Inverse stochastic microstructure design
Acta Materialia(2024)
摘要
Inverse Microstructure Design problems are ubiquitous in materials science; for example, property-driven microstructure design requires the inversion of a structure–property linkage. However, prior frameworks have struggled to address this problem’s unique combination of challenges: the high dimensionality and stochasticity of microstructures, under sampled initial datasets, and ill-conditioning of the inversion. In this work, we propose a computational framework for Inverse Microstructure Design problems using a Bayesian methodology. We construct this framework from three modular components, enabling flexible extension and re-use. First, we define a low-dimensional, informative microstructure prior by integrating domain knowledge (i.e., statistical continuum mechanics) into a distributional learning scheme. This scheme includes multiple latent representations which address the challenges inherent to representing microstructures. Second, we define a property-specific likelihood using a multi-output Gaussian process regression surrogate model. Finally, we efficiently learn the conditional posterior density for a given target property, and generate samples using deep variational inference. We demonstrate our proposed method for solving stochastic microstructure design problems by identifying woven ceramic matrix composites matching target anisotropic thermal conductivities. Through this example, we analyze the integral role of each component in the inversion framework.
更多查看译文
关键词
Inverse design,Generative modeling,Uncertainty quantification,Bayesian inference,Computational materials design,Microstructure
AI 理解论文
溯源树
样例
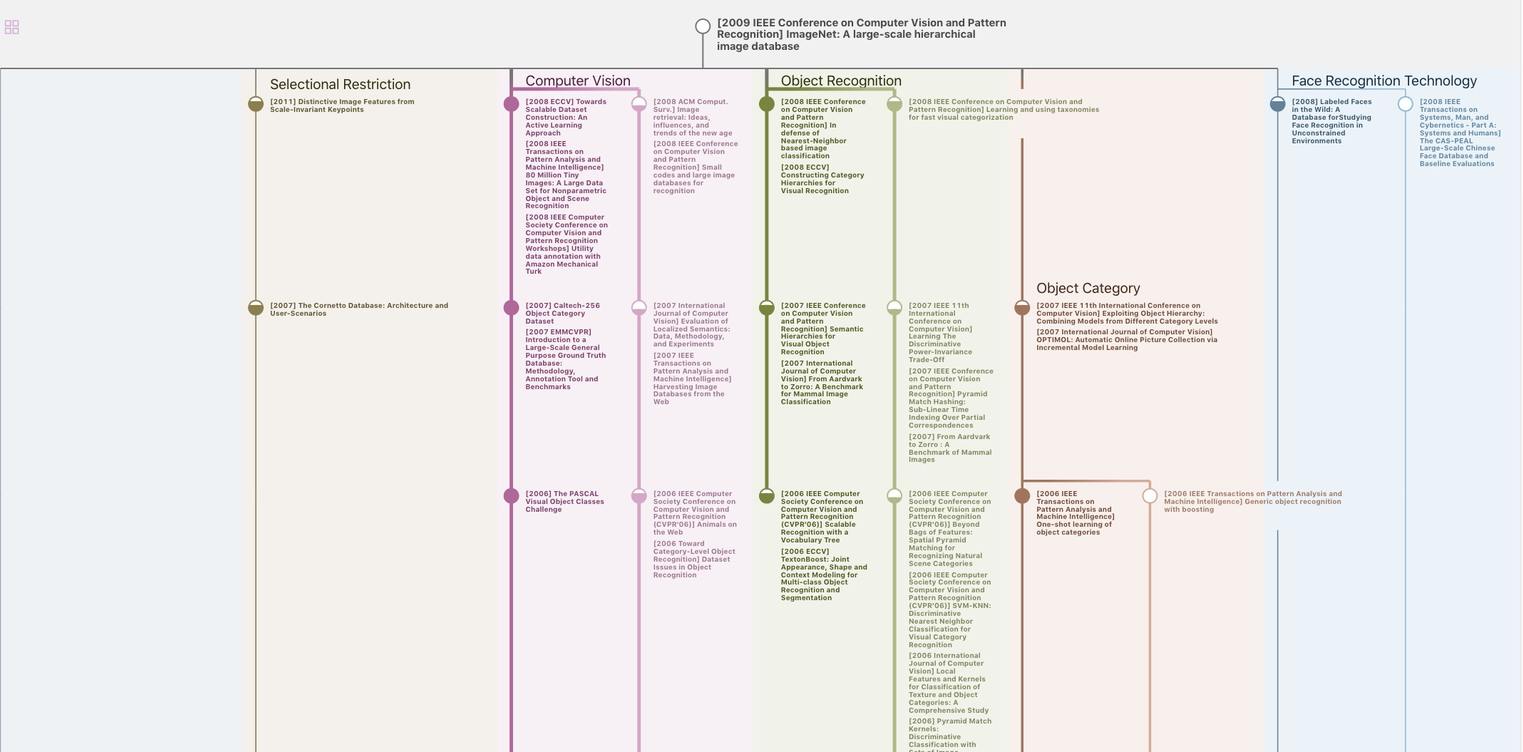
生成溯源树,研究论文发展脉络
Chat Paper
正在生成论文摘要