Convergence of Graph Neural Networks on Relatively Sparse Graphs.
Asilomar Conference on Signals, Systems and Computers(2023)
摘要
In this paper we study the connection between graph filters, graph neural networks (GNNs) and manifold filters, manifold neural networks (MNNs), Specifically, we consider the case when we have access to a set of uniformly sampled points from the manifold based on which we construct a relatively sparse graph to approximate the manifold, which is a suitable model for many real world applications. We prove a non-asymptotic approximation error bound or convergence rate for the graph filters and the GNNs on the relatively sparse graphs to the filters and neural networks on the manifold that the graphs are sampled from. An interesting trade-off between the convergence and the discriminability of the graph filters can be observed from the non-asymptotic error bound which indicates that graph filters cannot give good convergence and discriminability at the same time. While the nonlinearity function in GNNs can alleviate this trade-off and allows the GNNs to both converge to the MNNs and discriminate well. Equipped with this non-asymptotic error bound, we further interpret the transferability property of GNNs when the graphs are sampled from a common manifold. We verify our conclusions with a point-cloud classification problem.
更多查看译文
关键词
Graph neural networks,manifold convolution,manifold neural networks,relatively sparse graphs,convergence rate,transferability analysis
AI 理解论文
溯源树
样例
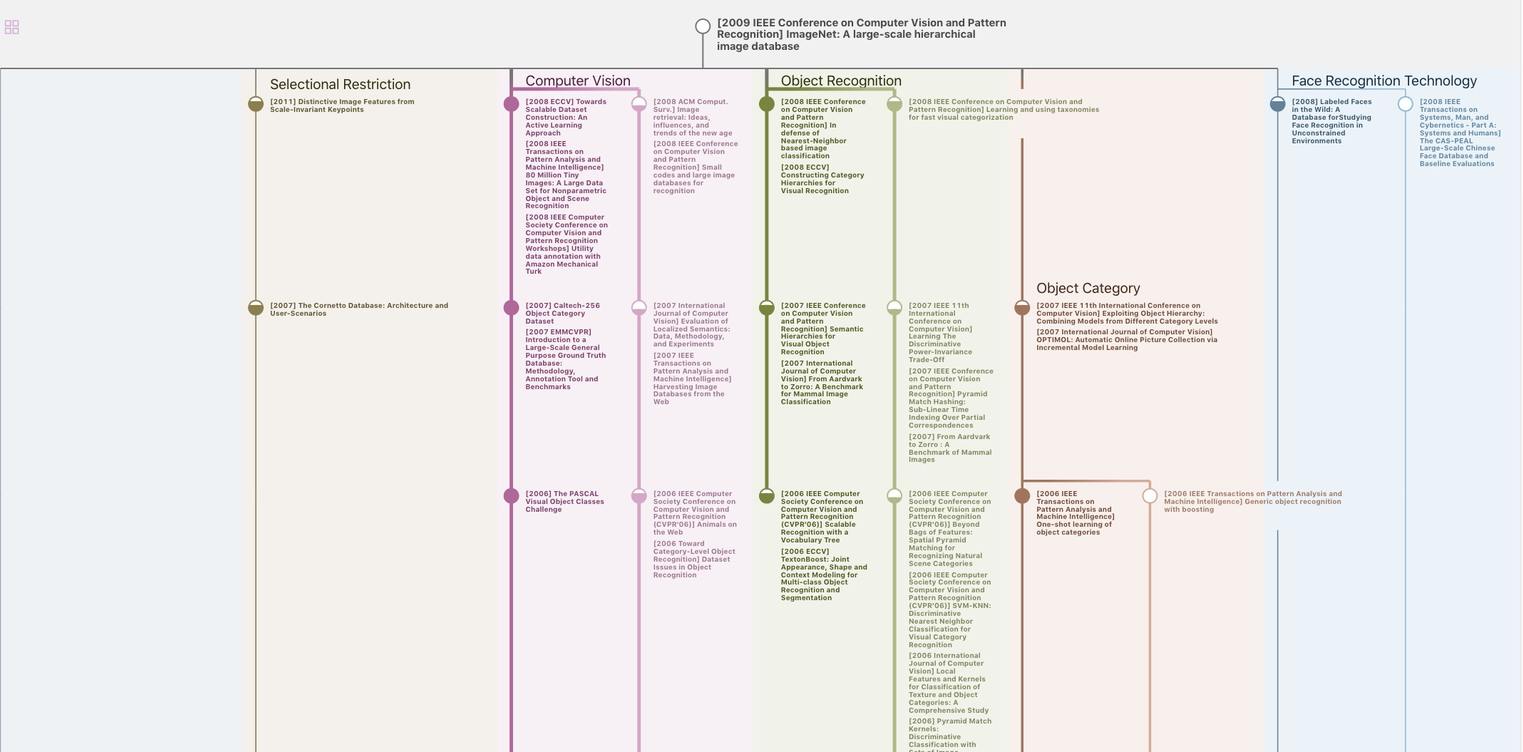
生成溯源树,研究论文发展脉络
Chat Paper
正在生成论文摘要