Two-Stage Trajectory Generation Model for Realistic Human Mobility Simulation.
IEEE International Conference on Smart City(2023)
摘要
Human mobility data is crucial for understanding mobility patterns and provides valuable insights for fields like urban planning and tourism recommendation. To address limitations in trajectory generation methods that treat all points equally, this study proposes a two-stage trajectory simulation model that generates separate sequences for stopping points and moving points. By differentiating between these two types of points, the model can effectively capture mobility intentions and moving paths. The stopping point sequence generator utilizes a GAN-based approach to capture mobility intentions and patterns, while the moving point generator constructs a weighted directed road network graph to determine efficient paths between stopping points. Extensive experiments using a real-life mobility dataset demonstrate the effectiveness of the proposed model in generating realistic trajectories. Moreover, when used for next location prediction task, the synthetic trajectory data generated by our model produces results that closely resemble real-world situations.
更多查看译文
关键词
Trajectory Simulation,GAN,Data Augmentation
AI 理解论文
溯源树
样例
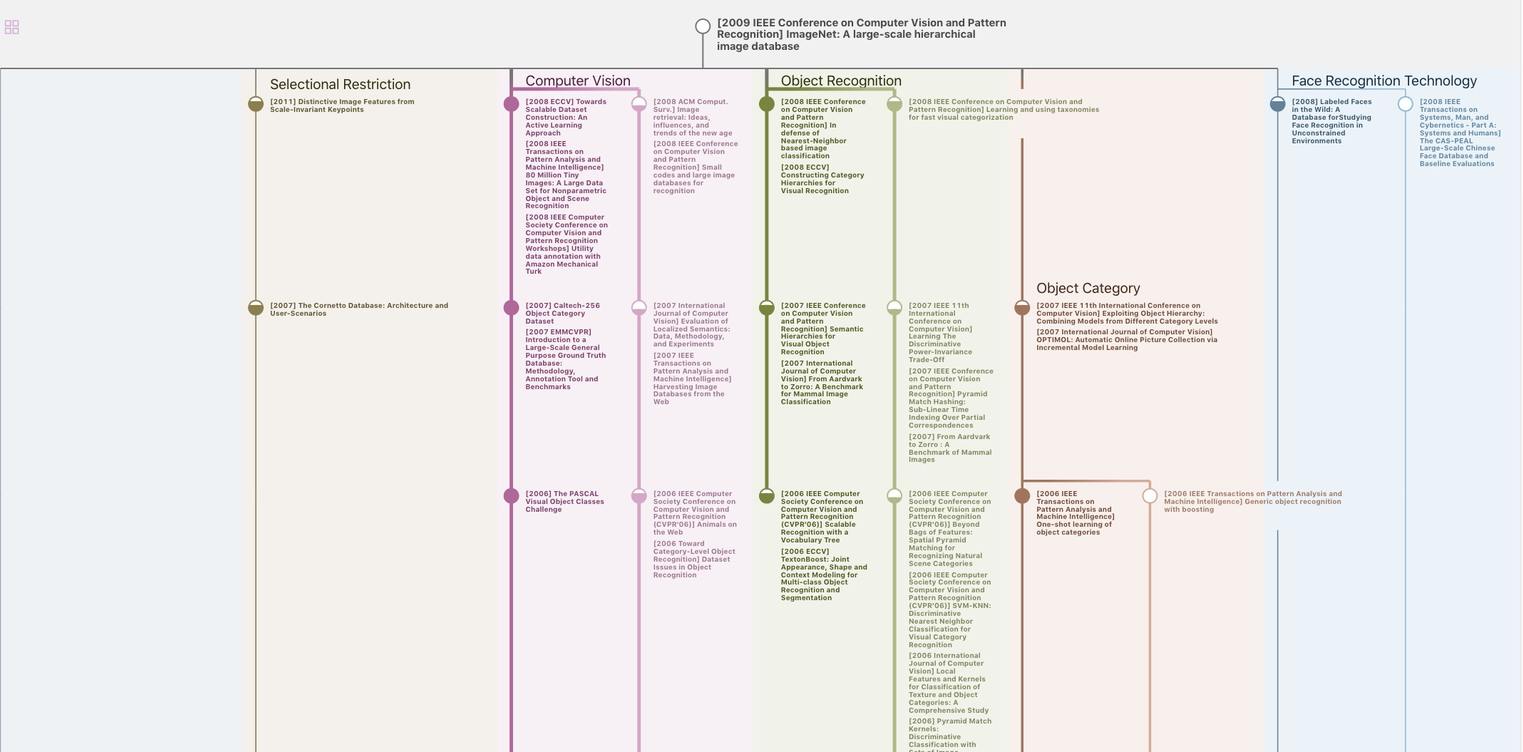
生成溯源树,研究论文发展脉络
Chat Paper
正在生成论文摘要