Traffic Flow Prediction Based on Interactive Dynamic Spatio-Temporal Graph Convolution with a Probabilistic Sparse Attention Mechanism
TRANSPORTATION RESEARCH RECORD(2024)
摘要
Accurate traffic flow prediction is of great practical significance to alleviate road congestion. Existing methods ignore the hidden dynamic associations between road nodes, and for the problem of difficulty capturing the dynamic spatio-temporal features of traffic flow in the prediction process, a novel model based on the interactive dynamic spatio-temporal graph convolutional probabilistic sparse attention mechanism (IDG-PSAtt) is proposed, which consists of an interactive dynamic graph convolutional network (IDGCN) structure with a spatio-temporal convolutional block (ST-Conv block) and a probabilistic sparse self-attention mechanism block (ProbSSAtt block). Among them, the IDGCN synchronizes the dynamic spatio-temporal features captured by interaction sharing, and the ST-Conv block is combined with the ProbSSAtt block to effectively capture the long short-term temporal features of the traffic flow. In addition, to effectively find the hidden dynamic associations between road network nodes, a dynamic graph convolutional network generated by the fusion of an adaptive neighbor matrix and a learnable neighbor matrix was constructed. Experimental results demonstrate that the prediction performance of the IDG-PSAtt model outperforms the baseline model under the evaluation criteria and experimental settings given in this paper. In the PEMS-BAY dataset, the mean absolute error and root mean square error of the IDG-PSAtt to 60 min are improved by 15.49% and 12.10%, compared with the state-of-the-art model, respectively.
更多查看译文
关键词
data and data science,artificial intelligence and advanced computing applications,deep learning,machine learning (artificial intelligence),neural networks
AI 理解论文
溯源树
样例
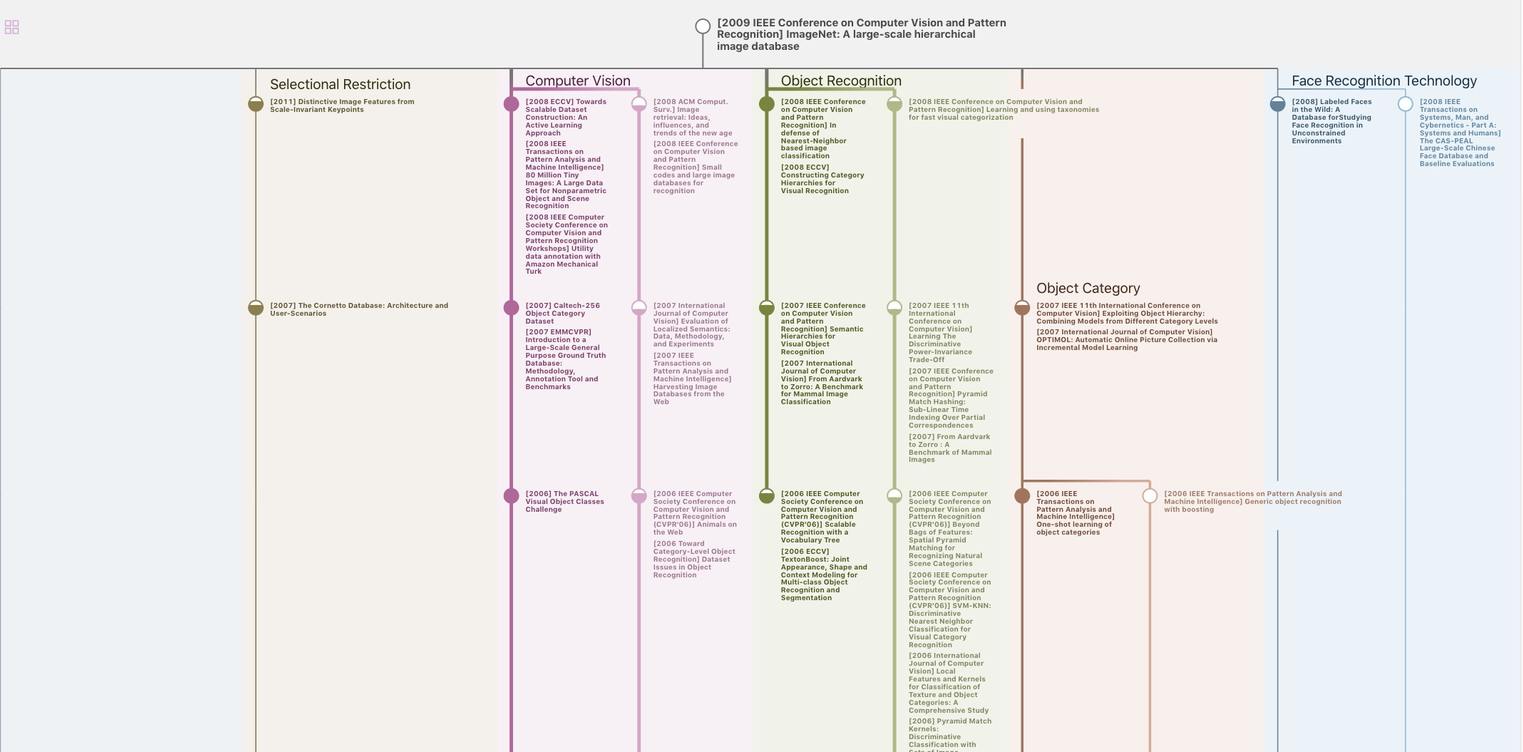
生成溯源树,研究论文发展脉络
Chat Paper
正在生成论文摘要