Generating Diverse Agricultural Data for Vision-Based Farming Applications
CoRR(2024)
摘要
We present a specialized procedural model for generating synthetic
agricultural scenes, focusing on soybean crops, along with various weeds. This
model is capable of simulating distinct growth stages of these plants, diverse
soil conditions, and randomized field arrangements under varying lighting
conditions. The integration of real-world textures and environmental factors
into the procedural generation process enhances the photorealism and
applicability of the synthetic data. Our dataset includes 12,000 images with
semantic labels, offering a comprehensive resource for computer vision tasks in
precision agriculture, such as semantic segmentation for autonomous weed
control. We validate our model's effectiveness by comparing the synthetic data
against real agricultural images, demonstrating its potential to significantly
augment training data for machine learning models in agriculture. This approach
not only provides a cost-effective solution for generating high-quality,
diverse data but also addresses specific needs in agricultural vision tasks
that are not fully covered by general-purpose models.
更多查看译文
AI 理解论文
溯源树
样例
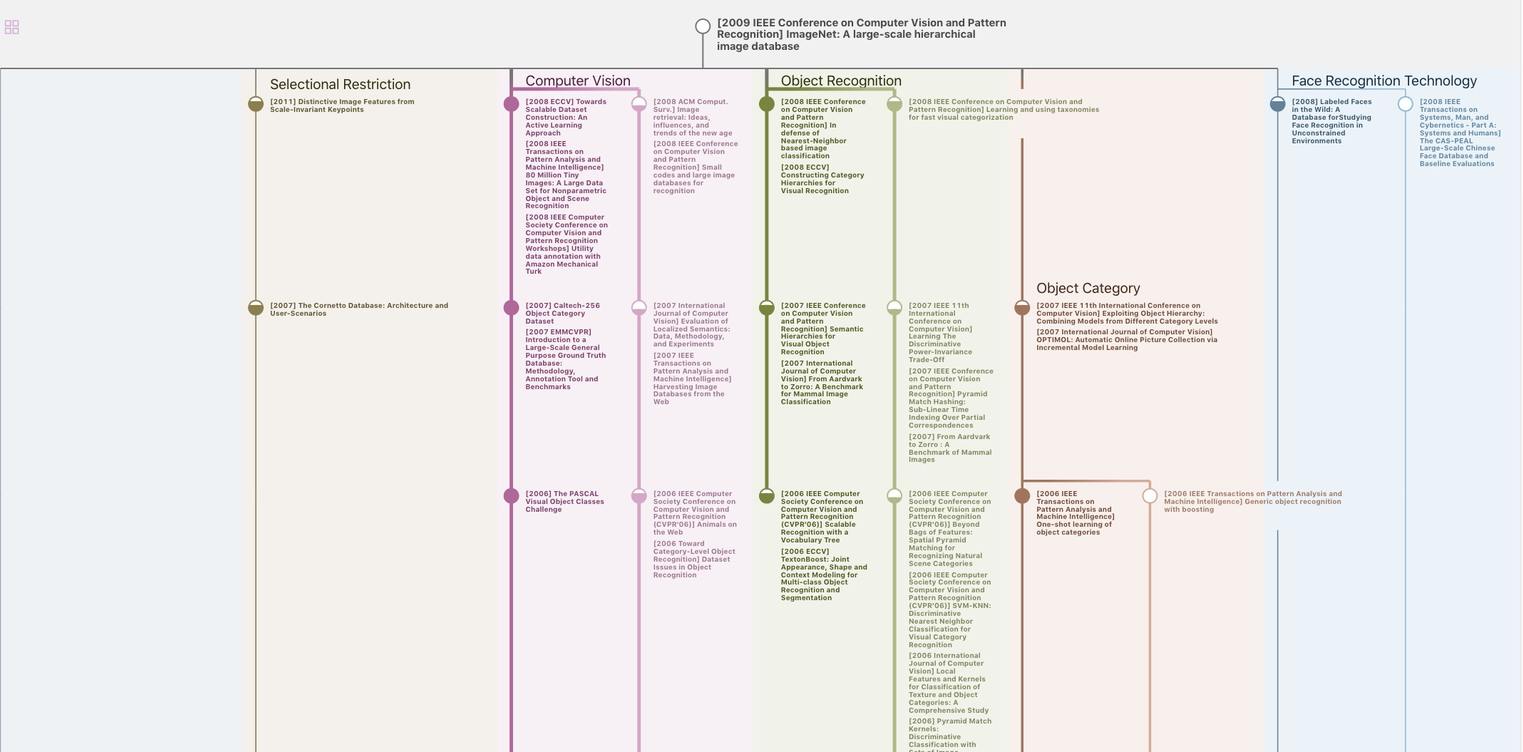
生成溯源树,研究论文发展脉络
Chat Paper
正在生成论文摘要