Machine learning analysis using RNA-seq to distinguish neuromyelitis optica from multiple sclerosis and identify therapeutic candidates
The Journal of Molecular Diagnostics(2024)
摘要
This study aims to identify RNA biomarkers distinguishing neuromyelitis optica (NMO) from relapsing-remitting multiple sclerosis (RRMS) and explore potential therapeutic applications leveraging machine learning (ML). An ensemble approach was developed using differential gene expression analysis and competitive ML methodologies, interrogating total RNA sequencing datasets from peripheral whole blood of treatment-naïve RRMS and NMO patients and healthy individuals. Pathway analysis of candidate biomarkers informed the biological context of disease, transcription factor activity, and small-molecule therapeutic potential. ML models differentiated between NMO and RRMS patients, with the performance of certain models exceeding 90% accuracy. RNA biomarkers driving model performance were associated with ribosomal dysfunction and viral infection. Regulatory networks of kinases and transcription factors informed biological context and identified potential therapeutic targets. Small-molecule candidates capable of reversing perturbed gene expression were uncovered. Two small molecules identified, mitoxantrone and vorinostat, reinforced discovered expression signatures and highlighted the potential to identify new therapeutic candidates, as their use in NMO patients and experimental autoimmune encephalomyelitis have been previously described. Putative RNA biomarkers were identified that accurately distinguish NMO from RRMS and healthy individuals. The application of multivariate approaches in analysis of RNA sequencing data enhances the discovery of unique RNA biomarkers, accelerating the development of new methods for disease detection, monitoring, and therapeutics. Integrating biological understanding enhances detection of disease-specific signatures and possible therapeutic targets.
更多查看译文
AI 理解论文
溯源树
样例
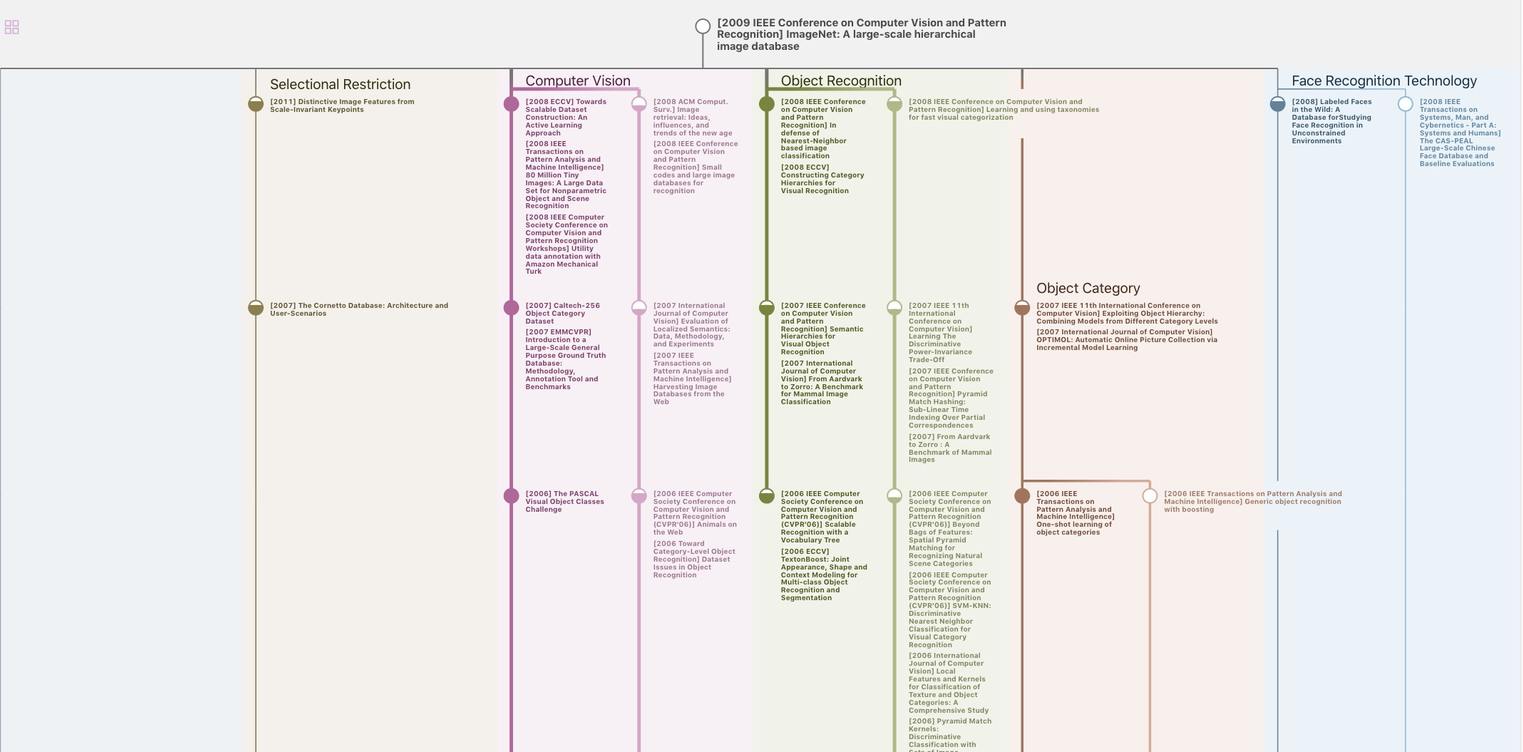
生成溯源树,研究论文发展脉络
Chat Paper
正在生成论文摘要