CNN and Random Forest-Based Tomato Disease Urgency Prediction: A Vital Tool for Agriculture
2024 International Conference on Intelligent and Innovative Technologies in Computing, Electrical and Electronics (IITCEE)(2024)
摘要
The study's main findings and performance metrics, which were obtained after a thorough examination of the classification model, are presented in the abstract. These results are based on the provided table of recall, accuracy, F1-Score, & support values at various Urgency levels. With continuous good precision, recall, or F1-Score values at all Urgency levels, the classification model performs admirably. The model's accuracy and usefulness in making exact predictions while capturing a significant share of real positive results are highlighted by this consistency. The table's support values also show that this data set is evenly distributed, demonstrating a fair representation of each class. The classification model achieves an average F1-Score of roughly 91.91% across various measures, namely Micro Average, Macro Average, and therefore Weighted Average, and achieves an overall accuracy of 98%. The accuracy with which the model can predict the levels of Urgency for plant diseases is highlighted by these data. This outstanding performance has promising agricultural applications, especially in the early identification and categorization of plant illnesses, which are essential for prompt interventions and efficient disease control. The dataset includes 9,096 photos that represent different plant illnesses and situations. This model's overall accuracy of 82.78% demonstrates how well it can foretell various plant diseases & their degree of urgency. Overall, the study's findings significantly advance the science of plant disease diagnostics by pointing at potential real-world applications as well as promising areas for further investigation.
更多查看译文
关键词
Precision,Diseases,Agriculture,Class
AI 理解论文
溯源树
样例
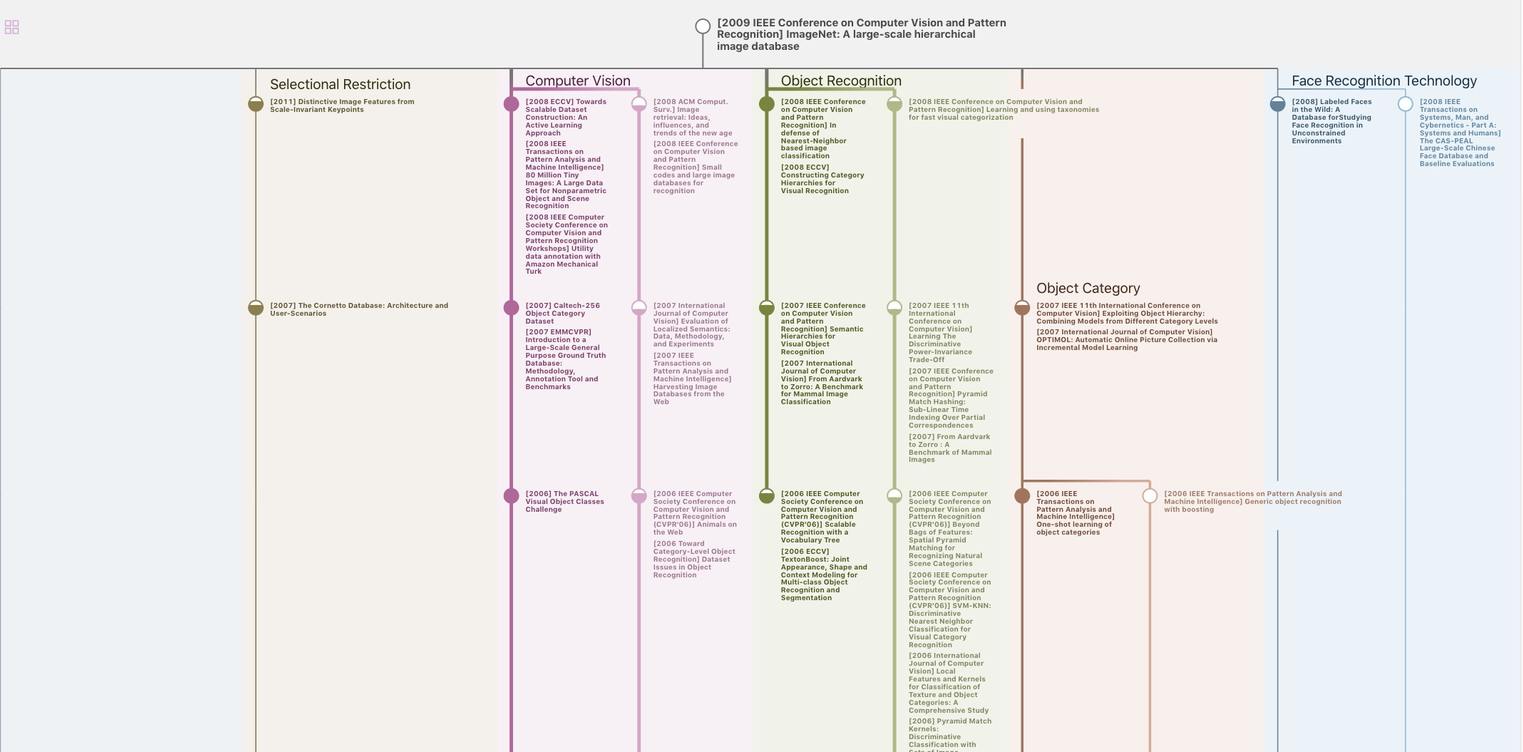
生成溯源树,研究论文发展脉络
Chat Paper
正在生成论文摘要