SAUI: Scale-Aware Unseen Imagineer for Zero-Shot Object Detection
AAAI 2024(2024)
摘要
Zero-shot object detection (ZSD) aims to localize and classify unseen objects without access to their training annotations. As a prevailing solution to ZSD, generation-based methods synthesize unseen visual features by taking seen features as reference and class semantic embeddings as guideline. Although previous works continuously improve the synthesis quality, they fail to consider the scale-varying nature of unseen objects. The generation process is preformed over a single scale of object features and thus lacks scale-diversity among synthesized features. In this paper, we reveal the scale-varying challenge in ZSD and propose a Scale-Aware Unseen Imagineer (SAUI) to lead the way of a novel scale-aware ZSD paradigm. To obtain multi-scale features of seen-class objects, we design a specialized coarse-to-fine extractor to capture features through multiple scale-views. To generate unseen features scale by scale, we innovate a Series-GAN synthesizer along with three scale-aware contrastive components to imagine separable, diverse and robust scale-wise unseen features. Extensive experiments on PASCAL VOC, COCO and DIOR datasets demonstrate SAUI's better performance in different scenarios, especially for scale-varying and small objects. Notably, SAUI achieves the new state-of-the art performance on COCO and DIOR.
更多查看译文
关键词
CV: Object Detection & Categorization
AI 理解论文
溯源树
样例
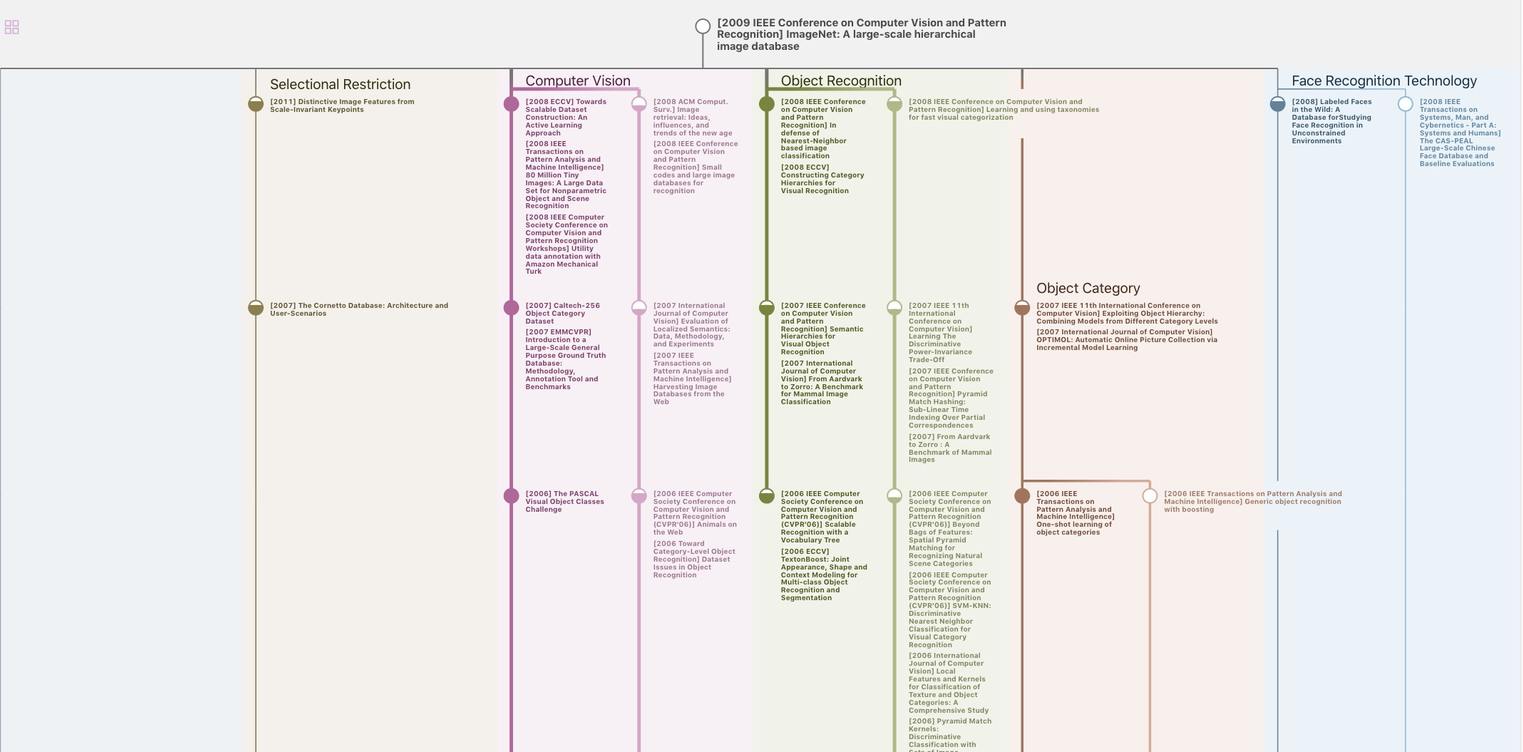
生成溯源树,研究论文发展脉络
Chat Paper
正在生成论文摘要