A Pre-convolved Representation for Plug-and-Play Neural Illumination Fields
AAAI 2024(2024)
摘要
Recent advances in implicit neural representation have demonstrated the ability to recover detailed geometry and material from multi-view images. However, the use of simplified lighting models such as environment maps to represent non-distant illumination, or using a network to fit indirect light modeling without a solid basis, can lead to an undesirable decomposition between lighting and material. To address this, we propose a fully differentiable framework named Neural Illumination Fields (NeIF) that uses radiance fields as a lighting model to handle complex lighting in a physically based way. Together with integral lobe encoding for roughness-adaptive specular lobe and leveraging the pre-convolved background for accurate decomposition, the proposed method represents a significant step towards integrating physically based rendering into the NeRF representation. The experiments demonstrate the superior performance of novel-view rendering compared to previous works, and the capability to re-render objects under arbitrary NeRF-style environments opens up exciting possibilities for bridging the gap between virtual and real-world scenes.
更多查看译文
关键词
CV: 3D Computer Vision,CV: Computational Photography, Image & Video Synthesis,CV: Low Level & Physics-based Vision
AI 理解论文
溯源树
样例
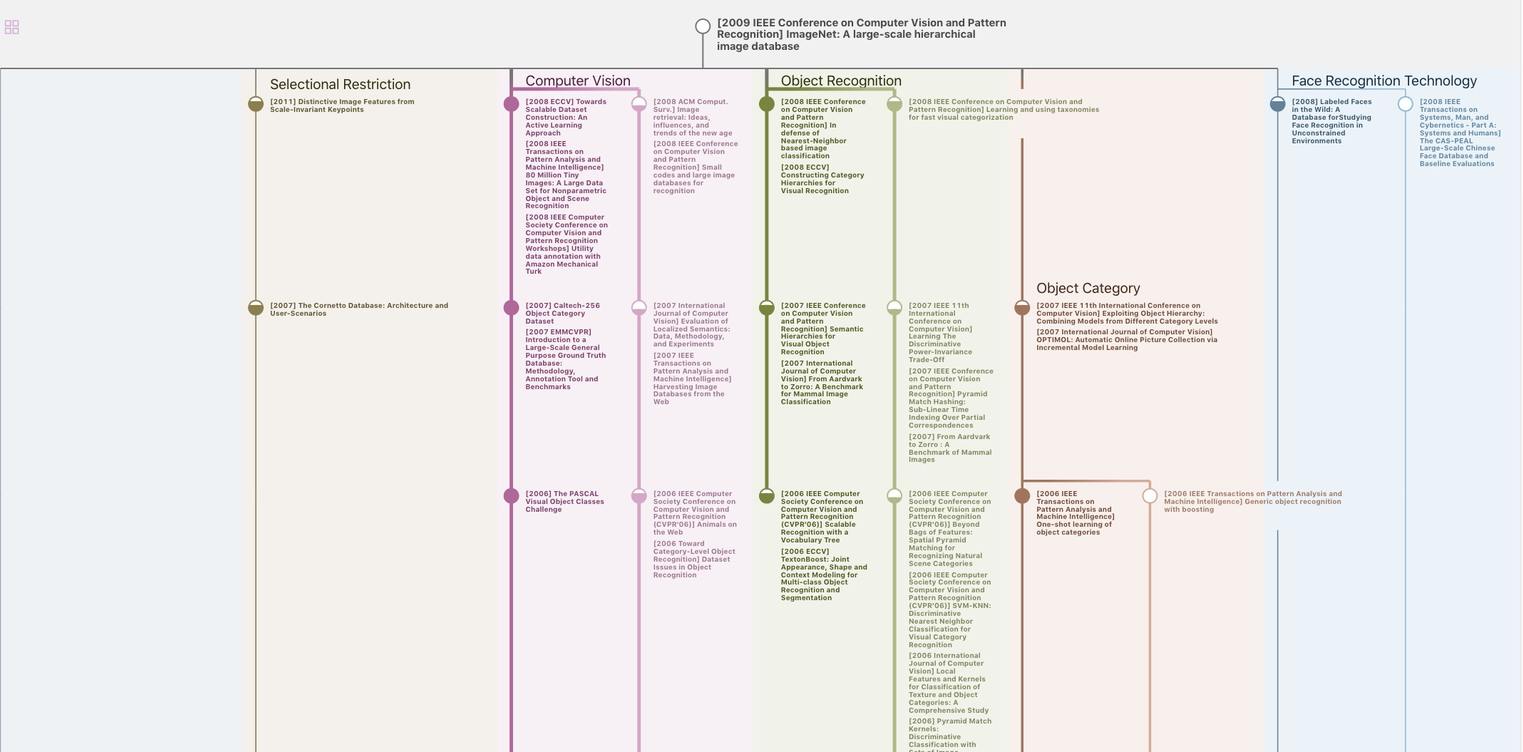
生成溯源树,研究论文发展脉络
Chat Paper
正在生成论文摘要