Integrating Machine Learning and Social Sensing in Smart City Digital Twin for Citizen Feedback.
IEEE International Conference on Smart City(2023)
摘要
Smart City Digital Twin (SCDT), a virtual representation of a physical city, is an emerging technology for optimizing urban services and enhancing urban planning and decision-making. The integration of Machine Learning (ML) and social sensing provides valuable insights into public feedback to policymakers and for informed decision-making and responsive urban governance. This study aims to explore the use of social media data and topic modeling algorithms in the context of SCDT to highlight the concerns of citizens in Saskatoon, Canada. In this work, we use the Uniform Manifold Approximation and Projection (UMAP) and K-means clustering algorithm in the BERTopic architecture to extract 30 topics from comments collected through the Saskatoon subreddit posts. The topics were then merged into 15 themes to discover the concerns. A pretrained transformer model, SiEBERT was used to determine the sentiments of the Reddit comments. The research findings highlighted concerns such as - unreliable public transit, high cost of living, long wait times in emergency rooms, shortage of family doctors, drug addiction, and lack of mental awareness.
更多查看译文
关键词
Machine Learning,Social Media Sensing,BERTopic,Sentiment Analysis,Smart City Digital Twin,Smart Governance,Reddit
AI 理解论文
溯源树
样例
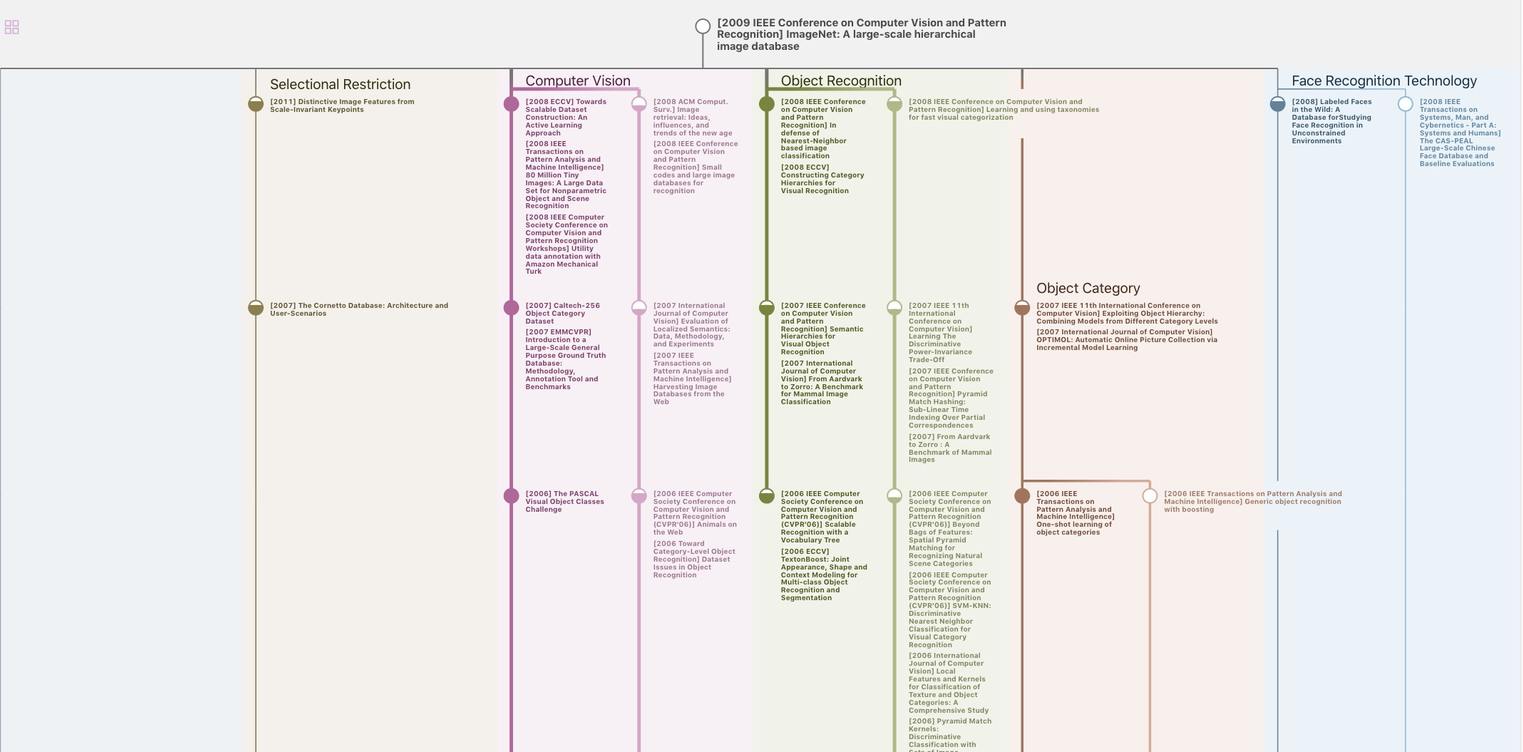
生成溯源树,研究论文发展脉络
Chat Paper
正在生成论文摘要