Active Learning Guided by Efficient Surrogate Learners
AAAI 2024(2024)
摘要
Re-training a deep learning model each time a single data point receives a new label is impractical due to the inherent complexity of the training process. Consequently, existing active learning (AL) algorithms tend to adopt a batch-based approach where, during each AL iteration, a set of data points is collectively chosen for annotation. However, this strategy frequently leads to redundant sampling, ultimately eroding the efficacy of the labeling procedure. In this paper, we introduce a new AL algorithm that harnesses the power of a Gaussian process surrogate in conjunction with the neural network principal learner. Our proposed model adeptly updates the surrogate learner for every new data instance, enabling it to emulate and capitalize on the continuous learning dynamics of the neural network without necessitating a complete retraining of the principal model for each individual label. Experiments on four benchmark datasets demonstrate that this approach yields significant enhancements, either rivaling or aligning with the performance of state-of-the-art techniques.
更多查看译文
关键词
ML: Active Learning,ML: Classification and Regression
AI 理解论文
溯源树
样例
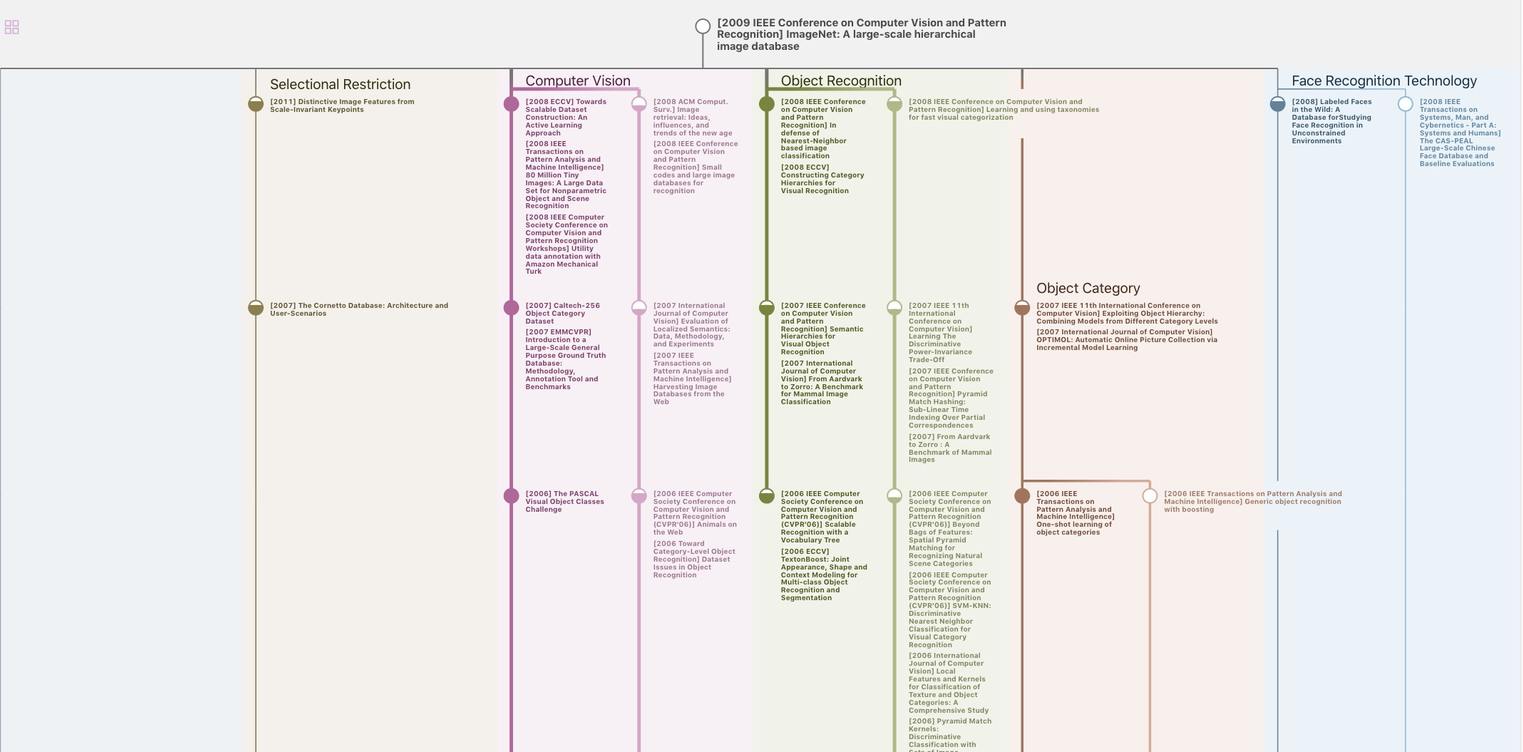
生成溯源树,研究论文发展脉络
Chat Paper
正在生成论文摘要