Unsupervised Classification of Multi-Contrast Magnetic Resonance Histology of Peripheral Arterial Disease Lesions Using a Convolutional Variational Autoencoder with a Gaussian Mixture Model in Latent Space: a Technical Feasibility Study
Computerized Medical Imaging and Graphics(2024)
摘要
Purpose
To investigate the feasibility of a deep learning algorithm combining variational autoencoder (VAE) and two-dimensional (2D) convolutional neural networks (CNN) for automatically quantifying hard tissue presence and morphology in multi-contrast magnetic resonance (MR) images of peripheral arterial disease (PAD) occlusive lesions.
Methods
Multi-contrast MR images (T2-weighted and ultrashort echo time) were acquired from lesions harvested from six amputated legs with high isotropic spatial resolution (0.078mm and 0.156mm, respectively) at 9.4T. A total of 4014 pseudo-color combined images were generated, with 75% used to train a VAE employing custom 2D CNN layers. A Gaussian mixture model (GMM) was employed to classify the latent space data into four tissue classes: I) concentric calcified (c), II) eccentric calcified (e), III) occluded with hard tissue (h) and IV) occluded with soft tissue (s). Test image probabilities, encoded by the trained VAE were used to evaluate model performance.
Results
GMM component classification probabilities ranged from 0.92-0.97 for class (c), 1.00 for class (e), 0.82-0.95 for class (h) and 0.56-0.93 for the remaining class (s). Due to the complexity of soft-tissue lesions reflected in the heterogeneity of the pseudo-color images, more GMM components (n=17) were attributed to class (s), compared to the other three (c, e and h) (n=6).
Conclusion
Combination of 2D CNN VAE and GMM achieves high classification probabilities for hard tissue-containing lesions. Automatic recognition of these classes may aid therapeutic decision-making and identifying uncrossable lesions prior to endovascular intervention.
更多查看译文
关键词
magnetic resonance imaging,artificial intelligence,variational autoencoder,computational neural networks,Gaussian mixture model,peripheral arterial disease
AI 理解论文
溯源树
样例
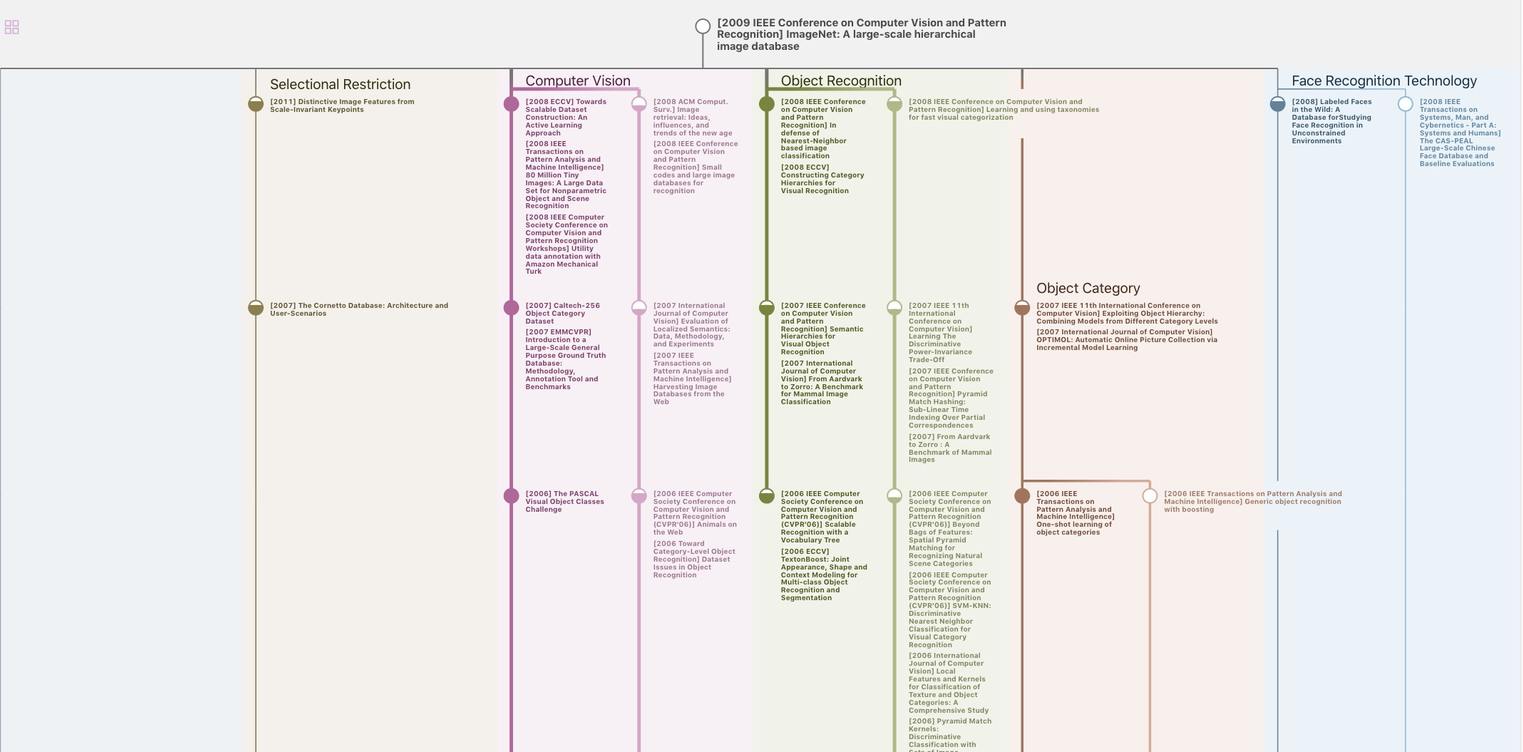
生成溯源树,研究论文发展脉络
Chat Paper
正在生成论文摘要