Fnhancing Social Recommendation with Multi -View BERT Network
23RD IEEE INTERNATIONAL CONFERENCE ON DATA MINING, ICDM 2023(2023)
摘要
With the emergence of online social platforms enabling users to share their opinions with others, there has been a critical need for developing a recommendation system incorporating users' social connections to learn their preferences. Social relations between users offer potential information about users' preferences, which alleviates the data sparsity issue and boosts recommendation performance. Several efforts have been made to develop an efficient social recommendation system. Nevertheless, existing approaches have two significant drawbacks: First, they haven't efficiently explored the complex correlations between the diverse influence of neighbours on users' item preferences. Second, contemporary systems rely on the unidirectional context of items and fail to embrace bidirectional contexts and long range dependencies while predicting the next user-item interaction. To address the above issues, we propose a novel framework for Social Recommendation called Multi-View Bert Network (MVBN). This model incorporated Bidirectional Encoder Representations from Transformer(BERT) with MultiTask learning to learn bidirectional context-aware user and item embeddings with neighbourhood sampling. Neighbourhood Sampling technique samples the most influential neighbours for all the items, and our proposed Sequence [leader correlates sampled neighbours with users. The main objective of our model is to predict the next item that a user would interact with based on its interaction behaviour. Experiments on three real-world dataset.s demonstrate that the proposed MVBN model outperforms the state-of-the-art recommendation methods consistently and significantly. Finally, an extensive ablation study is carried out to validate the importance of each component in the system.
更多查看译文
关键词
Reconm endation Systems,Social Reconm endations,Transformers,BERT,Multi-Task learning
AI 理解论文
溯源树
样例
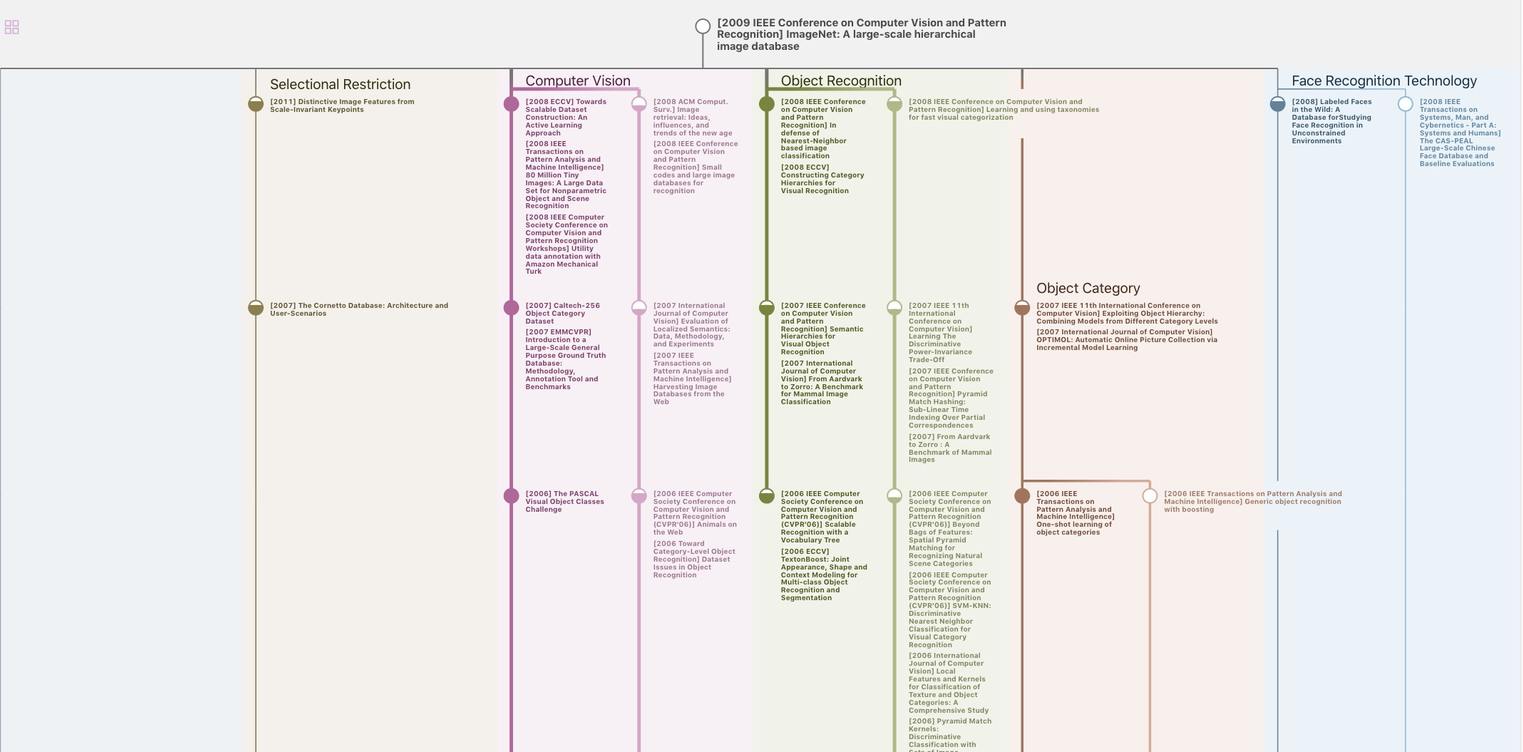
生成溯源树,研究论文发展脉络
Chat Paper
正在生成论文摘要