Discriminative Multi-View Fusion via Adaptive Regression
IEEE Transactions on Emerging Topics in Computational Intelligence(2024)
摘要
Data fusion has become an important task in multi-view learning. Previous methods suffer from the insufficient data fusion due to the following issues: (i) Several methods ignore the correlation and distinction among views and directly concatenate the features from different views; (ii) They involve intractable parameters to balance different views, degenerating the applicability of models; (iii) A fixed label matrix is used to guide feature fusion, overlooking the distances between different classes (i.e., inter-class distance) or within the same class (i.e., intra-class compactness). To overcome these problems, a novel fusion model is proposed to discriminate different views and samples in an adaptive manner, so as to effectively reduce the adverse impacts of low-quality views and outliers. In contrast to existing methods, a flexible regression target is designed to take full advantage of the label information of data, such that both the inter-class distance and the intra-class compactness are preserved. Benefiting from this, a compact and discriminative representation of multiple views is learned to maintain the consistent and complementary information of diverse views. Extensive experiments validate the effectiveness and the superiority of our proposed model.
更多查看译文
关键词
Adaptive regression,classification,feature projection,multi-view fusion,multi-view learning
AI 理解论文
溯源树
样例
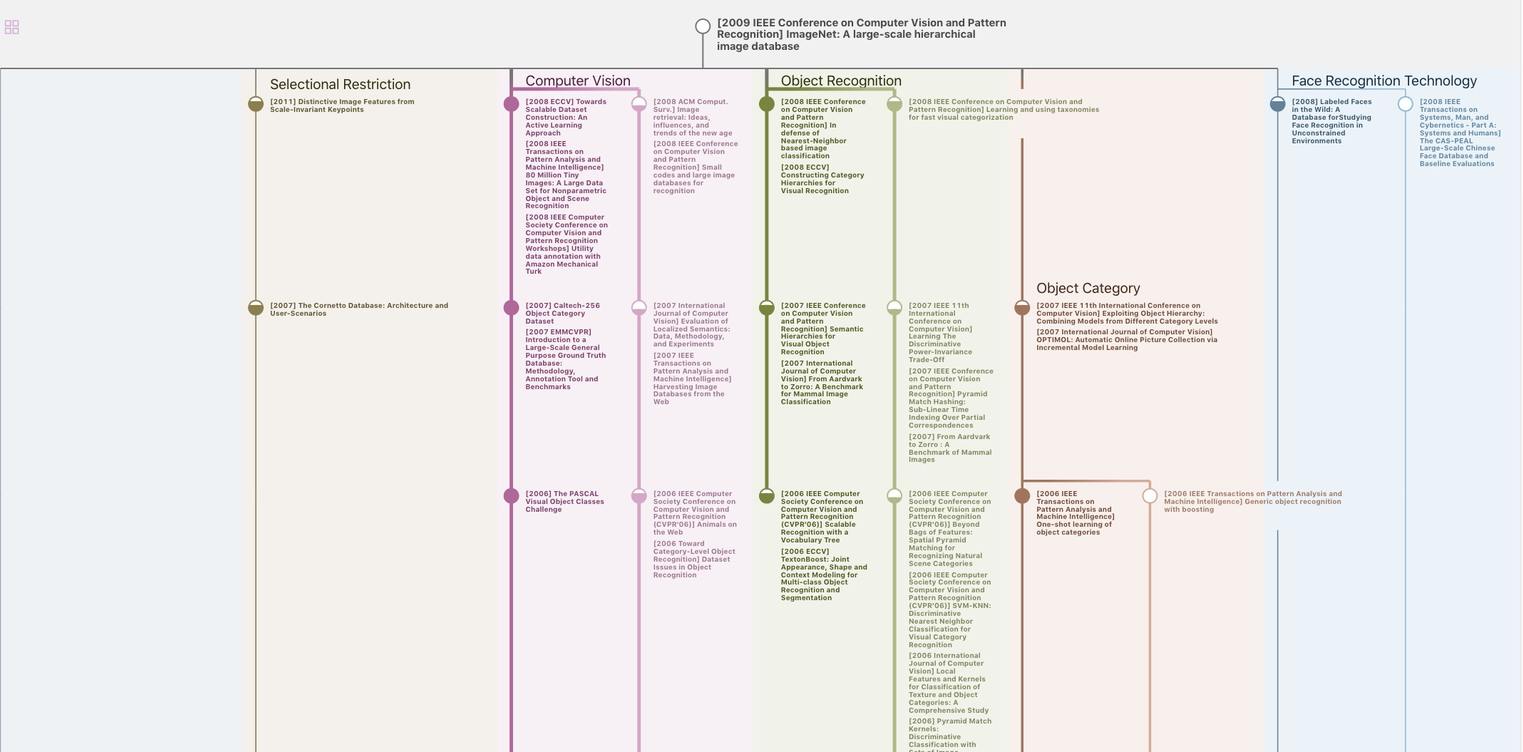
生成溯源树,研究论文发展脉络
Chat Paper
正在生成论文摘要