Synthetic Meets Authentic: Leveraging Text-to-Image Generated Datasets for Apple Detection in Orchard Environments
SSRN Electronic Journal(2024)
摘要
Abstract Training machine learning (ML) models for computer vision-based object detection process typically requires large, labeled datasets, a process often burdened by significant human effort and high costs associated with imaging systems and image acquisition. This research aimed to simplify image data collection for object detection in orchards by avoiding traditional fieldwork with different imaging sensors. Utilizing OpenAI's DALLE, a large language model (LLM) for realistic image generation, we generated and annotated a cost effective dataset. This dataset, exclusively generated with text-to-image prompts/inputs, was then utilized to train a deep learning model, YOLOv8, for apple detection, which was then tested with real-world (outdoor orchard) images captured by a digital (Nikon D5100) camera as well as a machine vision camera (IntelRealsense D435i). The model achieved a training precision of 0.83, recall of 0.99, an F1 score of 0.92, and mAP@50 at 0.96. Validation tests against actual images collected over two different varieties of apples (Honeycrisp and Envy) in a commercial orchard environment showed a precision of 0.82 and 0.75, recall of 0.88 and 0.63, and mAP@50 of 0.92 and 0.70, each respectively. The inference time of the model was 0.015 seconds for the digital camera-based images and 0.012 seconds for the machine vision camera based images. This study presents a pathway for generating large image datasets in challenging agricultural fields with minimal or no labor-intensive efforts in field data-collection, which could accelerate the development and deployment of computer vision and robotic technologies in orchard environments.
更多查看译文
AI 理解论文
溯源树
样例
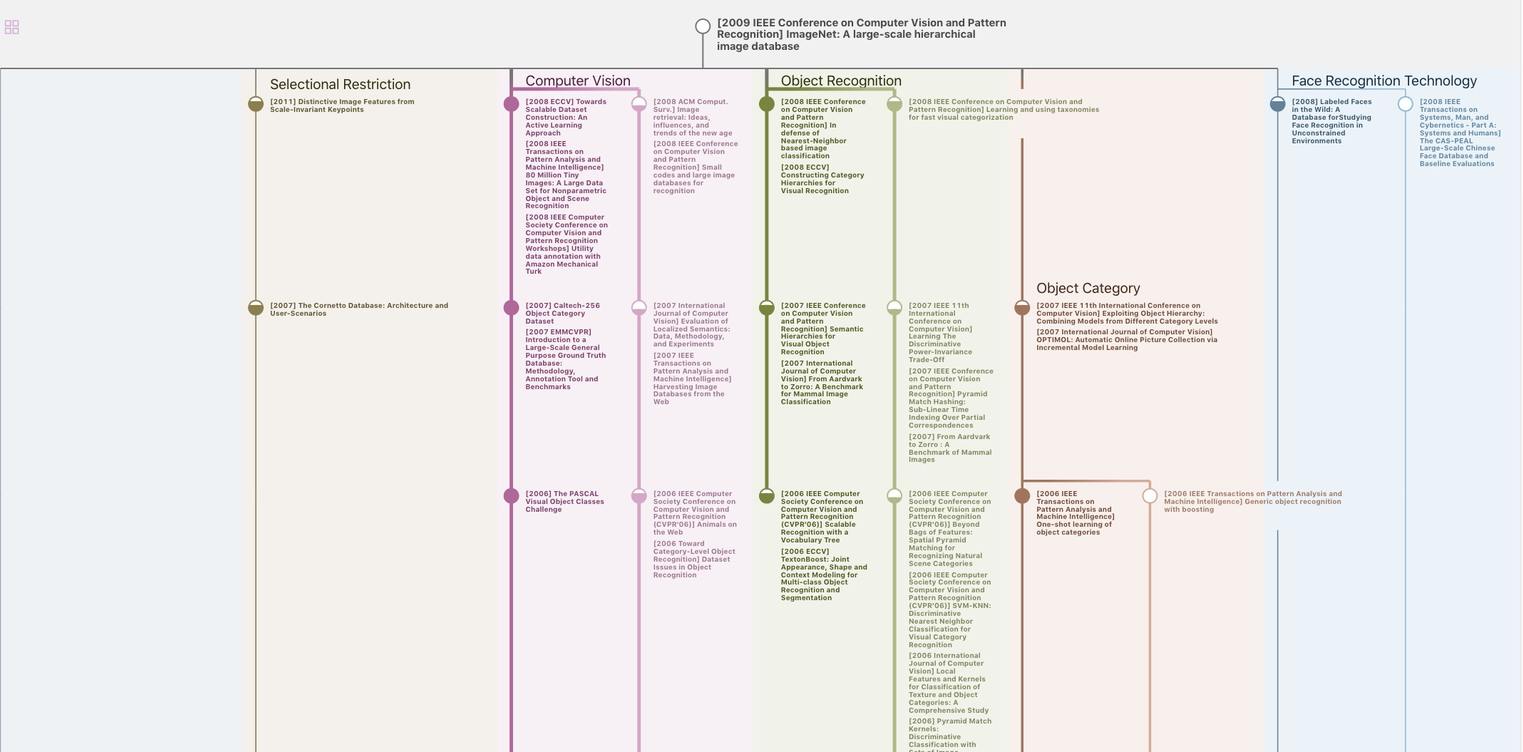
生成溯源树,研究论文发展脉络
Chat Paper
正在生成论文摘要