Sparse Graph Neural Networks for Two-Timescale Wireless Resource Allocation.
GLOBECOM (Workshops)(2023)
摘要
In this paper, we investigate the use of graph isomorphism networks (GINs), one of the most expressive graph neural networks, to solve large-scale radio resource management problems. Due to the fast fading characteristics of wireless channels, reporting full channel state information (CSI) of all users frequently in a dense wireless network can result in huge transmission overhead. In response, we develop a two-timescale resource allocation method based on GINs that leverages slowly-varying large-scale fading CSI of all links along with fast-changing small-scale fading CSI for only part of the interference links, which we term SGIN. Specifically, we model the wireless network as an interference graph and require each user to report large-scale fading CSI of all links to the base station while using
$l_{0}-\mathbf{norm}$
regularization to identify a small amount of important interfering links, whose small-scale fading CSI will then also be reported. Unsupervised training is performed based on this collection of CSI with two time scales to optimize power control in dense networks. The superior performance of SGIN has been demonstrated experimentally. In particular, SGIN can reduce about 67% of the transmission overhead when reporting small-scale fading CSI while maintaining performance comparable to that of a GIN with full CSI.
更多查看译文
关键词
Radio resource management,graph neural networks,sparsity learning,wireless networks
AI 理解论文
溯源树
样例
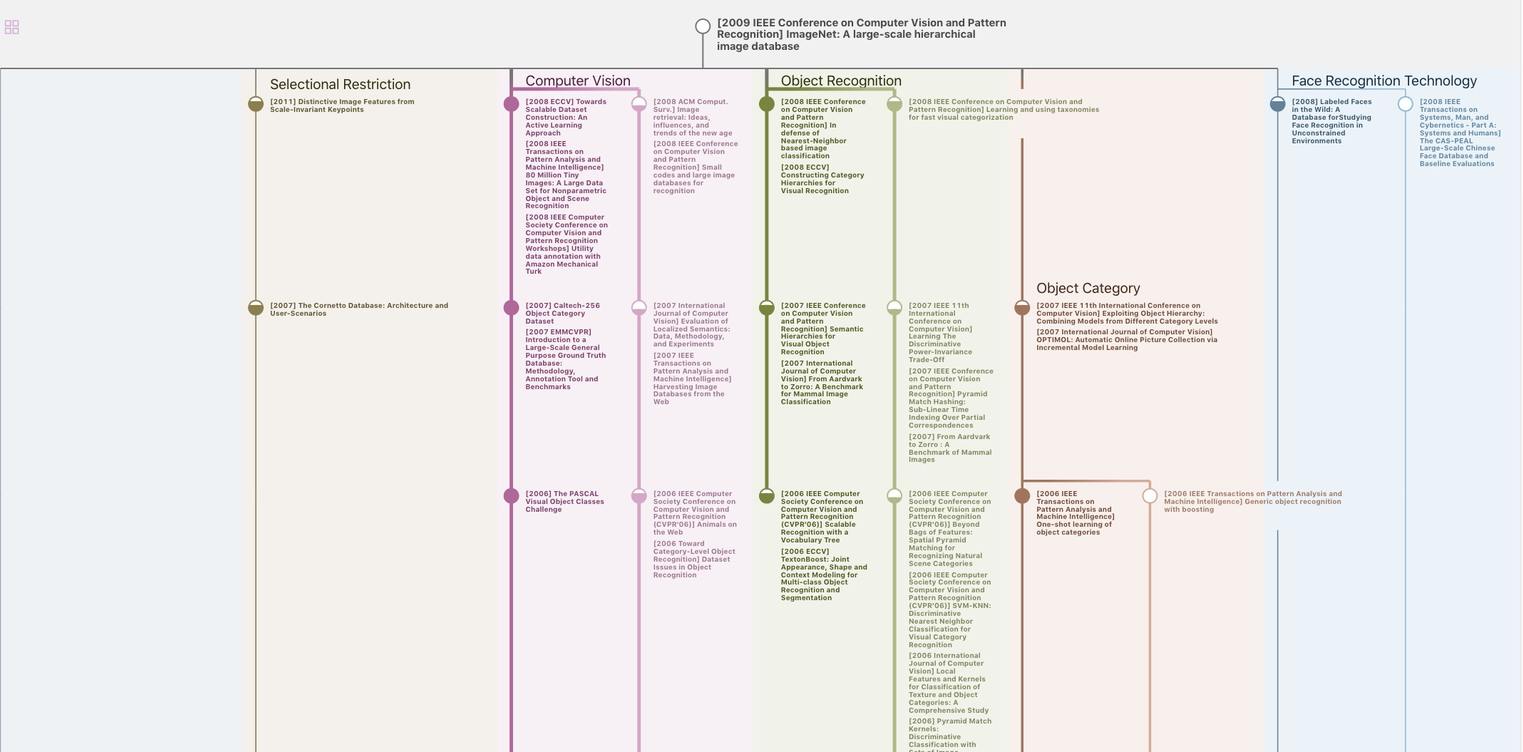
生成溯源树,研究论文发展脉络
Chat Paper
正在生成论文摘要