed methodology is tested on both lithium iron phosphate batteries and lithium nickel manganese cobalt oxide batteries, and experimental results demonstrate that the Delta Q(V) statistics perform more reliably and robustly than incremental capacity features. In addition, the mean, minimum, and variance are highly correlated with battery capacity, while higher-order moments such as skewness and kurtosis present insignificant impacts. Compared to estimation based on all discharge data, estimation based on partial voltage information yield very competitive results, with all root mean square errors less than 1%.
更多查看译文
关键词
Lithium-ion batteries,Feature extraction,State-of-health estimation,Remaining useful life prediction,Machine learning
AI 理解论文
溯源树
样例
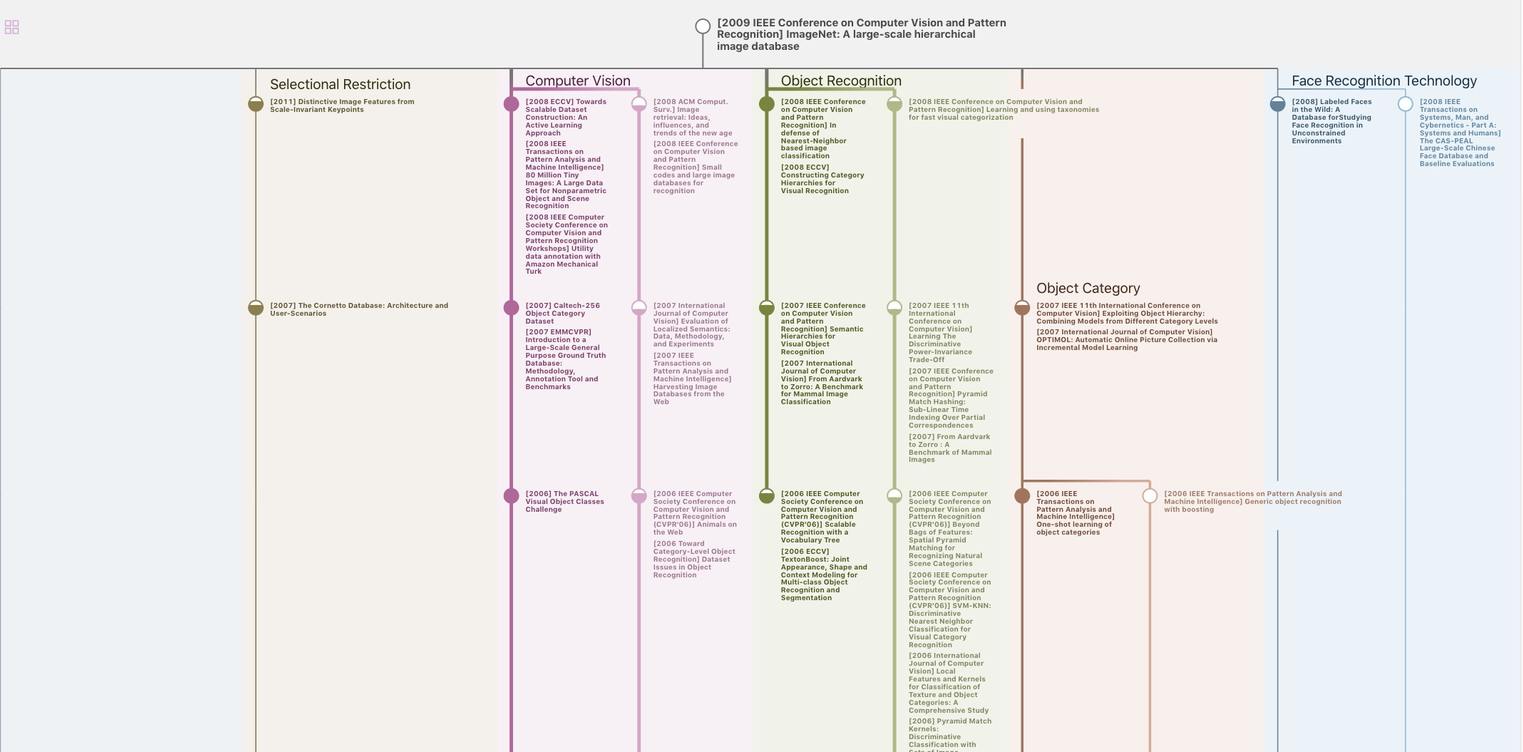
生成溯源树,研究论文发展脉络
Chat Paper
正在生成论文摘要