A Novel Loss Function-based Support Vector Machine for Binary Classification
CoRR(2024)
摘要
The previous support vector machine(SVM) including 0/1 loss SVM, hinge loss
SVM, ramp loss SVM, truncated pinball loss SVM, and others, overlooked the
degree of penalty for the correctly classified samples within the margin. This
oversight affects the generalization ability of the SVM classifier to some
extent. To address this limitation, from the perspective of confidence margin,
we propose a novel Slide loss function (ℓ_s) to construct the support
vector machine classifier(ℓ_s-SVM). By introducing the concept of proximal
stationary point, and utilizing the property of Lipschitz continuity, we derive
the first-order optimality conditions for ℓ_s-SVM. Based on this, we
define the ℓ_s support vectors and working set of ℓ_s-SVM. To
efficiently handle ℓ_s-SVM, we devise a fast alternating direction method
of multipliers with the working set (ℓ_s-ADMM), and provide the
convergence analysis. The numerical experiments on real world datasets confirm
the robustness and effectiveness of the proposed method.
更多查看译文
AI 理解论文
溯源树
样例
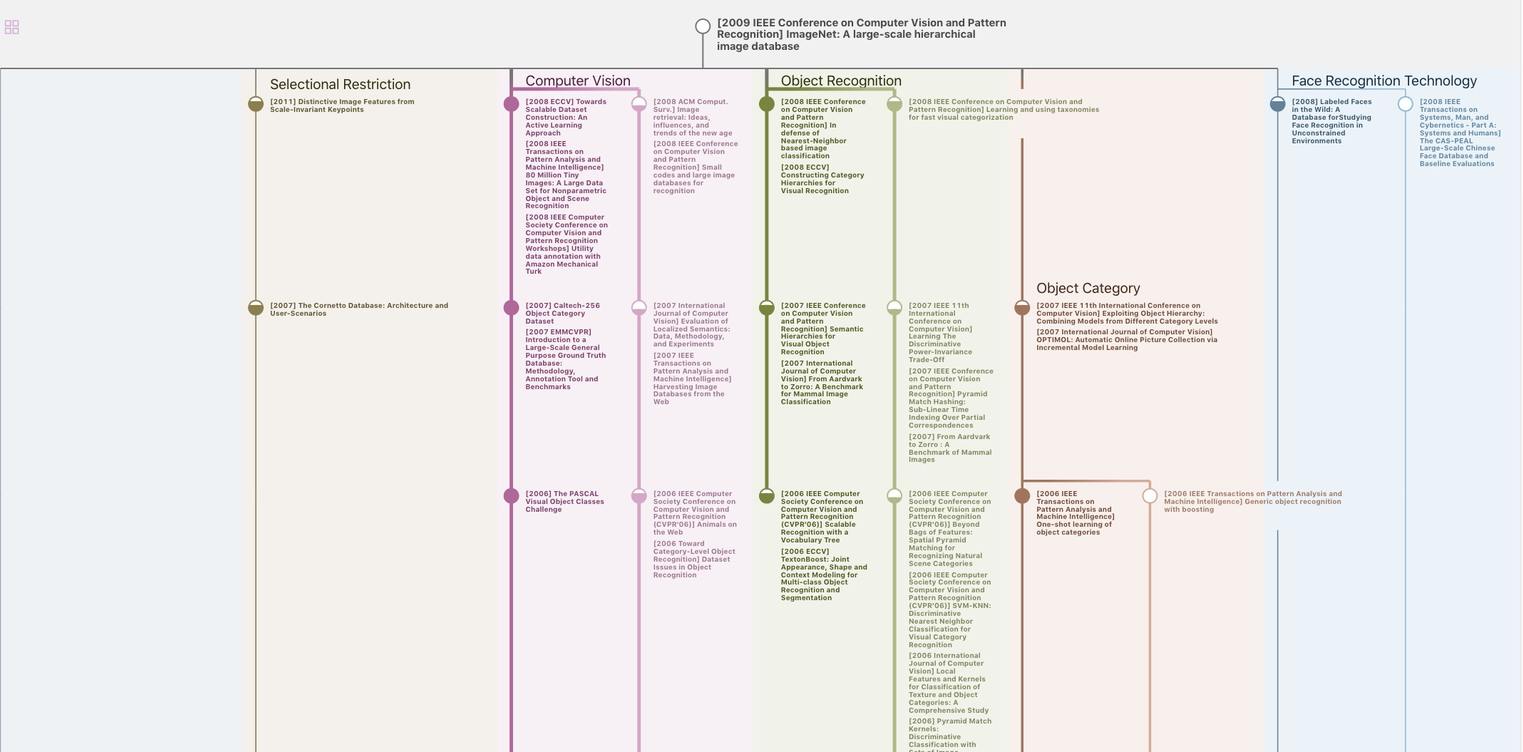
生成溯源树,研究论文发展脉络
Chat Paper
正在生成论文摘要