Stable Surface Regularization for Fast Few-Shot NeRF
CoRR(2024)
摘要
This paper proposes an algorithm for synthesizing novel views under few-shot
setup. The main concept is to develop a stable surface regularization technique
called Annealing Signed Distance Function (ASDF), which anneals the surface in
a coarse-to-fine manner to accelerate convergence speed. We observe that the
Eikonal loss - which is a widely known geometric regularization - requires
dense training signal to shape different level-sets of SDF, leading to
low-fidelity results under few-shot training. In contrast, the proposed surface
regularization successfully reconstructs scenes and produce high-fidelity
geometry with stable training. Our method is further accelerated by utilizing
grid representation and monocular geometric priors. Finally, the proposed
approach is up to 45 times faster than existing few-shot novel view synthesis
methods, and it produces comparable results in the ScanNet dataset and
NeRF-Real dataset.
更多查看译文
关键词
Implicit surface reconstruction,Sparse and noisy views
AI 理解论文
溯源树
样例
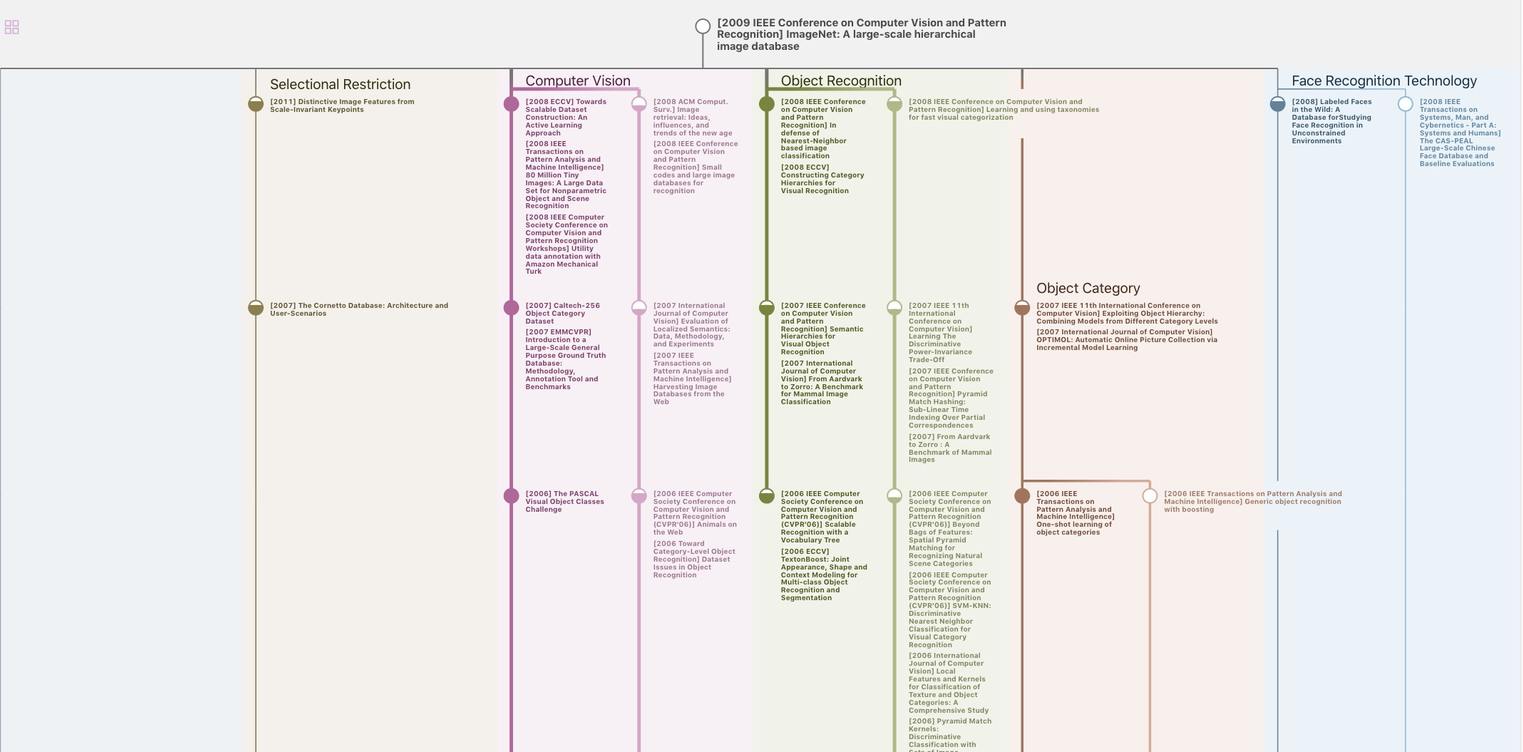
生成溯源树,研究论文发展脉络
Chat Paper
正在生成论文摘要