Simulated Task Oriented Dialogues for Developing Versatile Conversational Agents.
European Conference on Advances in Information Retrieval(2024)
AI 理解论文
溯源树
样例
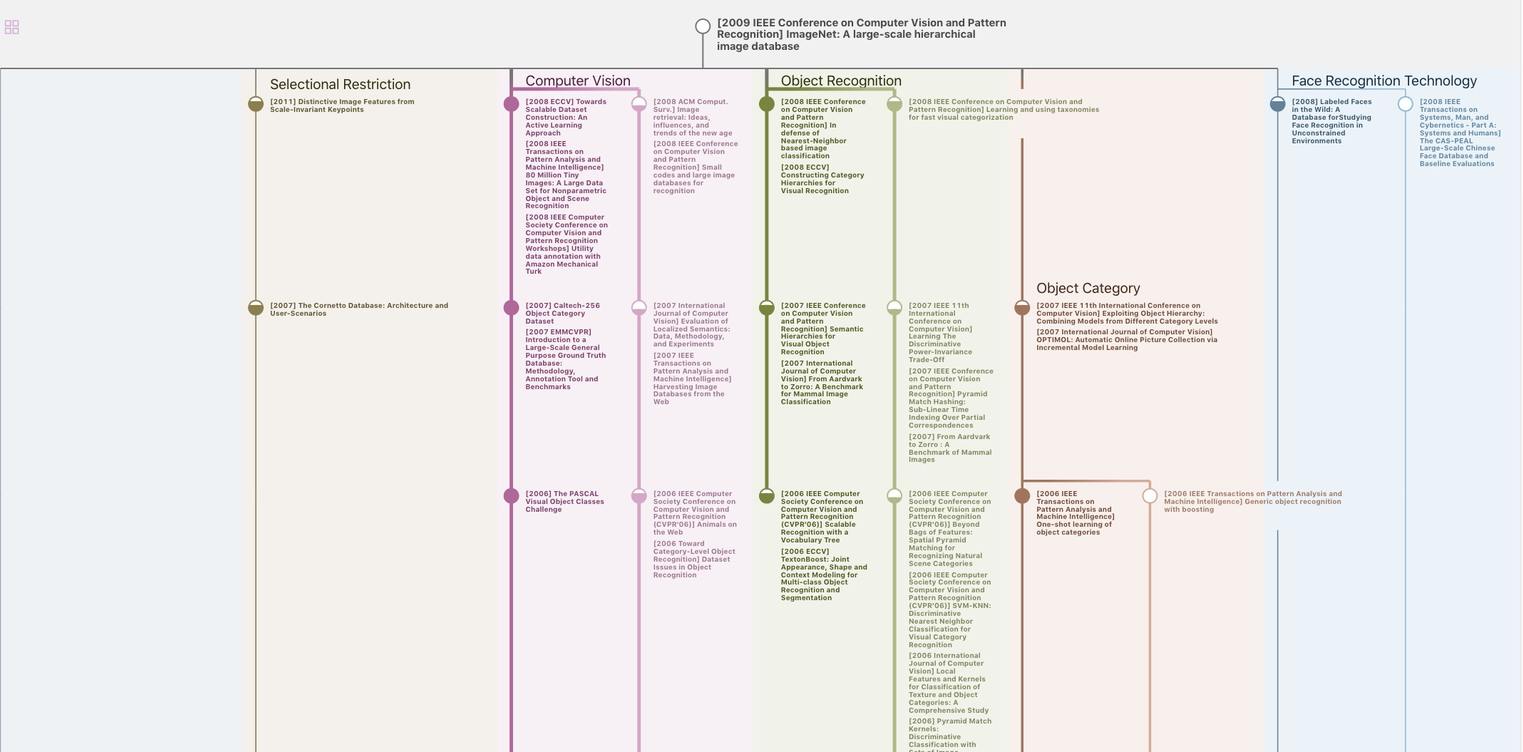
生成溯源树,研究论文发展脉络
Chat Paper
正在生成论文摘要
European Conference on Advances in Information Retrieval(2024)