Tiny Object Detection in Remote Sensing Images Based on Object Reconstruction and Multiple Receptive Field Adaptive Feature Enhancement
IEEE Transactions on Geoscience and Remote Sensing(2024)
摘要
Tiny object detection in the field of remote sensing has always been a challenging and interesting topic. Despite many researchers have been working on this problem, it has not been well solved due to its complexity. In this paper, we analyze the reasons for the poor performance of deep learning-based object detection methods for tiny objects in remote sensing images. Moreover, we propose a new remote sensing image tiny object detection network based on object reconstruction and multiple receptive field adaptive feature enhancement module (MRFAFEM), called ORFENet. Detailedly, object reconstruction aims to reduce the information loss of tiny objects within deep neural networks, which is only used in the training phase and can be discarded in the inference phase. MRFAFEM is designed to enhance the features for detecting tiny objects by dynamically adjusting the multiple receptive field features. We have conducted several experiments on the AI-TODv2 and LEVIR-Ship datasets, both of which are proposed for tiny object detection in remote sensing images. The experimental results indicate the effectiveness of the proposed method. Specifically, the proposed ORFENet can achieve the AP of 24.8% on the AI-TODv2 dataset and 83.3% AP50 on the LEVIR-Ship dataset. The code will be released at https://github.com/dyl96/ORFENet.
更多查看译文
关键词
Tiny object detection,remote sensing images,object reconstruction,multiple receptive field adaptive feature enhancement
AI 理解论文
溯源树
样例
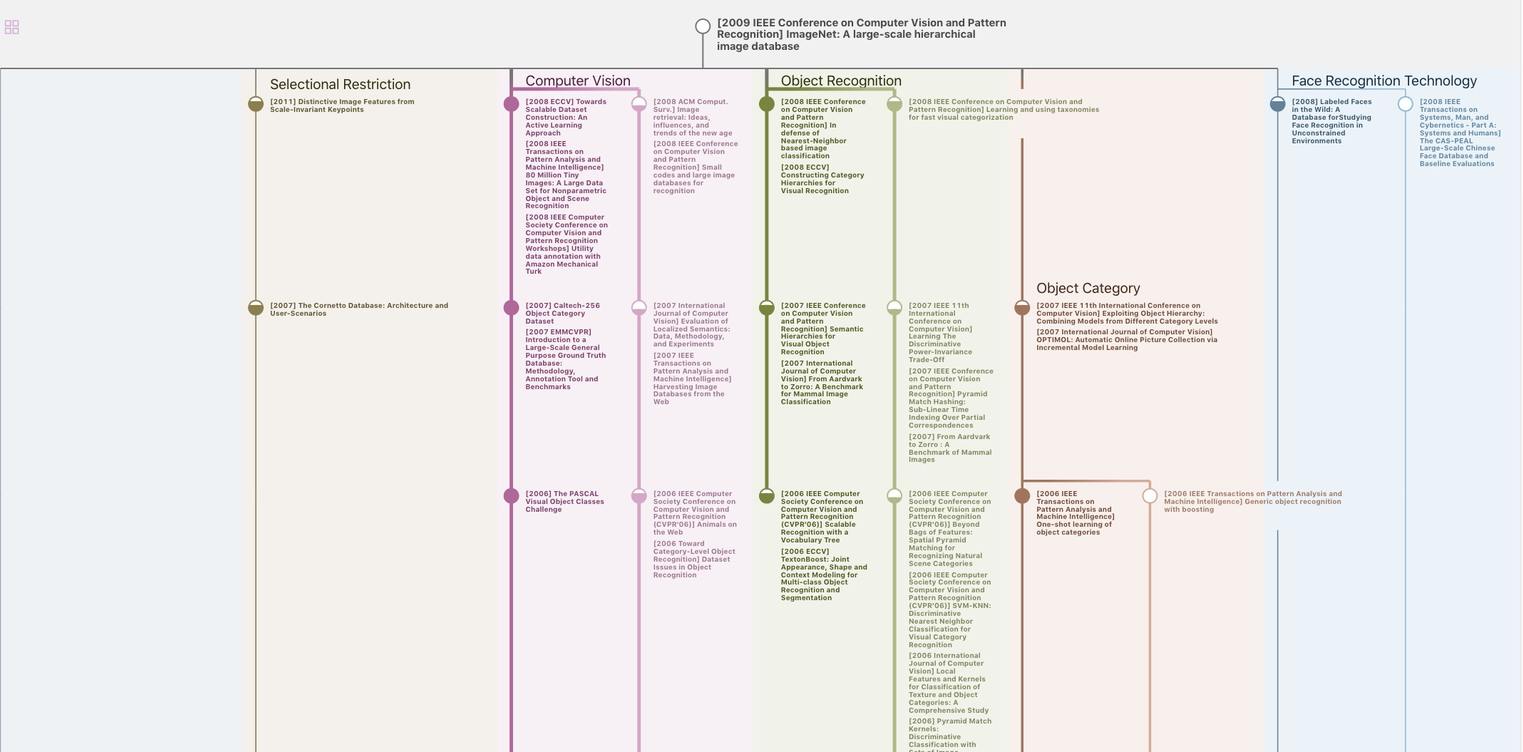
生成溯源树,研究论文发展脉络
Chat Paper
正在生成论文摘要