GCN-DevLSTM: Path Development for Skeleton-Based Action Recognition
arxiv(2024)
摘要
Skeleton-based action recognition (SAR) in videos is an important but
challenging task in computer vision. The recent state-of-the-art models for SAR
are primarily based on graph convolutional neural networks (GCNs), which are
powerful in extracting the spatial information of skeleton data. However, it is
yet clear that such GCN-based models can effectively capture the temporal
dynamics of human action sequences. To this end, we propose the DevLSTM module,
which exploits the path development – a principled and parsimonious
representation for sequential data by leveraging the Lie group structure. The
path development, originated from Rough path theory, can effectively capture
the order of events in high-dimensional stream data with massive dimension
reduction and consequently enhance the LSTM module substantially. Our proposed
G-DevLSTM module can be conveniently plugged into the temporal graph,
complementing existing advanced GCN-based models. Our empirical studies on the
NTU60, NTU120 and Chalearn2013 datasets demonstrate that our proposed hybrid
model significantly outperforms the current best-performing methods in SAR
tasks. The code is available at https://github.com/DeepIntoStreams/GCN-DevLSTM.
更多查看译文
AI 理解论文
溯源树
样例
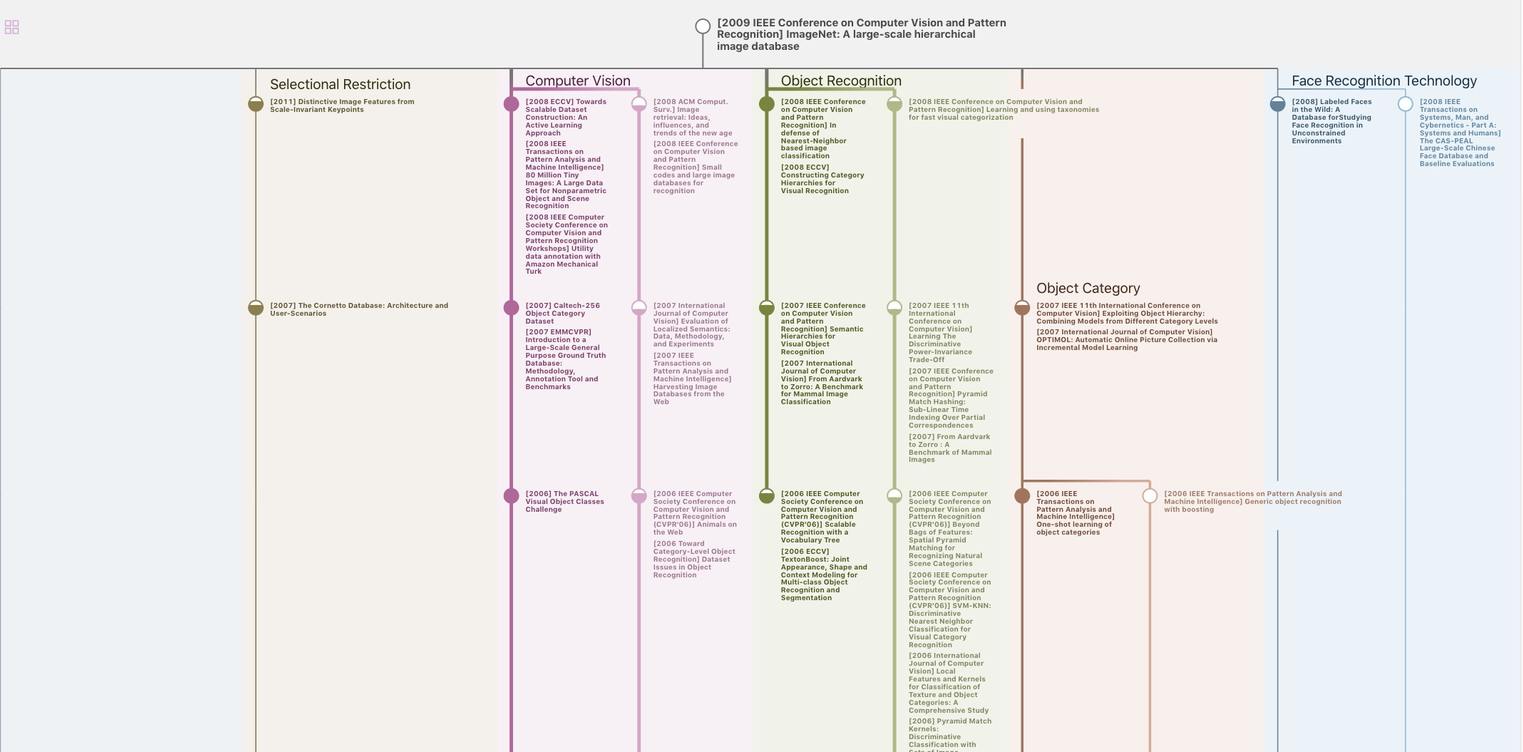
生成溯源树,研究论文发展脉络
Chat Paper
正在生成论文摘要