Joint Exploitation of Coherent Change Detection and Global-Context Capturing Network for Subtle Changed Track Detection with Airborne SAR
IEEE Journal of Selected Topics in Applied Earth Observations and Remote Sensing(2024)
摘要
Change detection is a crucial remote sensing application because it can locate the interesting changed regions and provide corresponding time-series information with multitemporal remote sensing images acquired in the same region. Synthetic aperture radar (SAR), with the advantages of all-day and all-weather conditions, can achieve high-resolution imaging from long operation distances. Moreover, the coherence imaging characteristics of the SAR system cause the attained complex-value images to be more sensitive to ground surface features. Traditional intensity-based change detection methods merely use the intensity difference between multitemporal images; thus, the results only reflect the significant landmark changes, such as seismic disasters and flood disasters. In this study, we use the coherent imaging characteristics of the SAR complex images to detect very subtle changes, such as vehicle tracks and footprints. Specifically, coherent change detection based on amplitude and phase generates a difference image utilizing the repeat-pass repeat-geometry SAR images, while the global attention-based convolutional neural network achieves automatic subtle change track extraction from the difference images. The experimental results based on our measured airborne SAR data demonstrate that our proposed method reduces the sensitivity of the detectable changed region from the meter level to the centimeter level, further providing our method with the ability to detect very subtle changes, such as vehicle tire tracks or footprints by human activities. This subtle change detection capability can be used for the search and rescue of vehicles and personnel lost in the field.
更多查看译文
关键词
Synthetic Aperture Radar (SAR),Coherence Change Detection (CCD),Convolutional Neural Network (CNN),UNet
AI 理解论文
溯源树
样例
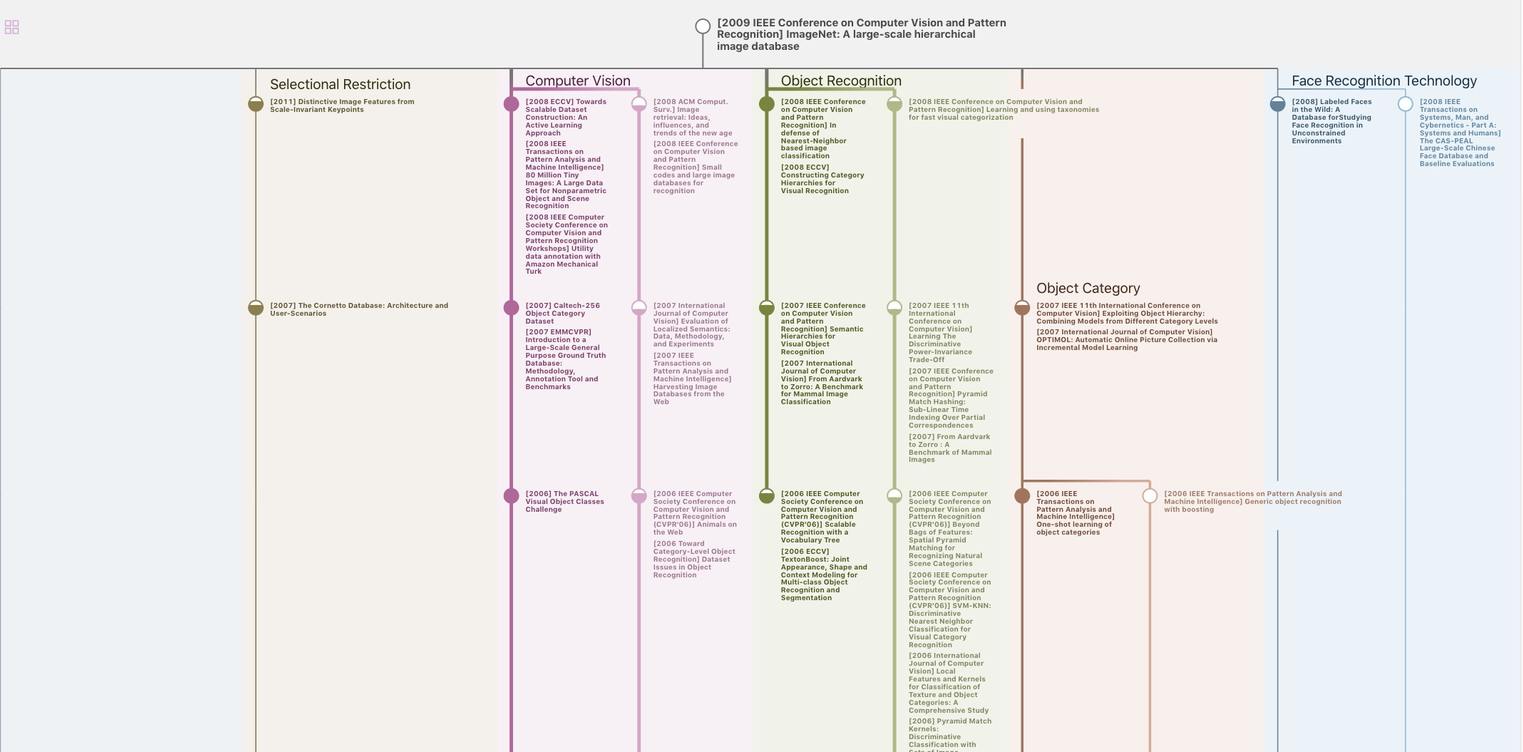
生成溯源树,研究论文发展脉络
Chat Paper
正在生成论文摘要