A Non-parametric Graph Clustering Framework for Multi-View Data
AAAI 2024(2024)
摘要
Multi-view graph clustering (MVGC) derives encouraging grouping results by seamlessly integrating abundant information inside heterogeneous data, and has captured surging focus recently.
Nevertheless, the majority of current MVGC works involve at least one hyper-parameter, which not only requires additional efforts for tuning, but also leads to a complicated solving procedure,
largely harming the flexibility and scalability of corresponding algorithms. To this end, in the article we are devoted to getting rid of hyper-parameters, and devise a non-parametric graph clustering (NpGC) framework to more practically partition multi-view data. To be specific, we hold that hyper-parameters play a role in balancing error item and regularization item so as to form high-quality clustering representations. Therefore, under without the assistance of hyper-parameters, how to acquire high-quality representations becomes the key. Inspired by this, we adopt two types of anchors, view-related and view-unrelated, to concurrently mine exclusive characteristics and common characteristics among views. Then, all anchors' information is gathered together via a consensus bipartite graph. By such ways, NpGC extracts both complementary and consistent multi-view features, thereby obtaining superior clustering results. Also, linear complexities enable it to handle datasets with over 120000 samples. Numerous experiments reveal NpGC's strong points compared to lots of classical approaches.
更多查看译文
关键词
ML: Clustering,ML: Multi-instance/Multi-view Learning
AI 理解论文
溯源树
样例
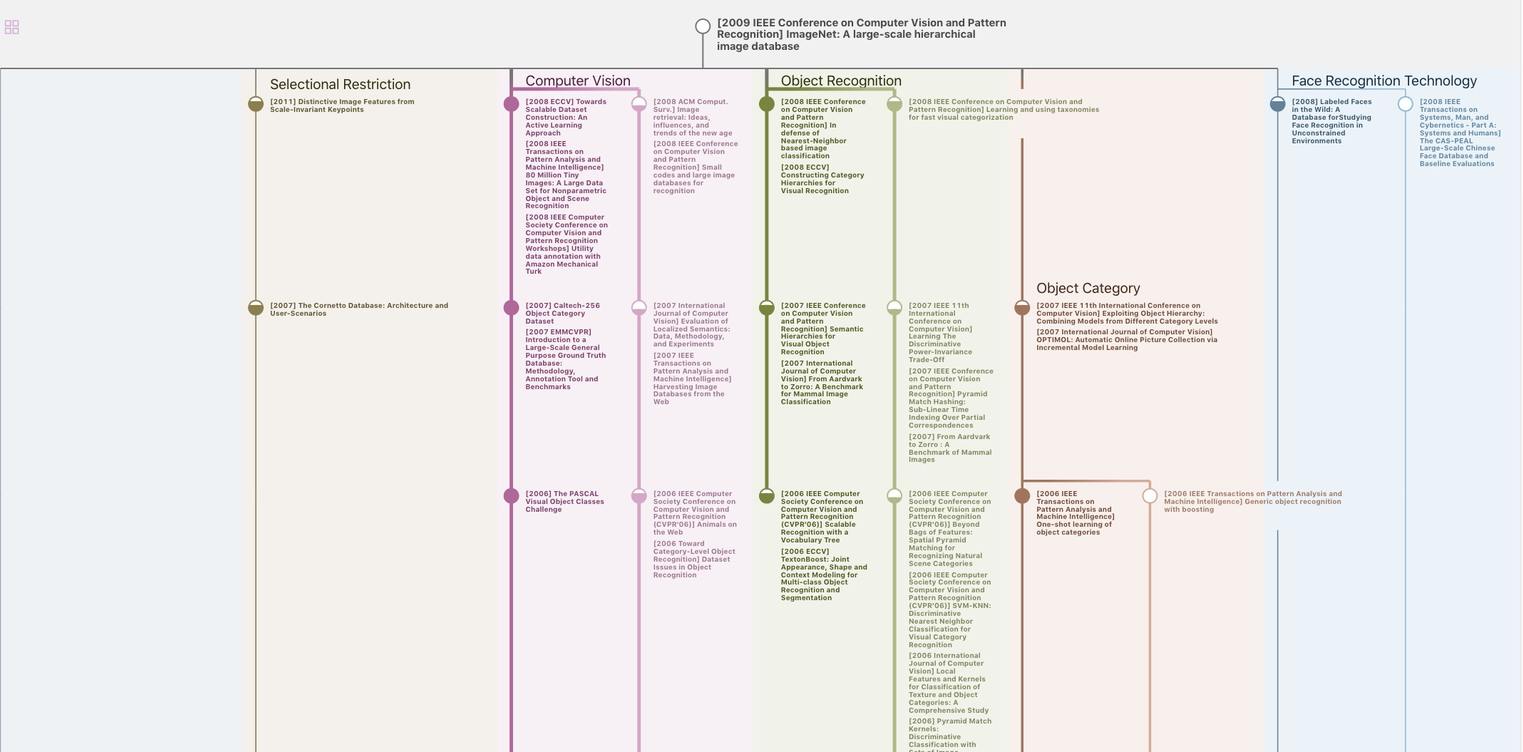
生成溯源树,研究论文发展脉络
Chat Paper
正在生成论文摘要