Depression Detection via Capsule Networks with Contrastive Learning
AAAI 2024(2024)
摘要
Depression detection is a challenging and crucial task in psychological illness diagnosis. Utilizing online user posts to predict whether a user suffers from depression seems an effective and promising direction. However, existing methods suffer from either poor interpretability brought by the black-box models or underwhelming performance caused by the completely separate two-stage model structure. To alleviate these limitations, we propose a novel capsule network integrated with contrastive learning for depression detection (DeCapsNet). The highlights of DeCapsNet can be summarized as follows. First, it extracts symptom capsules from user posts by leveraging meticulously designed symptom descriptions, and then distills them into class-indicative depression capsules. The overall workflow is in an explicit hierarchical reasoning manner and can be well interpreted by the Patient Health Questionnaire-9 (PHQ9), which is one of the most widely adopted questionnaires for depression diagnosis. Second, it integrates with contrastive learning, which can facilitate the embeddings from the same class to be pulled closer, while simultaneously pushing the embeddings from different classes apart. In addition, by adopting the end-to-end training strategy, it does not necessitate additional data annotation, and mitigates the potential adverse effects from the upstream task to the downstream task. Extensive experiments on three widely-used datasets show that in both within-dataset and cross-dataset scenarios our proposed method outperforms other strong baselines significantly.
更多查看译文
关键词
General
AI 理解论文
溯源树
样例
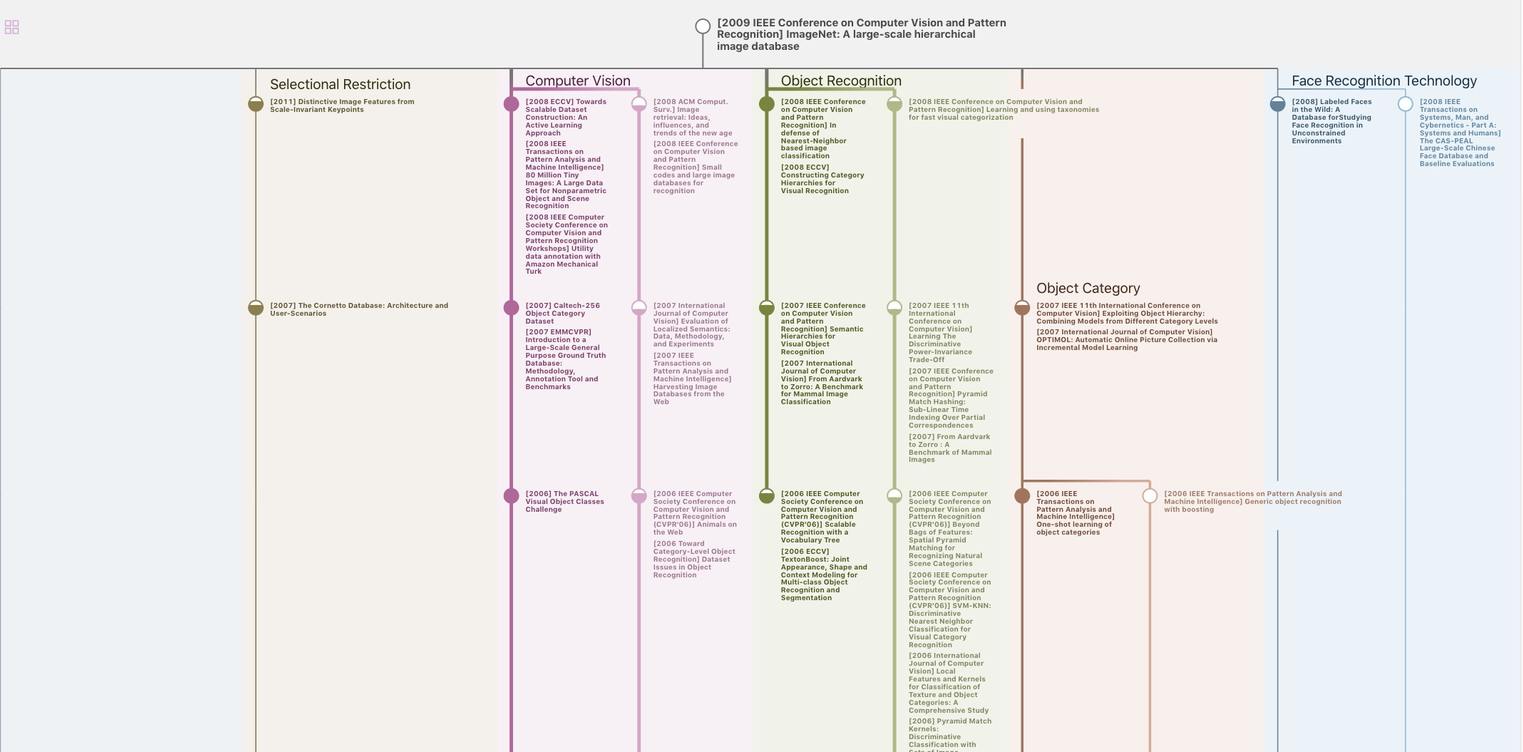
生成溯源树,研究论文发展脉络
Chat Paper
正在生成论文摘要