Applying Neural Network for Extending Cavity Perturbation: Extraction of Complex Permittivity in Non-Linear Region
2023 IEEE Microwaves, Antennas, and Propagation Conference (MAPCON)(2023)
摘要
To ensure precise modeling and simulation of microwave circuits and devices using CAD tools, it is essential to have a thorough knowledge of the dielectric properties of microwave substrates. However, in nonlinear regions, inaccurate characterization can occur as electromagnetic perturbation assumptions get invalid. The paper presents a novel neural network (NN) model, for accurately characterizing the dielectric materials’ properties under nonlinear region: the relative permittivity and loss tangent using a cylindrical cavity resonator operating in TM
010
mode. The proposed technique provides a complete characterization of the dielectric sample in both conventional (linear) and extended (non-linear) regions of the cavity perturbation. The NN model underwent training utilizing Levenberg Marquardt algorithm with a comprehensive dataset of approximately 4 million samples containing resonant frequency, quality factor, 3-dB bandwidth, insertion loss, reflection loss. This dataset was derived from the cylindrical cavity model, which incorporated diverse dielectric samples exhibiting varying relative permittivity and loss factor, all for a given sample thickness. Subsequently, the model underwent validation to assess its accuracy across a broad range of dielectric constants, loss tangent, and pragmatic thickness of the sample. The proposed model achieved an accuracy exceeding 99 %, underscoring its effectiveness in predicting the dielectric properties of different materials.
更多查看译文
关键词
Cylindrical cavity,neural networks,non-linearity,resonant technique
AI 理解论文
溯源树
样例
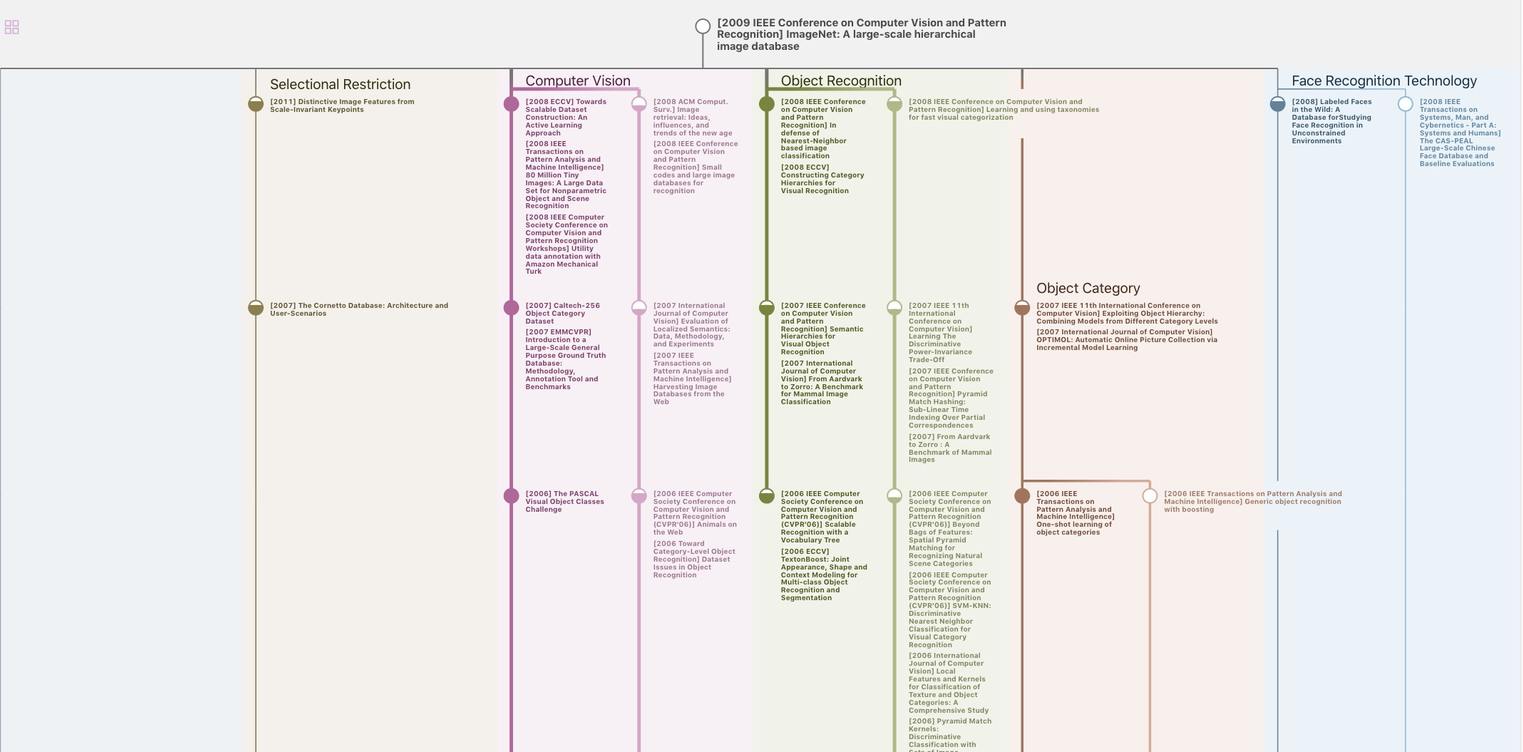
生成溯源树,研究论文发展脉络
Chat Paper
正在生成论文摘要