A Multi-Scale Forest Above-Ground Biomass Mapping Approach: Employing a Step-by-Step Spatial Downscaling Method with Bias-Corrected Ensemble Machine Learning
REMOTE SENSING(2024)
摘要
The accurate estimation of forest above-ground biomass (AGB) is vital for monitoring changes in forest carbon sinks. However, the spatial heterogeneity of AGB, coupled with inherent uncertainties, poses challenges in acquiring high-quality AGBs. This study introduced a bias-corrected ensemble machine learning (ML) algorithm for AGB downscaling that integrated a ML for AGB mapping with another for residual mapping. The accuracies of six bias-corrected ensemble ML algorithms were evaluated at resolutions of 0.05 degrees, 0.025 degrees, and 0.01 degrees. Moreover, a step-by-step downscaling (SBSD) method was introduced, utilizing bias-corrected ensemble ML algorithms to downscale AGB from 0.1 degrees to 0.05 degrees, 0.025 degrees, and 0.01 degrees resolutions and was compared with the direct downscaling (DD) at three scales. A comparative analysis was conducted in the Daxing'anling Mountains and Xiaoxing'anling Mountains. AGB and corresponding uncertainty maps at three scales were generated using SBSD. The results showed that the efficacy of the XGBoost-based AGB model combined with the random forest-based residual correction model was superior. Spatial patterns in AGB maps generated by SBSD and DD were found to be similar. Notably, SBSD yielded enhanced accuracy in the Daxing'anling Mountains with complex topography, while both performed comparably in the Xiaoxing'anling Mountains with milder topography, highlighting SBSD's advantages in high heterogeneity areas.
更多查看译文
关键词
ensemble machine learning,above-ground biomass,step-by-step downscaling
AI 理解论文
溯源树
样例
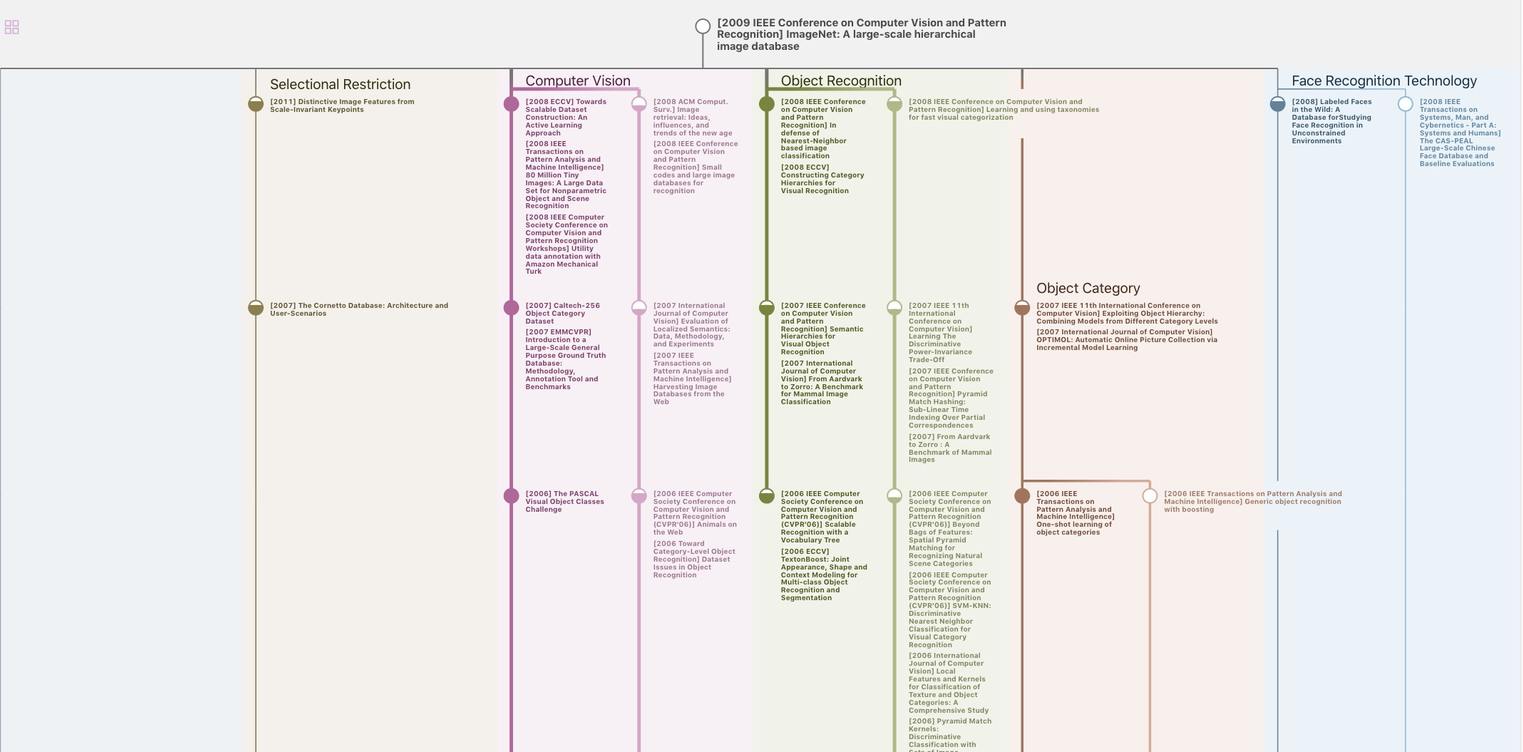
生成溯源树,研究论文发展脉络
Chat Paper
正在生成论文摘要