Efficient Federated Learning via Joint Communication and Computation Optimization
IEEE Transactions on Vehicular Technology(2024)
摘要
Federated learning (FL) is a promising distributed framework that a server leverages a large number of clients to collaboratively learn a model while keeping the privacy of their local data. However, the heterogeneity of computation and communication resources among clients, which maybe lead to the different time arriving at the server, has a negative effect on the convergence of FL algorithms because the stragglers heavily slow down the runtime. In this paper, we develop a joint optimization model of client central processing unit (CPU) frequency, channel assignment, power allocation and local model accuracy based on a designated global model accuracy to minimize the maximum delay of communication and computation of all participating clients. Unfortunately, this problem is a non-convex mixed-integer programming, which is NP-hard. Thus, an equivalent problem by transforming discrete variables into continuous ones is first developed in order to make the original problem tractable. Then we propose a novel Alternating Momentum Gradient Descent with Variable Similarity (AMGDVS) algorithm to solve the min-max problem, which incorporates a similarity index into the previous partial gradients so as to consider the interaction of variables. The proposed AMGDVS algorithm converges to the first-order stationary point with the accuracy factor being
$\eta$
within
$\mathcal {O}(\eta ^{-4})$
iterations. Our simulations, conducted under various parameter settings, demonstrate the effectiveness of the proposed algorithm. Furthermore, the average training time for learning an FL model on FMNIST and CIFAR-10 datasets, both in the Independent Identically Distributed (IID) and Non-Independent Identically Distributed (Non-IID) scenarios, is noticeably reduced.
更多查看译文
关键词
Federated Learning,Joint Communication and Computation Optimization,Channel Assignment,Gradient Descent with Momentum
AI 理解论文
溯源树
样例
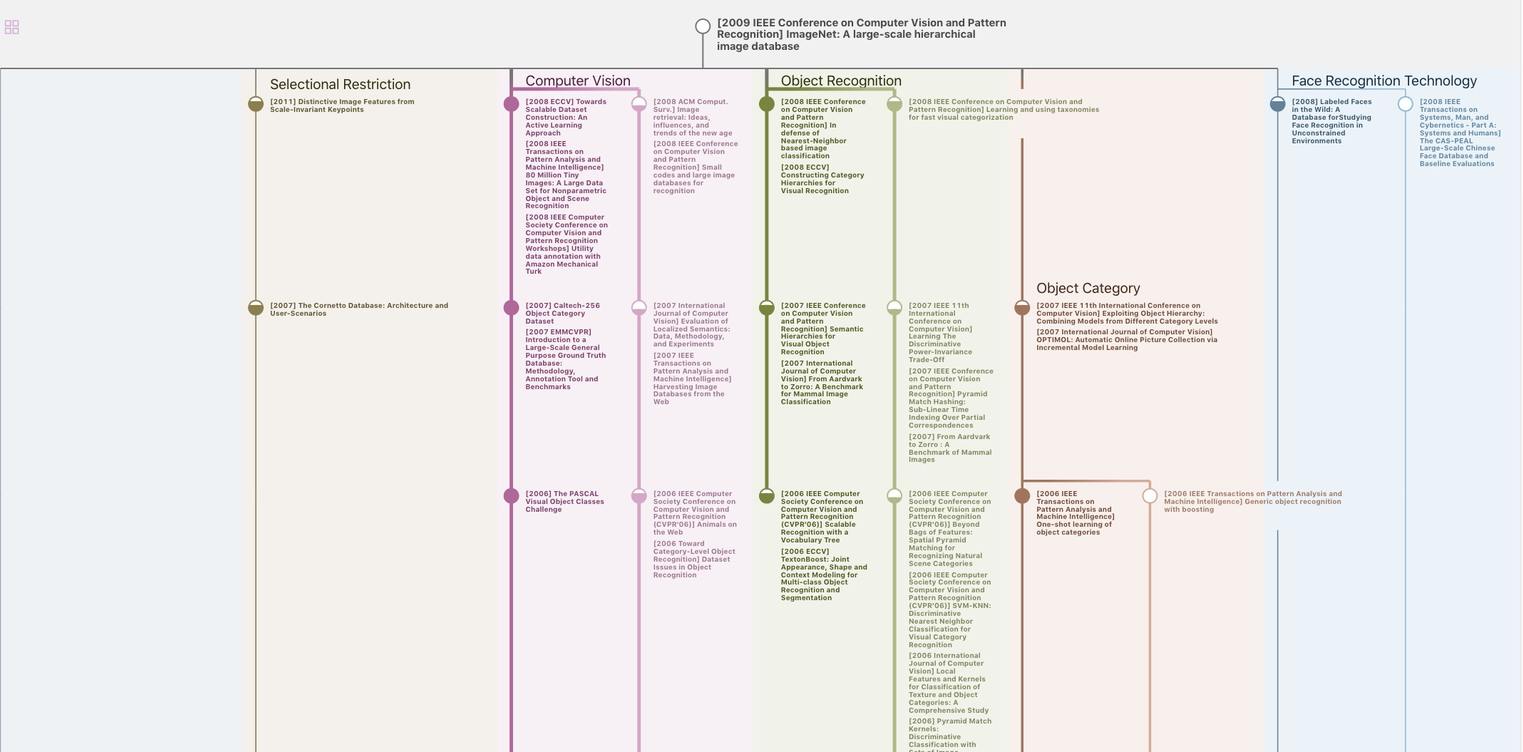
生成溯源树,研究论文发展脉络
Chat Paper
正在生成论文摘要