An expert system for hybrid edge to cloud computational offloading in heterogeneous MEC-MCC environments
Journal of Network and Computer Applications(2024)
摘要
Mobile Cloud Computing (MCC) integrates cloud computing into the mobile environment, effectively tackling performance, environmental, and security constraints. However, the proliferation of clouds and applications introduces complexities in offloading decisions. Mobile Edge Computing (MEC) has emerged as a solution to bolster MCC performance, capitalizing on the proximity to edge devices. However, optimizing computation offloading remains paramount for heterogeneous smart (mobile phones, wearables, and IoT) devices, necessitating efficient utilization of network resources and computational offloading. To address these challenges, this paper introduces NSANNOM (Network and Device Resources Utilization through Smart ANN-based Offloading Mechanism), an expert system designed for optimal computational offloading decision-making and efficient network resource allocation mechanisms. NSANNOM employs Artificial Neural Networks (ANN) for precise decision-making by validating real-world datasets, underscoring ANN’s superiority over existing algorithms, and showcasing enhanced energy savings, cost efficiency, and latency response. Experimental evaluations demonstrate that the proposed ANN model for the offloading decision-making algorithm achieves a training accuracy of 97% and a validation accuracy of 99%. The system consumes minimal energy (10 MJ) for task scheduling and exhibits remarkable accuracy in resource utilization across multiple tasks (10-50) ranging in size (from 1 to 16 GB). Additionally, it minimizes time delays (in milliseconds) during the offloading process.
更多查看译文
关键词
MEC,MCC,Computation offloading,ANN algorithm,Machine learning,Optimization
AI 理解论文
溯源树
样例
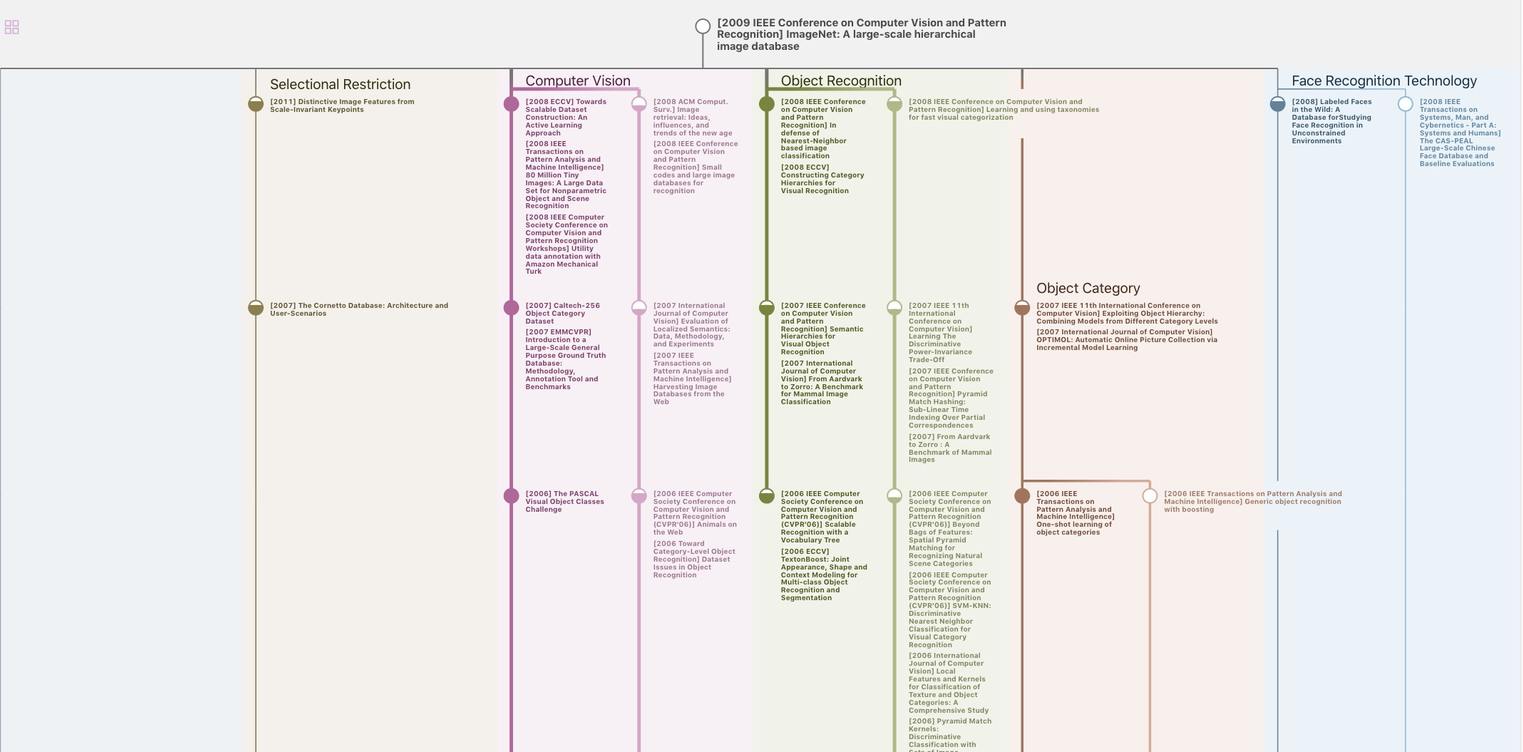
生成溯源树,研究论文发展脉络
Chat Paper
正在生成论文摘要