Low-rank matrix recovery with total generalized variation for defending adversarial examples
Frontiers of Information Technology & Electronic Engineering(2024)
摘要
Low-rank matrix decomposition with first-order total variation (TV) regularization exhibits excellent performance in exploration of image structure. Taking advantage of its excellent performance in image denoising, we apply it to improve the robustness of deep neural networks. However, although TV regularization can improve the robustness of the model, it reduces the accuracy of normal samples due to its over-smoothing. In our work, we develop a new low-rank matrix recovery model, called LRTGV, which incorporates total generalized variation (TGV) regularization into the reweighted low-rank matrix recovery model. In the proposed model, TGV is used to better reconstruct texture information without over-smoothing. The reweighted nuclear norm and L1-norm can enhance the global structure information. Thus, the proposed LRTGV can destroy the structure of adversarial noise while re-enhancing the global structure and local texture of the image. To solve the challenging optimal model issue, we propose an algorithm based on the alternating direction method of multipliers. Experimental results show that the proposed algorithm has a certain defense capability against black-box attacks, and outperforms state-of-the-art low-rank matrix recovery methods in image restoration.
更多查看译文
关键词
Total generalized variation,Low-rank matrix,Alternating direction method of multipliers,Adversarial example,广义全变分,低秩矩阵,交替方向乘子法,对抗样本,TP37
AI 理解论文
溯源树
样例
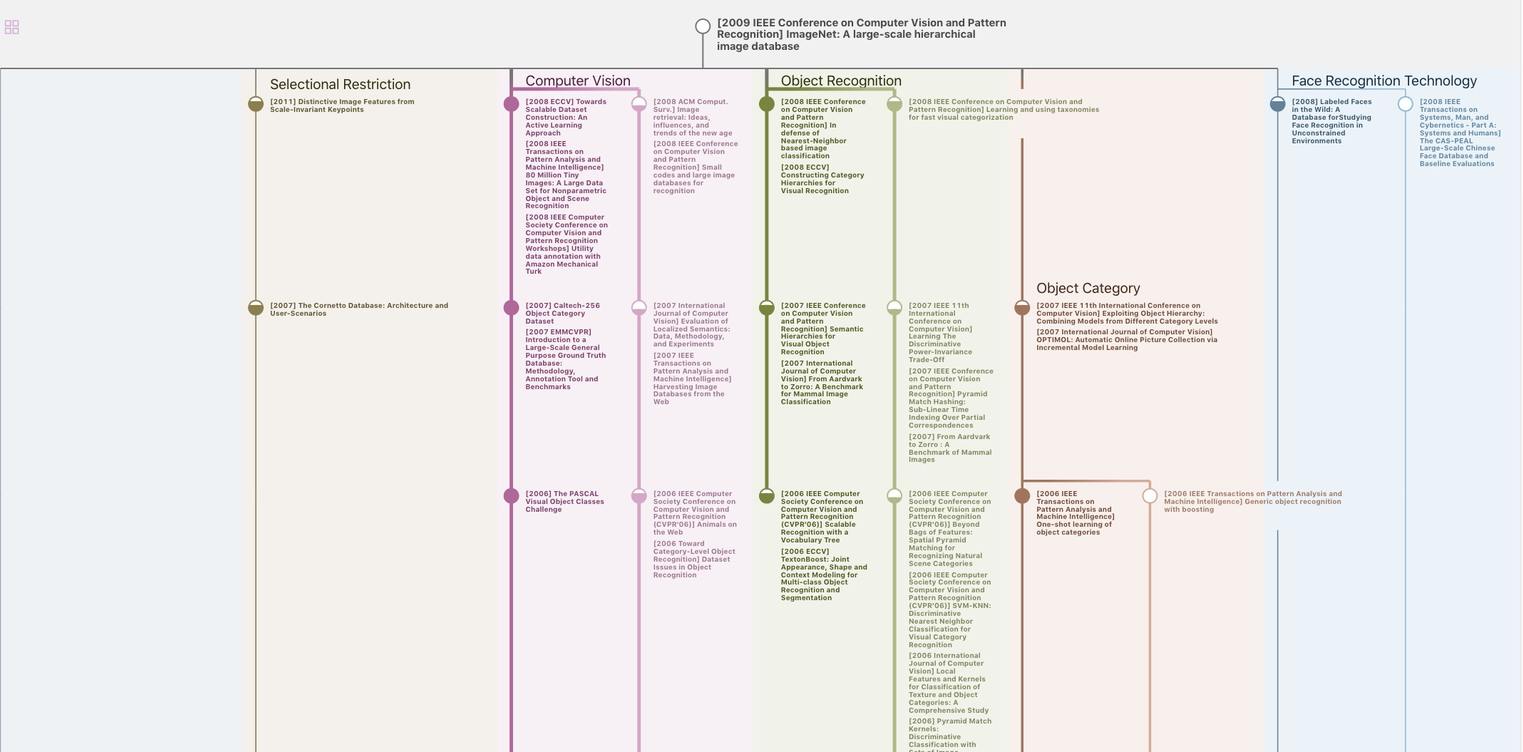
生成溯源树,研究论文发展脉络
Chat Paper
正在生成论文摘要