Abstract 902: Explainable AI: Graph machine learning for response prediction and biomarker discovery
Cancer Research(2024)
摘要
Abstract Accurately predicting drug sensitivity and understanding what is driving it are major challenges in drug discovery. Graphs are a natural framework for capturing diverse pharmacological data for efficacy predictions, thanks to their ability to integrate multimodal data and represent relationships such as gene-gene or drug-target interactions as edges. They have also had proven success across a range of other drug discovery tasks including repositioning and target identification. In this study, we sought to address the explainability challenges of drug response predictions. Recent developments in the field of Graph AI have led to improvements in interpretability mechanisms that highlight parts of a graph which are driving predictions. We have conducted a comprehensive review of multiple major approaches for tackling drug efficacy prediction using graph methods, benchmarking the performance and interpretability of these algorithms across indications. Methods: We assembled a combined dataset of GDSC1 and GDSC2 drug response data in cell lines, with multiomic cell line data and drug target and chemical structure data. We then applied graph-based approaches for the prediction of binarized IC50 on an indication-by-indication basis. Approach 1 involved the creation of a ‘GDSC knowledge graph’, where drug response and cell line ‘omic information is represented in an unweighted knowledge graph: cell lines are connected to genes expressed in them, drugs are connected to genes they target, and so on. We then used state-of-the-art graph embedding techniques to predict IC50 using paired drug and cell line embeddings. In Approach 2 we used a weighted knowledge graph instead, and generated embeddings using heterogeneous graph neural networks (HGNNs). In Approach 3, we modelled response prediction as a graph classification task, where one single graph captures one drug-cell line interaction. The graph classifier and HGNN models both have in-built interpretability mechanisms, including graph attention, that can signify the genes in the cell line which were most important for the eventual prediction. We can also integrate biomedical prior knowledge with all these models by capturing gene-pathway and gene-gene data in the graphs. Results: Our models outperformed benchmark models including DNNs and GBMs, and identified both established and novel response biomarkers in NSCLC cell lines (AUC = 0.94, Accuracy = 89%). We have also applied our models to Breast Cancer, Pancreatic Cancer, Colorectal Cancer and Haematological malignancies with similar predictive performance and explainability. Conclusions:Our graph analytical framework for response predictions showed better performance than benchmarking models and provided insights from explainability. This framework is easily extendable to response and ‘omic data from any disease model and patient studies. Citation Format: Jake Cohen-Setton, Krishna Bulusu, Jonathan Dry, Ben Sidders. Explainable AI: Graph machine learning for response prediction and biomarker discovery [abstract]. In: Proceedings of the American Association for Cancer Research Annual Meeting 2024; Part 1 (Regular Abstracts); 2024 Apr 5-10; San Diego, CA. Philadelphia (PA): AACR; Cancer Res 2024;84(6_Suppl):Abstract nr 902.
更多查看译文
AI 理解论文
溯源树
样例
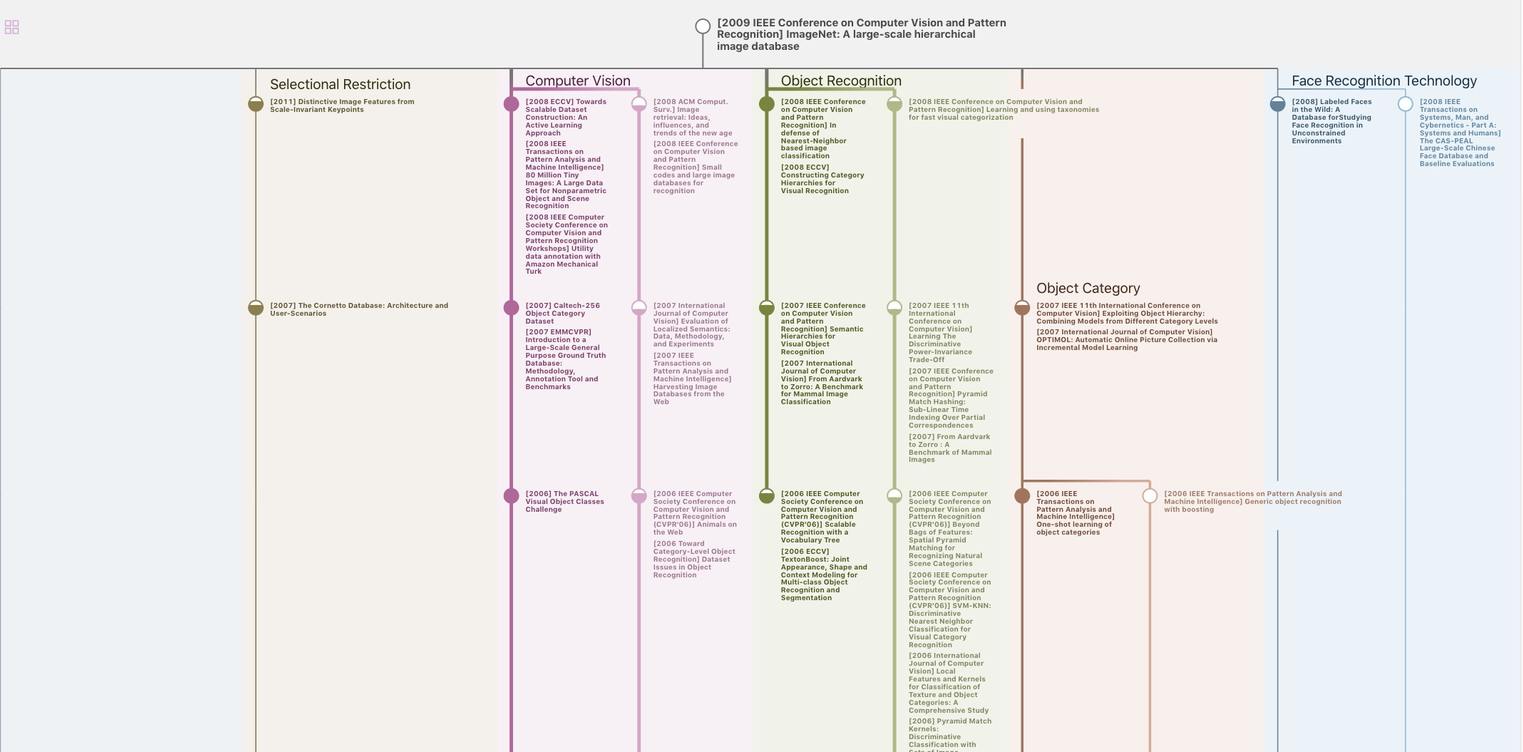
生成溯源树,研究论文发展脉络
Chat Paper
正在生成论文摘要