Multi-task Framework for Vibration-Based Structural Damage Detection of Spatial Truss Structure Using Graph Learning
Journal of Vibration Engineering & Technologies(2024)
摘要
The spatial truss is a special type of structure in civil engineering with striking visualization, used for covering large spaces such as stadiums, commercial centers, and train stations. Due to its multi-component nature and large size, timely monitoring of its structural health is a tedious and challenging mission, for which using vibration signals measured from embedded sensors has shown promising results, reducing significant time and effort compared to manual methods. In order to exploit vibration data more effectively, this study explores a novelty data-driven approach that performs multiple structural damage detection tasks ranging from detecting damage, localizing damage, and quantifying damage severity. The main steps of the proposed approach are: converting truss data, including geometrical information and vibration signal, into graph data, leveraging the graph attention network for spatial-temporal feature extraction, and elaborating a compound loss function for multi-task learning. The proposed approach’s efficiency and efficacy are quantitatively demonstrated via four case studies with increasing levels of complexity. The results show that the detection achieves more than 95
更多查看译文
关键词
Deep learning,Stochastic processes,Forecasting,Structural engineering,Numerical simulation
AI 理解论文
溯源树
样例
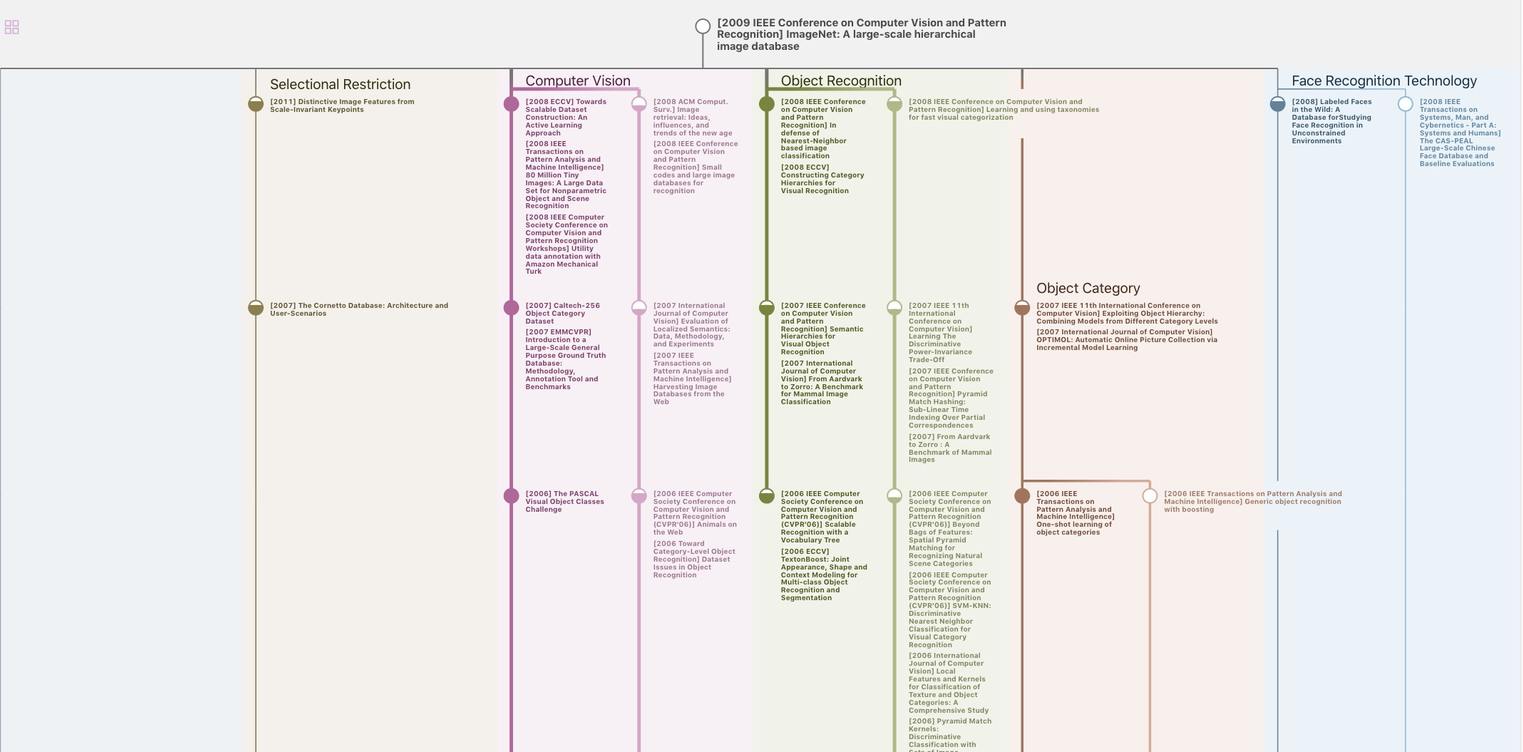
生成溯源树,研究论文发展脉络
Chat Paper
正在生成论文摘要