Multilevel State-Space Models Enable High Precision Event Related Potential Analysis.
Asilomar Conference on Signals, Systems and Computers(2023)
摘要
During cognitive tasks, the elicited brain responses that are time-locked to the stimulus presentation are manifested in electroencephalogram (EEG) as Event Related Potentials (ERPs). In general, ERPs are
$\sim 1\mu \mathrm{V}$
signals embedded in the background of much stronger neural oscillations, and thus they are traditionally extracted by averaging hundreds of trial responses so that the neural oscillations can cancel out each other. However, often in cognitive science experiments, it is difficult to administer large number of trials due to physical constraints. Additionally, these excessive averaging can also blur fine structures of the ERPs signals, which might otherwise be indicative of various intrinsic factors. Here we propose to model the background oscillations using a novel oscillation state-space representation and identify their time-traces in a data-driven way. This allows us to effectively separate the oscillations from the response signals of interest, thus improving the signal-to-noise of the evoked response, and eventually increasing trial fidelity. We also consider a random-walk like continuity constraint for the ERP waveforms to recover smooth, de-noised estimates. We employ a generalized expectation maximization algorithm for estimating the model parameters, and then infer the approximate posterior distribution of ERP waveforms. We demonstrate the reduced reliance of our proposed ERP extraction technique via a simulation study. Finally, we showcase how the extracted ERPs using our method can be more informative than the traditional average-based ERPs when analyzing EEG data in cognitive task settings with fewer trials.
更多查看译文
关键词
Event related potentials,neural oscillations,state-space model,variational approximation
AI 理解论文
溯源树
样例
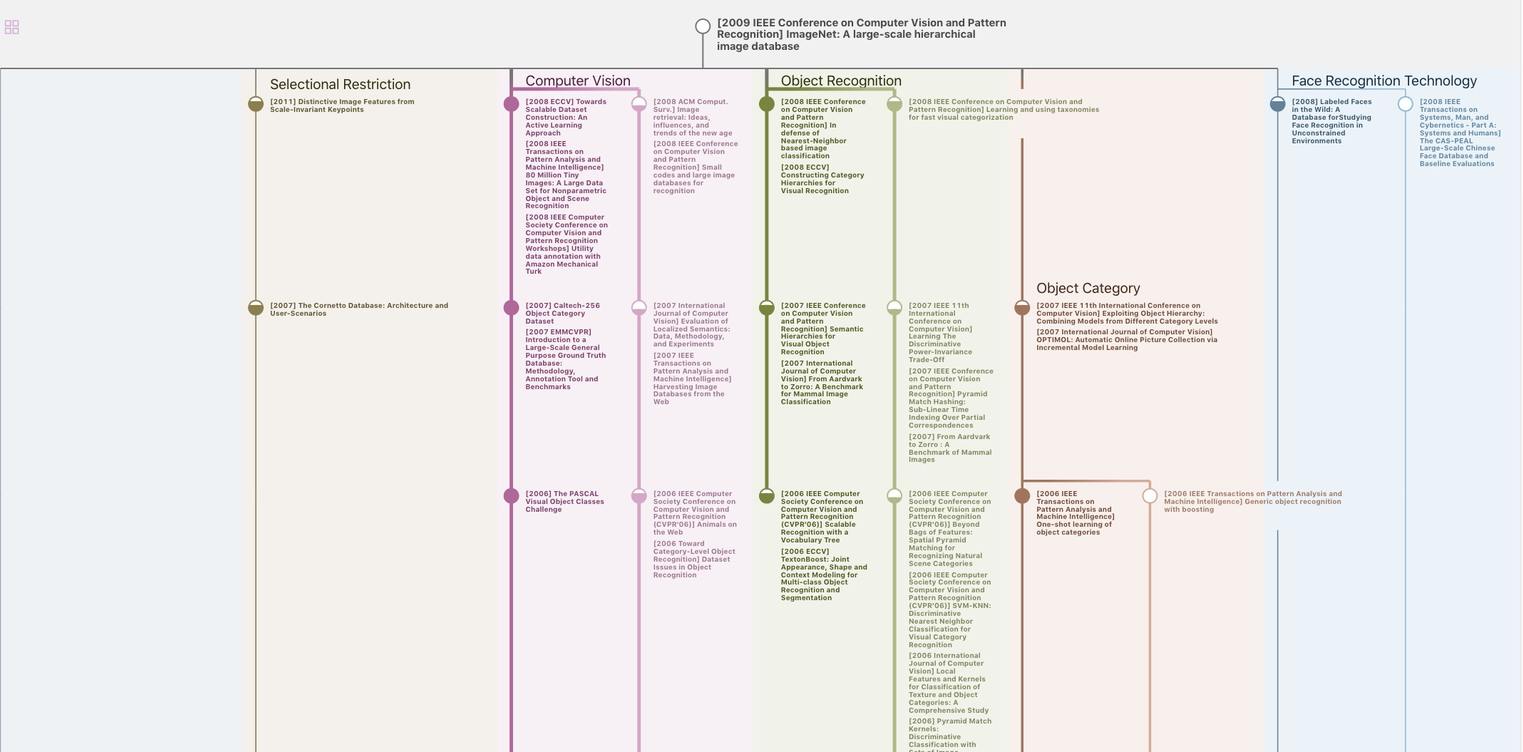
生成溯源树,研究论文发展脉络
Chat Paper
正在生成论文摘要