Asymptotic optimality of the square-root transformation on the gamma distribution using the Kullback–Leibler information number criterion
Statistics & Probability Letters(2024)
摘要
The Kullback–Leibler information number criterion is a useful criterion for determining an optimal power transformation to approximate the target distribution. When the normal distribution with a fixed variance is set as the target distribution, this criterion may be used to show the asymptotic optimality of the square-root transformation. In particular, when the Box–Cox transformation corresponding to the square-root transformation is applied, the Kullback–Leibler information number of the transformed gamma distribution with the scale parameter equal to the fixed variance converges to zero asymptotically as the shape parameter approaches infinity. That provides a stronger result on the mode of convergence of the variance-stabilizing power transformation on the gamma distribution. Moreover, an analogous result may be obtained by setting the Laplace distribution with a fixed scale parameter as the target distribution. This result utilizes a novel application of the asymptotic expansion for the normalized upper incomplete gamma function at the transition point.
更多查看译文
关键词
Box–Cox transformation,Incomplete gamma function,Kullback–Leibler divergence,Laplace distribution,Normality,Variance-stabilizing transformation
AI 理解论文
溯源树
样例
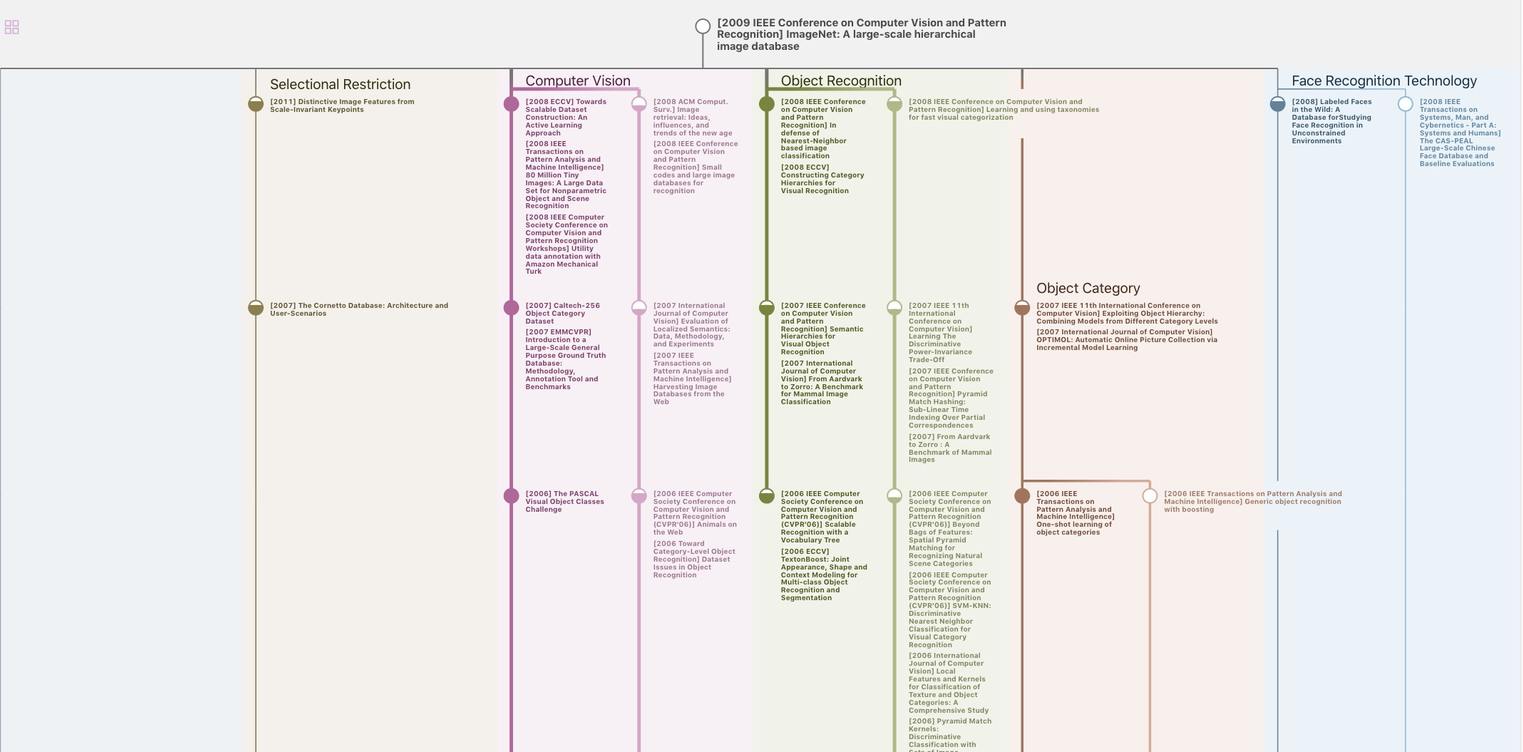
生成溯源树,研究论文发展脉络
Chat Paper
正在生成论文摘要