Long-short interest network with graph-based method for sequential recommendation
International Journal of Machine Learning and Cybernetics(2024)
摘要
In recommender systems, sequence information is crucial. Sequence data contains user preferences and reflects the evolution of user interests over time. Therefore, how to utilize sequence information to capture dynamic user interests is a critical issue in sequential recommender systems (SRSs). Attention-based methods are commonly used in SRSs and achieve state-of-the-art results. However, attention mechanisms lack the ability to represent the temporal dimension and cannot use sequence order effectively. To this end, this paper proposes a novel model structure called Long-Short Interest Network (LSIN), which fuses Long Short Term Memory (LSTM) and Transformer encoder. We use two LSTM layers to capture the user’s long-term and short-term interests, respectively. Furthermore, adding the LSTM can help the self-attention mechanism better model the sequential relationship between items. In addition, most embedding models generate embedding vectors based on individual items without considering the connection between items and users. This will be an obstacle and bring difficulty for later models to capture the evolution of user interests. Therefore, we use a heterogeneous graph to model the interactions between users and items. And design a weight-based graph embedding for generating embedding vectors, which can encode higher-order structural information by propagating on the graph. Finally, we propose LSRec, a framework that unites the above two structures to achieve more accurate recommendations. The new model yielded significant benefits. The experiments on four benchmark data sets demonstrate the effectiveness of LSRec, which achieves almost 5 % improvement in NDCG@10 compared with Self-Attention based Sequential Recommendation model (SASRec).
更多查看译文
关键词
Recommender system,LSTM,Self-attention,Neural networks,Graph embedding
AI 理解论文
溯源树
样例
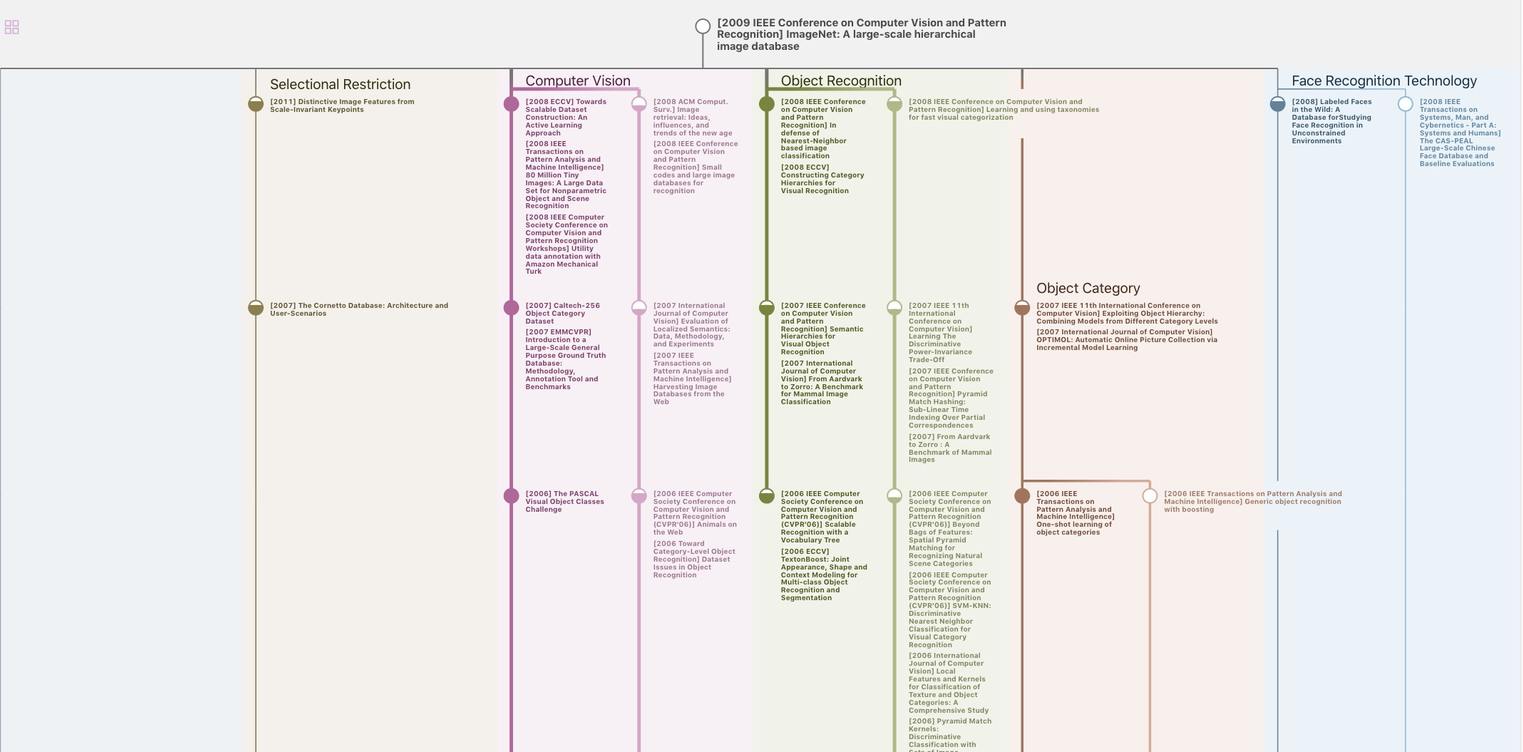
生成溯源树,研究论文发展脉络
Chat Paper
正在生成论文摘要