Optimisation strategies for directed evolution without sequencing
crossref(2024)
摘要
Directed evolution can enable engineering of biological systems with minimal knowledge of their underlying sequence-to-function relationships. A typical directed evolution process consists of iterative rounds of mutagenesis and selection that are designed to steer changes in a biological system (e.g. a protein) towards some functional goal. Much work has been done, particularly leveraging advancements in machine learning, to optimise the process of directed evolution. Many of these methods, however, require DNA sequencing and synthesis, making them resource-intensive and incompatible with developments in targeted in vivo mutagenesis. Operating within the experimental constraints of established sorting-based directed evolution techniques (e.g. Fluorescence-Activated Cell Sorting, FACS), we explore approaches for optimisation of directed evolution that do not require sequencing information. We then expand our methods to the context of emerging experimental techniques in directed evolution, which allow for single-cell selection based on fitness objectives defined from any combination measurable traits. Finally, we validate the developed selection strategies on the GB1 empirical landscape, demonstrating that they can lead to up to a 7.5 fold increase in the probability of attaining the global fitness peak.
更多查看译文
AI 理解论文
溯源树
样例
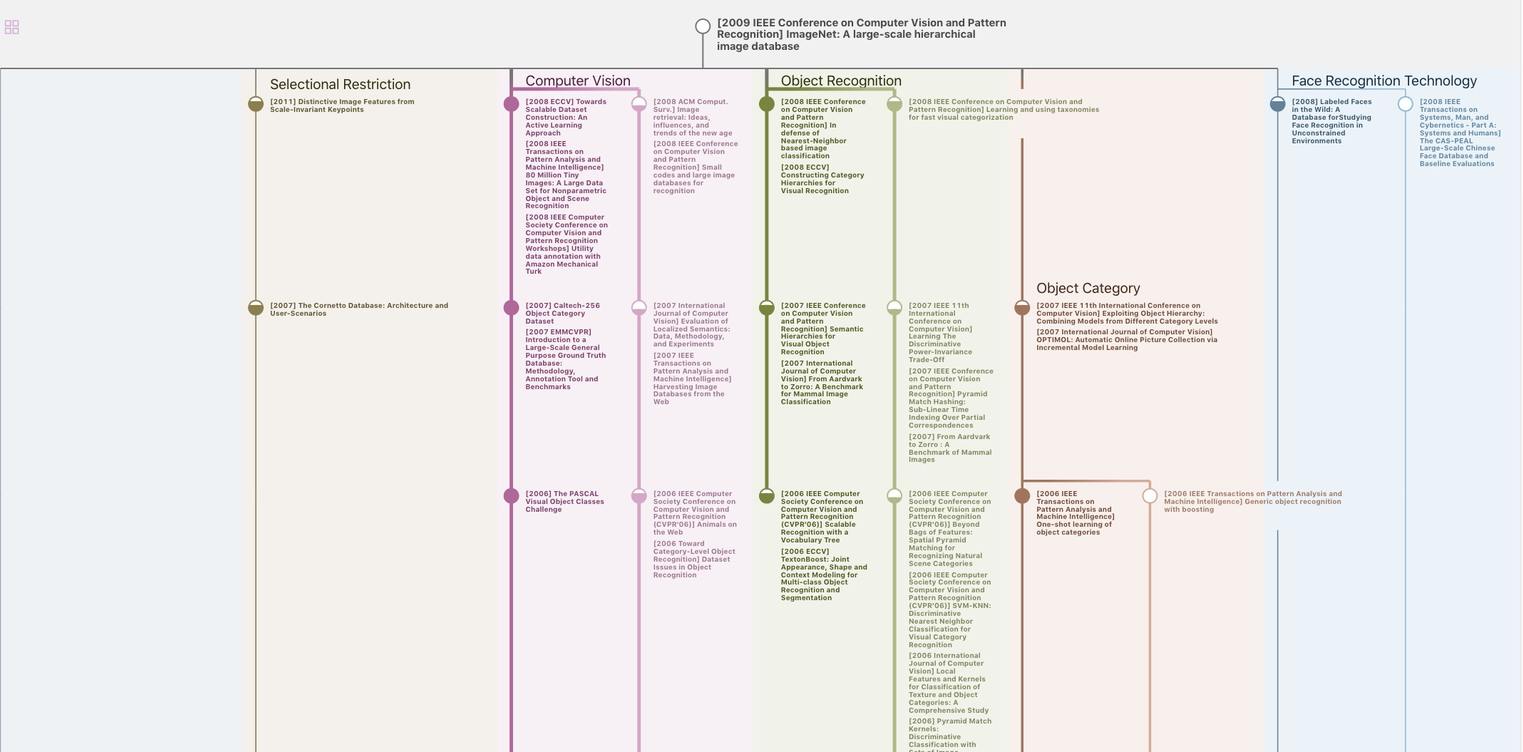
生成溯源树,研究论文发展脉络
Chat Paper
正在生成论文摘要