Adaptive Fourier Convolution Network for Road Segmentation in Remote Sensing Images.
IEEE Trans. Geosci. Remote. Sens.(2024)
摘要
Segmentation of roads in remote sensing images is a challenging task due to the inhomogeneous intensity, non-consistent contrast, and very cluttered background in remote sensing images. Recent approaches, mostly relying on convolutions or self-attention, make it difficult to extract weak and continuous road objects. Fourier neural operators provide another novel mechanism for capturing long-range and fine-grained features beyond self-attention. Based on it, we propose an adaptive Fourier convolution network (AFCNet) on the spatial-spectral domain for road segmentation in this paper. The AFCNet is built on the pipeline of the classical U-Net model and its core is the proposed Fourier neural encoder (FNE), which is built on a feed-forward layer and a flexible Fourier convolutional structure composed of Fourier-domain pooling layers, asymmetric convolutions, squeeze-excitation inspired self-attention and adaptive multiscale fusion layers. Furthermore, we combine the FNE and bottleneck in ResNet to form a hybrid global-local feature representation scheme to capture the long and weak road objects in remote sensing images. The experiments on two public datasets, the Massachusetts Roads and DeepGlobe Road Datasets, have shown that AFCNet worked with fewer parameters and outperformed most previous methods in terms of accuracy, precision, recall, and mean intersection over union (mIoU), etc.
更多查看译文
关键词
Fourier asymmetric convolution,adaptive Fourier convolution,Fourier neural encoder,road segmentation,U-Net
AI 理解论文
溯源树
样例
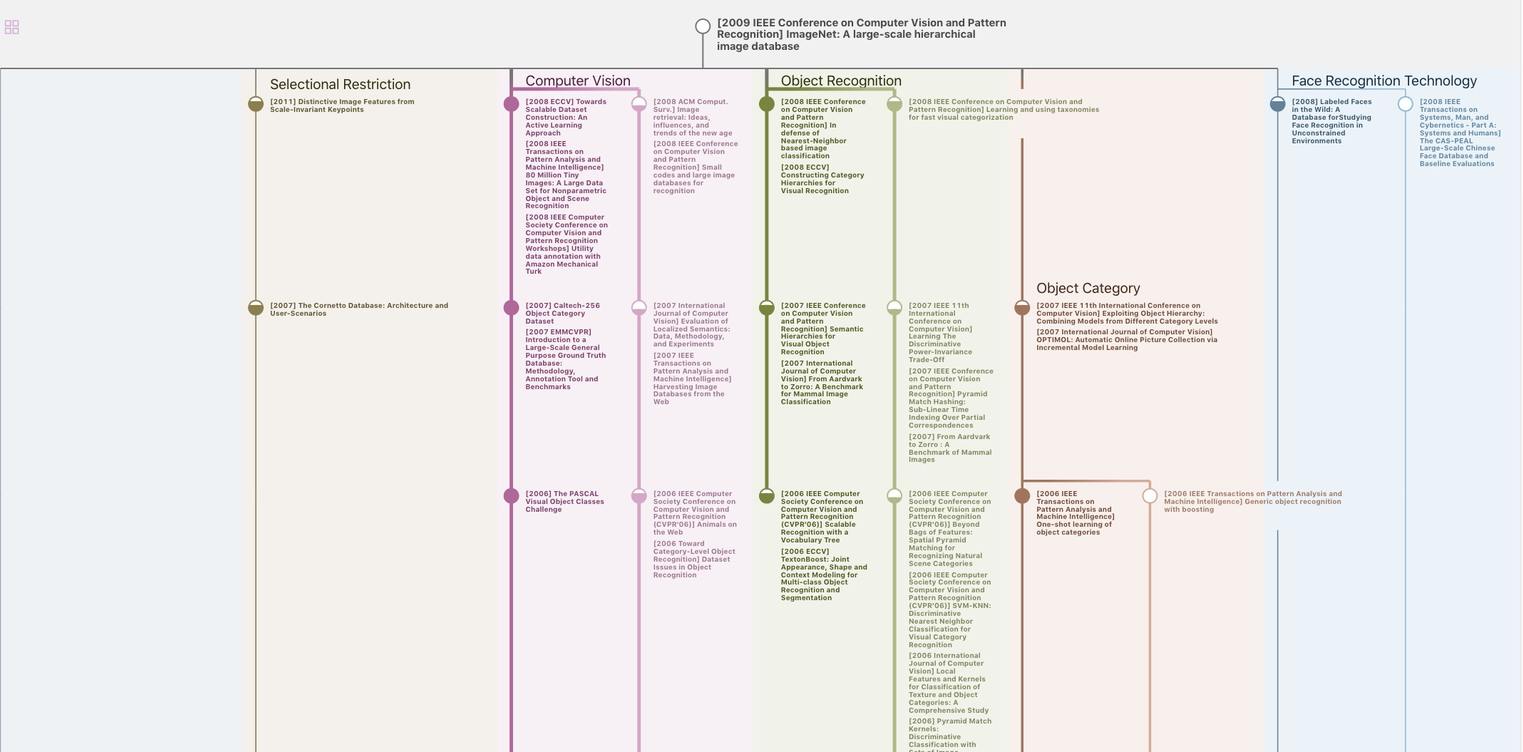
生成溯源树,研究论文发展脉络
Chat Paper
正在生成论文摘要