Rolling-Unet: Revitalizing MLP’s Ability to Efficiently Extract Long-Distance Dependencies for Medical Image Segmentation
AAAI 2024(2024)
摘要
Medical image segmentation methods based on deep learning network are mainly divided into CNN and Transformer. However, CNN struggles to capture long-distance dependencies, while Transformer suffers from high computational complexity and poor local feature learning. To efficiently extract and fuse local features and long-range dependencies, this paper proposes Rolling-Unet, which is a CNN model combined with MLP. Specifically, we propose the core R-MLP module, which is responsible for learning the long-distance dependency in a single direction of the whole image. By controlling and combining R-MLP modules in different directions, OR-MLP and DOR-MLP modules are formed to capture long-distance dependencies in multiple directions. Further, Lo2 block is proposed to encode both local context information and long-distance dependencies without excessive computational burden. Lo2 block has the same parameter size and computational complexity as a 3×3 convolution. The experimental results on four public datasets show that Rolling-Unet achieves superior performance compared to the state-of-the-art methods.
更多查看译文
关键词
CV: Representation Learning for Vision,CV: Medical and Biological Imaging,CV: Segmentation
AI 理解论文
溯源树
样例
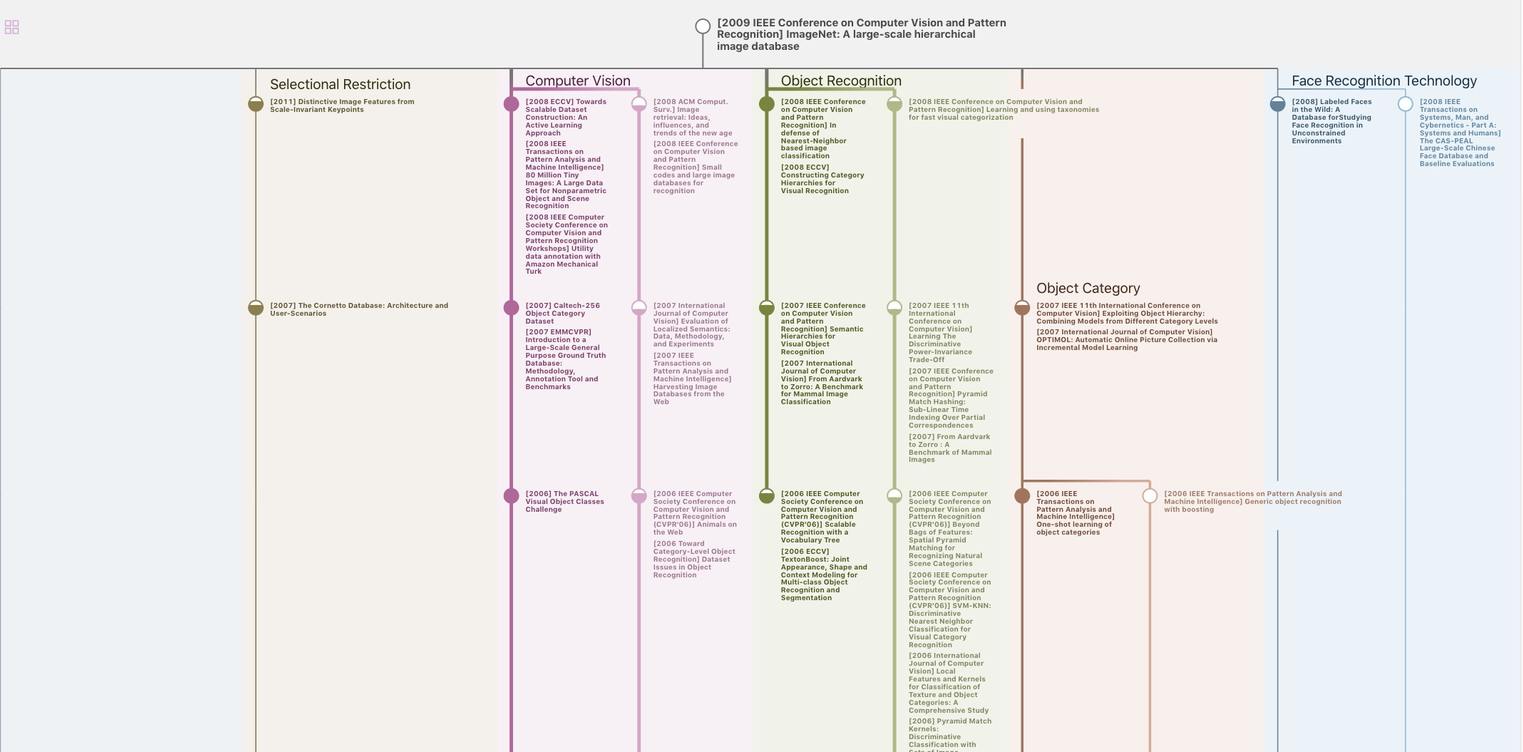
生成溯源树,研究论文发展脉络
Chat Paper
正在生成论文摘要