Fourier Transform-based Estimators for Data Sketches
arxiv(2024)
摘要
In this paper we consider the problem of estimating the f-moment
(∑_v∈ [n] (f(𝐱(v))-f(0))) of a dynamic vector 𝐱∈𝔾^n over some abelian group (𝔾,+), where the
f_∞ norm is bounded. We propose a simple sketch and new estimation
framework based on the Fourier transform of f. I.e., we decompose f
into a linear combination of homomorphisms f_1,f_2,… from
(𝔾,+) to (ℂ,×), estimate the f_k-moment for each
f_k, and synthesize them to obtain an estimate of the f-moment. Our
estimators are asymptotically unbiased and have variance asymptotic to
𝐱_0^2 (f_∞^2 m^-1 + f̂_1^2 m^-2), where
the size of the sketch is O(mlog nlog|𝔾|) bits.
When 𝔾=ℤ this problem can also be solved using
off-the-shelf ℓ_0-samplers with space O(mlog^2 n) bits, which does not
obviously generalize to finite groups. As a concrete benchmark, we extend
Ganguly, Garofalakis, and Rastogi's singleton-detector-based sampler to work
over 𝔾 using O(mlog nlog|𝔾|log(mlog n)) bits.
We give some experimental evidence that the Fourier-based estimation
framework is significantly more accurate than sampling-based approaches at the
same memory footprint.
更多查看译文
AI 理解论文
溯源树
样例
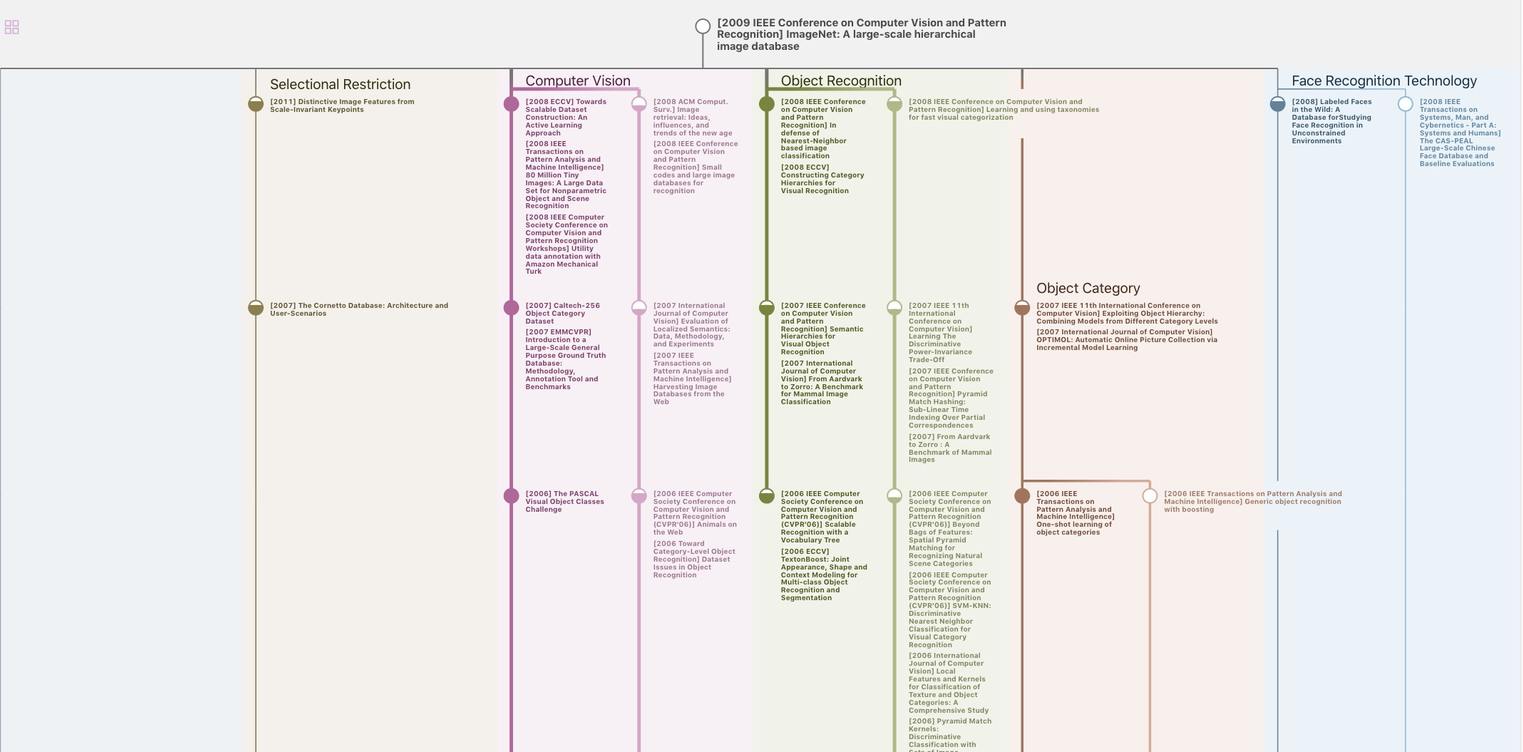
生成溯源树,研究论文发展脉络
Chat Paper
正在生成论文摘要