A Transfer Approach Using Graph Neural Networks in Deep Reinforcement Learning
AAAI 2024(2024)
摘要
Transfer learning (TL) has shown great potential to improve Reinforcement Learning (RL) efficiency by leveraging prior knowledge in new tasks. However, much of the existing TL research focuses on transferring knowledge between tasks that share the same state-action spaces. Further, transfer from multiple source tasks that have different state-action spaces is more challenging and needs to be solved urgently to improve the generalization and practicality of the method in real-world scenarios. This paper proposes TURRET (Transfer Using gRaph neuRal nETworks), to utilize the generalization capabilities of Graph Neural Networks (GNNs) to facilitate efficient and effective multi-source policy transfer learning in the state-action mismatch setting. TURRET learns a semantic representation by accounting for the intrinsic property of the agent through GNNs, which leads to a unified state embedding space for all tasks. As a result, TURRET achieves more efficient transfer with strong generalization ability between different tasks and can be easily combined with existing Deep RL algorithms. Experimental results show that TURRET significantly outperforms other TL methods on multiple continuous action control tasks, successfully transferring across robots with different state-action spaces.
更多查看译文
关键词
ML: Reinforcement Learning,ROB: Learning & Optimization for ROB,ML: Transfer, Domain Adaptation, Multi-Task Learning,ML: Deep Neural Architectures and Foundation Models
AI 理解论文
溯源树
样例
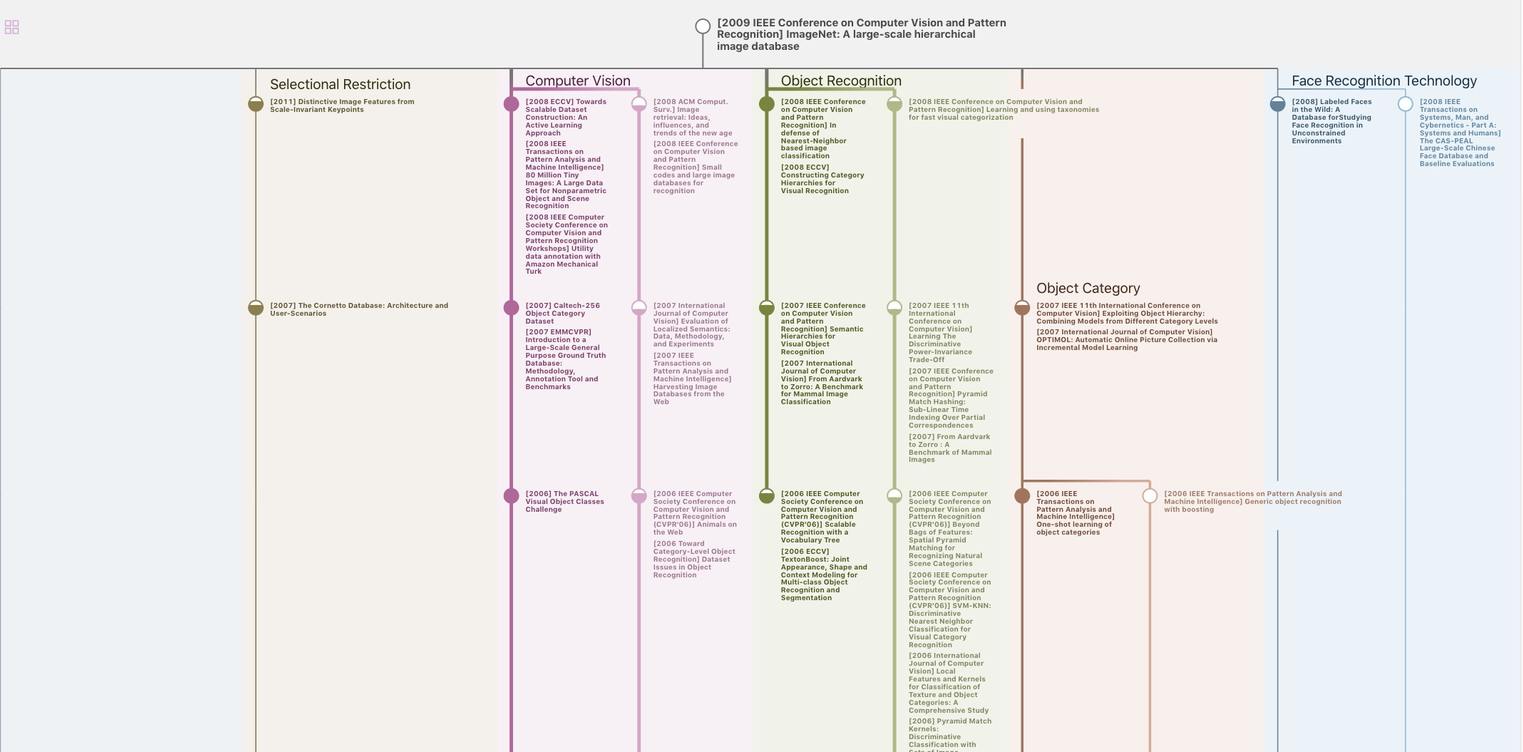
生成溯源树,研究论文发展脉络
Chat Paper
正在生成论文摘要