Contrastive Domain Generalization Convolution Neural Network Correcting the Drift of Gas Sensors
SENSORS AND ACTUATORS A-PHYSICAL(2024)
关键词
Drift problems,Gas sensor,Time series classification,Domain generalization,Contrastive learning
AI 理解论文
溯源树
样例
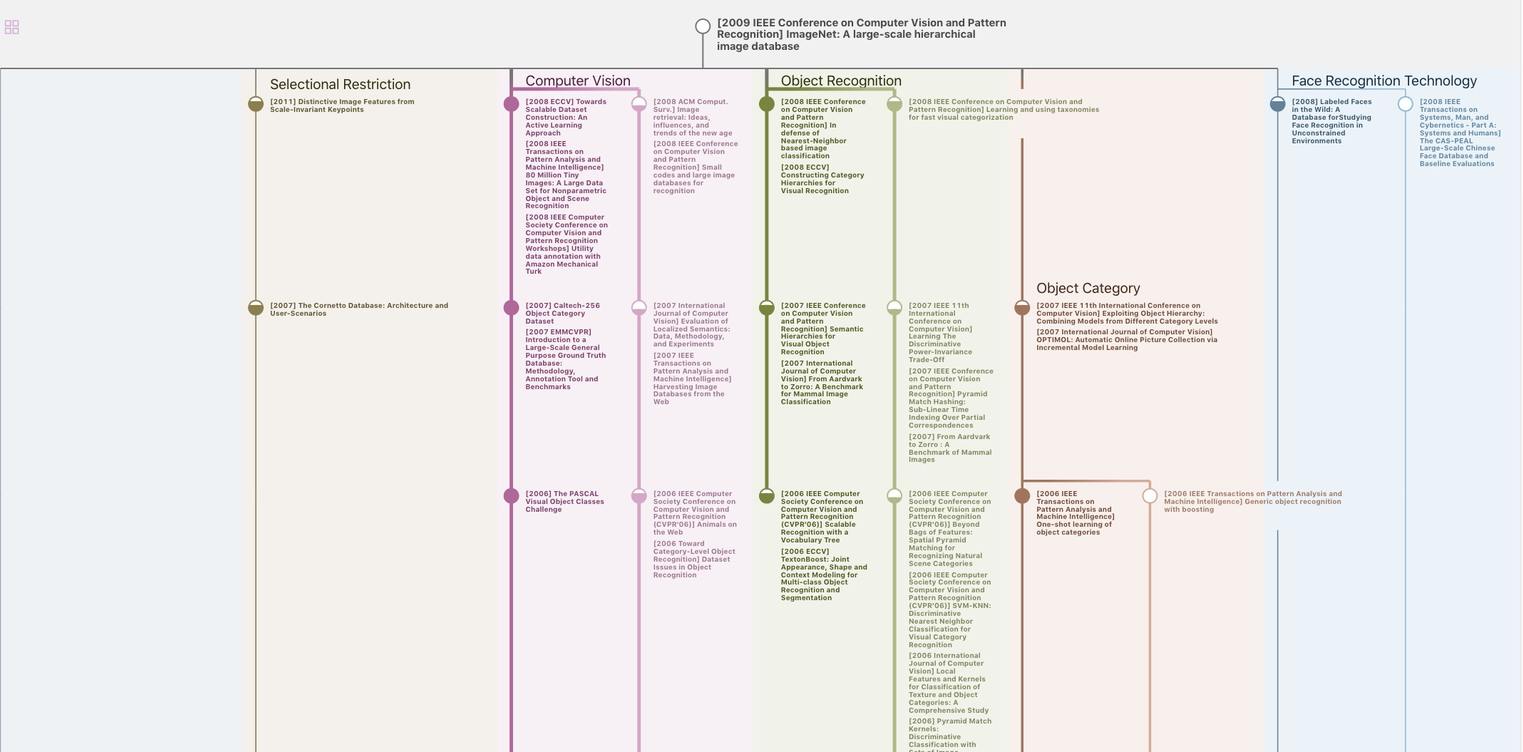
生成溯源树,研究论文发展脉络
Chat Paper
正在生成论文摘要