Digital audio tampering detection based on spatio-temporal representation learning of electrical network frequency
Multimedia Tools and Applications(2024)
摘要
The majority of Digital Audio Tampering Detection (DATD) methods, which are based on Electrical Network Frequency (ENF), predominantly concentrate on the static spatial information of ENF. Unfortunately, this focus neglects the temporal variation present in the ENF time series. This limitation significantly hampers the ENF feature representation capability, consequently diminishing the overall accuracy of tampering detection. To address this gap, our paper introduces an innovative digital audio tampering detection method founded on ENF spatio-temporal feature representation learning. To enhance the feature representation capability and subsequently improve tampering detection accuracy, we propose the construction of a parallel spatio-temporal network model. This model incorporates both Convolutional Neural Network (CNN) and Bidirectional Long Short-Term Memory (BiLSTM) network architectures. Through this hybrid model, we aim to deeply extract both ENF spatial and temporal feature information. In the process of extracting spatial and temporal features of ENF, we utilize high-precision Discrete Fourier Transform (DFT) analysis on digital audio. This analysis allows us to extract ENF phase sequences, which are then adaptively divided into frames through frame shifting. The result is feature matrices of uniform size, effectively representing the spatial features of ENF. Concurrently, phase sequences are segmented into frames based on ENF time changes to capture the temporal features of ENF. Subsequently, deep spatial and temporal features are extracted using CNN and BiLSTM, respectively. To further enhance the representation capability of the spatio-temporal features, we introduce an attention mechanism. This mechanism dynamically assigns weights to the deep spatial and temporal features, providing a nuanced and refined representation. Finally, a deep neural network is employed to discern whether the audio has undergone tampering. Our experimental results validate the effectiveness of our approach, showcasing superior performance compared to six state-of-the-art methods across three public databases for digital audio tampering detection. This comprehensive methodology, focusing on both spatial and temporal aspects of ENF, establishes a robust foundation for advancing the field of DATD and contributes significantly to improving detection accuracy.
更多查看译文
关键词
Audio forensics,Spatio-temporal features,ENF,Convolution neural network,Bidirectional long short-term memory network
AI 理解论文
溯源树
样例
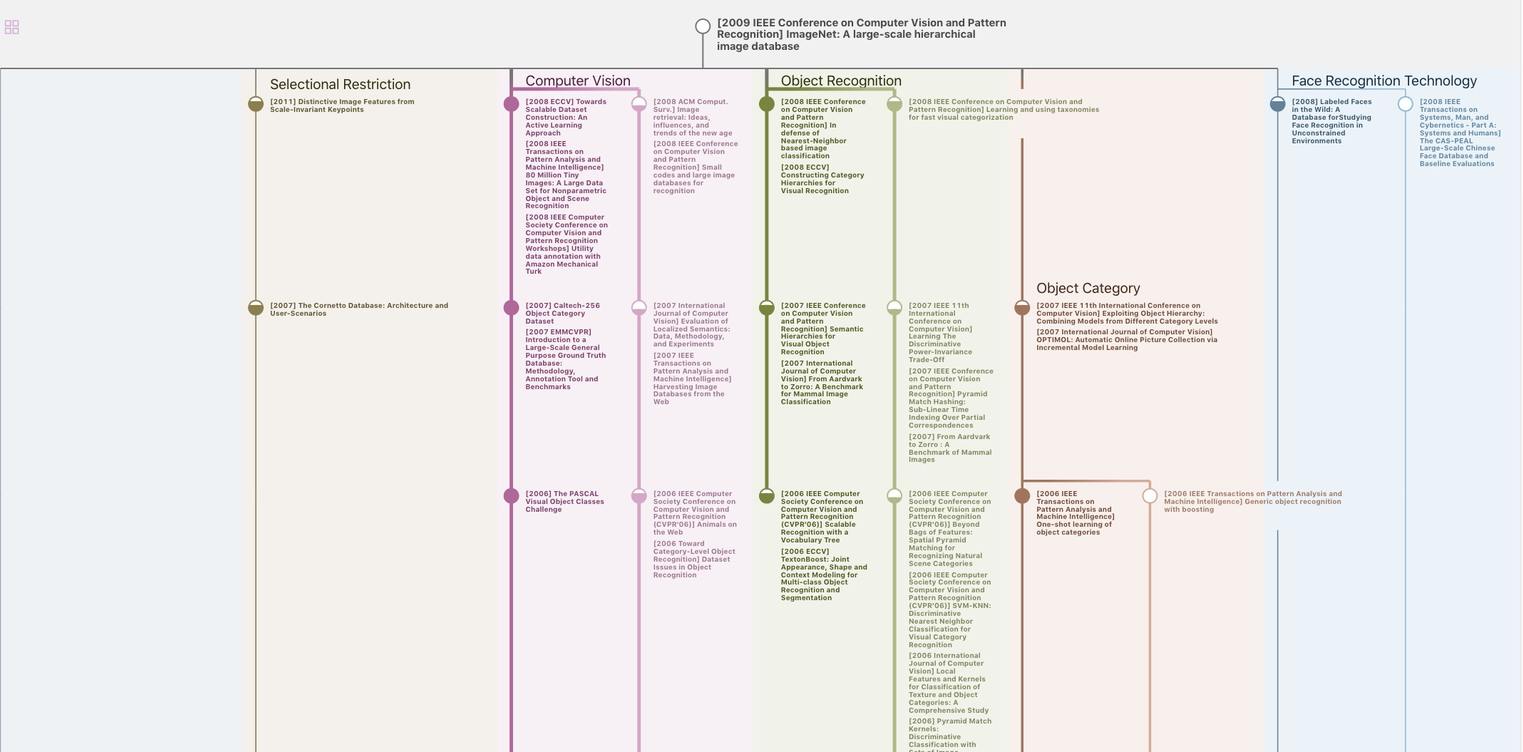
生成溯源树,研究论文发展脉络
Chat Paper
正在生成论文摘要