RLAfford: End-to-End Affordance Learning for Robotic Manipulation
2023 IEEE INTERNATIONAL CONFERENCE ON ROBOTICS AND AUTOMATION, ICRA(2023)
摘要
Learning to manipulate 3D objects in an interactive environment has been a challenging problem in Reinforcement Learning (RL). In particular, it is hard to train a policy that can generalize over objects with different semantic categories, diverse shape geometry and versatile functionality. In this study, we focused on the contact information in manipulation processes, and proposed a unified representation for critical interactions to describe different kinds of manipulation tasks. Specifically, we take advantage of the contact information generated during the RL training process and employ it as unified visual representation to predict contact map of interest. Such representation leads to an end-to-end learning framework that combined affordance based and RL based methods for the first time. Our unified framework can generalize over different types of manipulation tasks. Surprisingly, the effectiveness of such framework holds even under the multi-stage and multi-agent scenarios. We tested our method on eight types of manipulation tasks. Results showed that our methods outperform baseline algorithms, including visual affordance methods and RL methods, by a large margin on the success rate. The demonstration can be found at https://sites.google.com/view/rlafford/.
更多查看译文
AI 理解论文
溯源树
样例
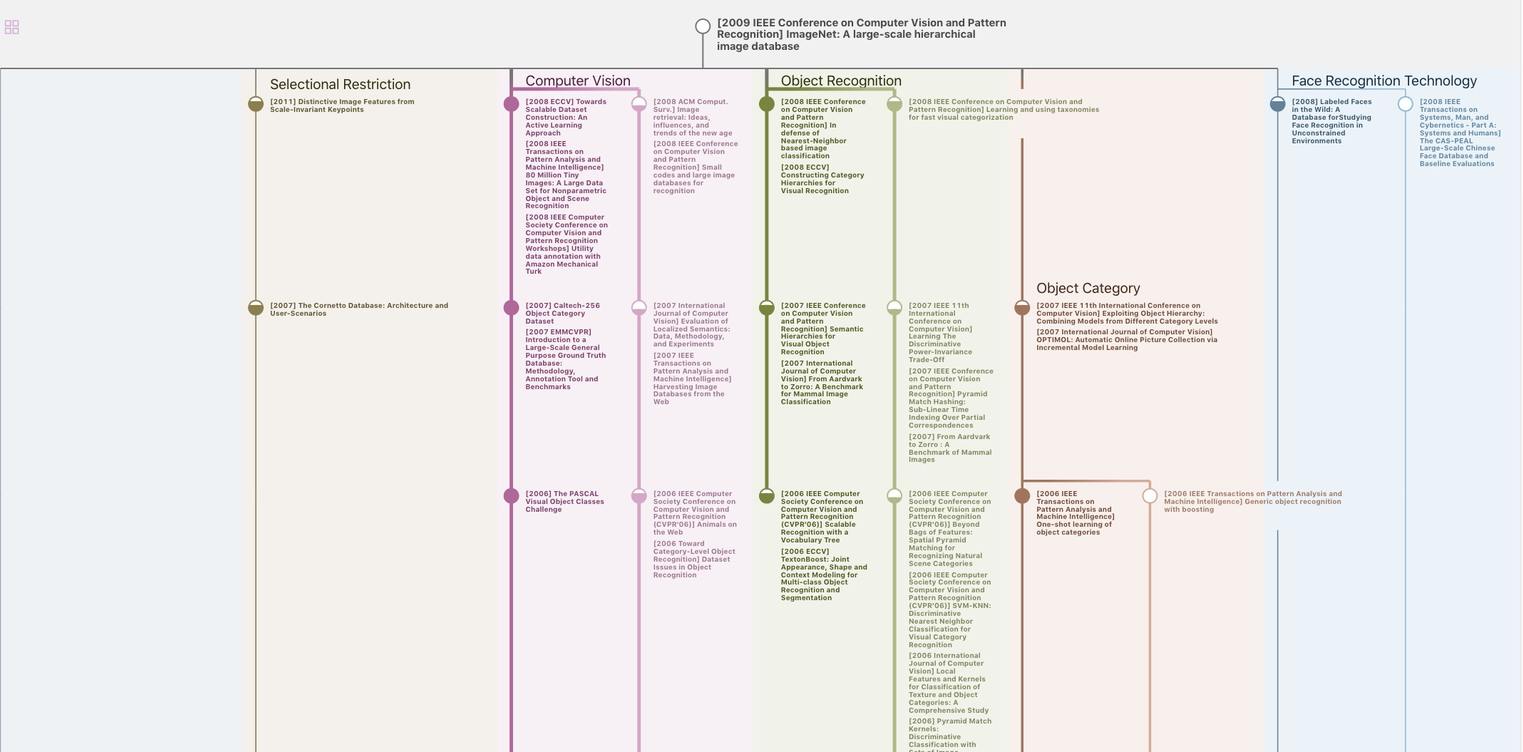
生成溯源树,研究论文发展脉络
Chat Paper
正在生成论文摘要