Efficient Model-Relational Data Management: Challenges and Opportunities
IEEE Transactions on Knowledge and Data Engineering(2024)
摘要
As modern data pipelines continue to collect, produce, and store various data formats, extracting and combining value from traditional and context-rich sources becomes unsuitable for RDBMS. To tap into the dark data, domain experts analyze and extract insights and integrate them into various data repositories. This can involve out-of-DBMS processing with high manual effort and suboptimal performance. While AI systems based on ML models can automate the analysis, they can further generate context-rich answers. Using multiple data sources and models further exacerbates the problem of consolidating and analyzing the data of interest. We envision an analytical engine co-optimized with components that enable context-rich analysis. Firstly, as all the data from different sources is expensive to clean ahead of time, we propose using online data integration via model-assisted similarity operations. Secondly, we aim for a holistic pipeline cost- and rule-based optimization across relational and model-based operators. Thirdly, with increasingly heterogeneous hardware and workloads ranging from relational analytics to generative model inference, we envision a system that adapts to the complex query requirements at runtime. Composing ML-driven insights with established approaches aims to expand decades of research and systems-building effort in making complex functionality and performance effortless for the end user.
更多查看译文
关键词
analytics,machine learning,data integration,query optimization,vector data,hardware-conscious processing
AI 理解论文
溯源树
样例
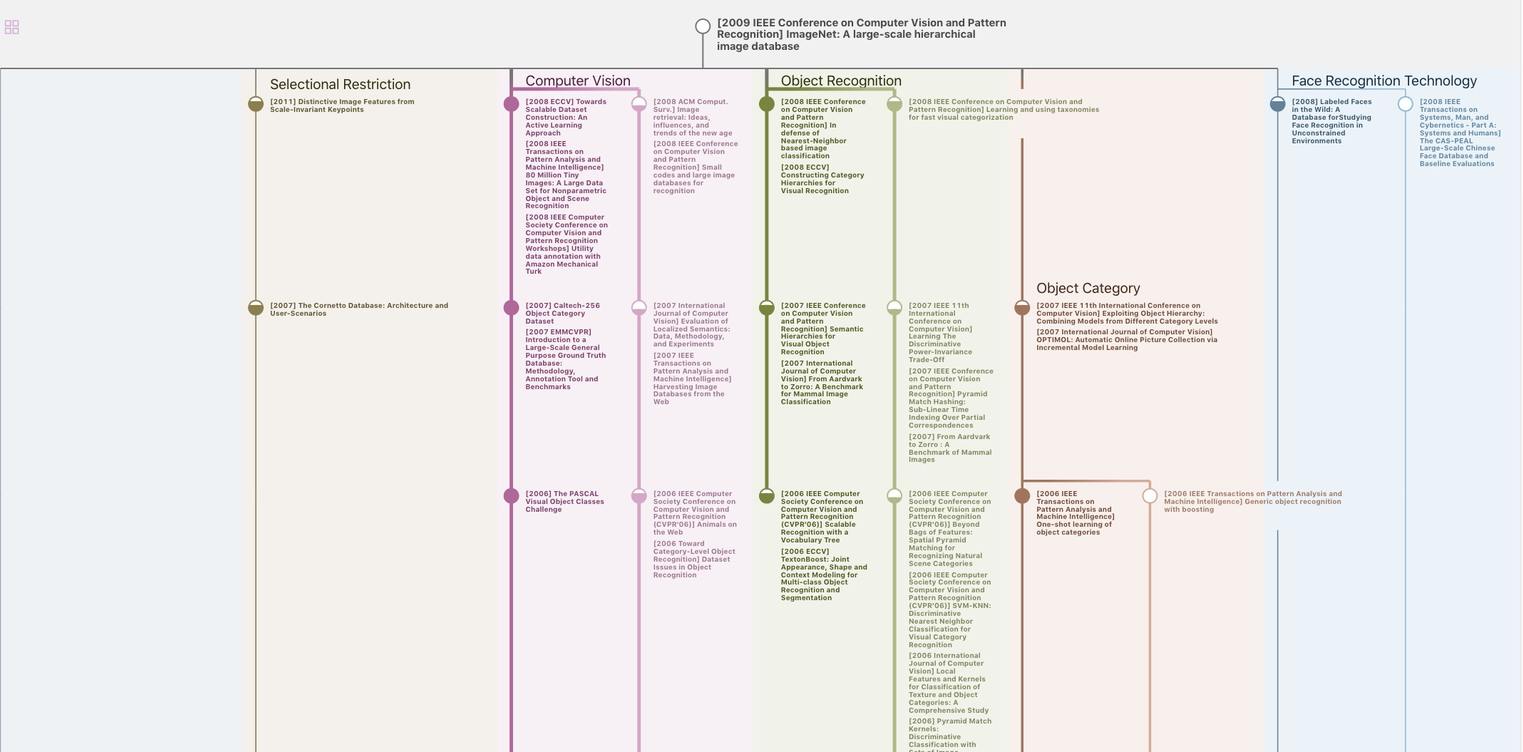
生成溯源树,研究论文发展脉络
Chat Paper
正在生成论文摘要