A multi-granularity hierarchical network for long- and short-term forecasting on multivariate time series data
Applied Soft Computing(2024)
摘要
Multivariate time series forecasting is a significant research problem in many fields such as economics, finance and transportation, where simultaneous long- and short-term forecasting is required. However, current techniques are typically limited to a single short-term or a long-term forecast. To address the limitation, a novel multi-granularity hierarchical network, GNet-LS, is proposed for long- and short-term forecasting on multivariate time series data, which takes into account the separate role of internal correlation and external relationship. First, the original time series sequence is divided into multiple granular sequences based on downsampling, to reduce error accumulation caused by long-term prediction. In order to discover the external relationships between variables, the CNN module slides over the sequence of variables. The global CNN and local CNN are built to implement periodic and nonperiodic extraction, respectively. Next, a self-attention module is used to model dependencies between the output of local CNN and global CNN. The LSTM networks and attention mechanisms are used to mine internal correlation of the target variable on time series. Then, multiple granular external relationships and internal correlation are obtained in parallel. Finally, external relationships and internal correlation are fused together by splicing and overlay to obtain both long-term and short-term forecasts. The experimental results demonstrate that the proposed GNet-LS outperforms a bunch of compared methods in terms of RSE, CORR, MAE and RMSE.
更多查看译文
关键词
Multivariate time series,Multi-granularity,Hierarchical networks,Long- and short-term forecasting
AI 理解论文
溯源树
样例
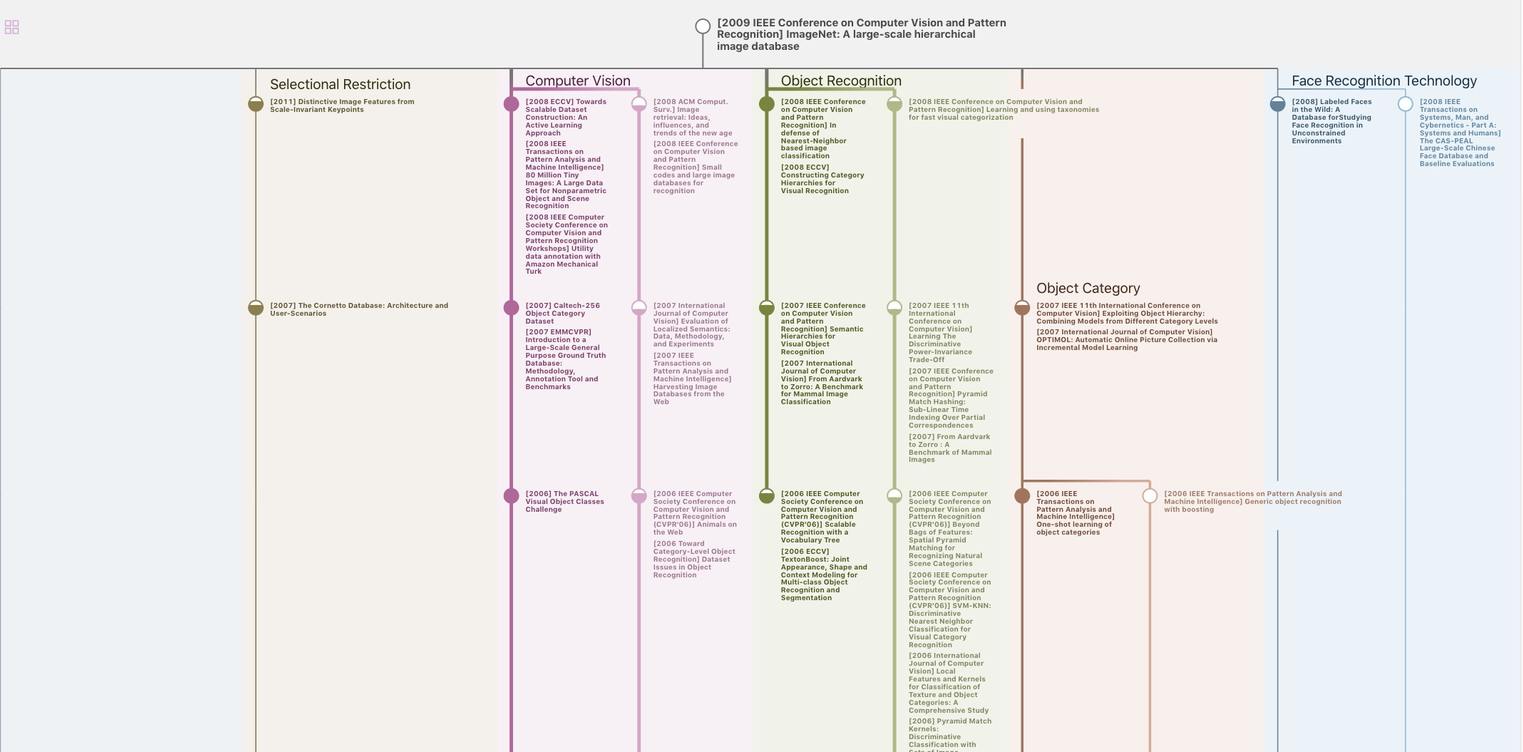
生成溯源树,研究论文发展脉络
Chat Paper
正在生成论文摘要