Multi -View Clustering Using Sparse Non-Negative Matrix Factorization for Recommendation Systems.
International Conference on Machine Learning and Applications(2023)
摘要
Real-world datasets often comprise multiple views or representations, such as user profiles, comments, and preferences. Integrating information from these multi-view datasets can significantly enhance recommendation performance. Consequently, several multi-view clustering (MVC) approaches have been proposed and extensively applied to exploit the compatibility of diverse data sources. Among these methods, Non-negative Matrix Factorization (NMF) has garnered significant attention due to its ability to process high-dimensional data. However, most NMF-based MVC methods suffer from two critical drawbacks: the similarity matrices constructed using traditional methods fail to fully exploit neighborhood information among data points, and they are highly sensitive to noise, missing values, and outliers. Additionally, existing NMF-based clustering methods often do not generate sparse coefficient matrices, leading to suboptimal data representation based on the basis matrix. To address these challenges and enhance the accuracy and efficiency of recommendation algorithms, we propose a novel approach called Multi-View Clustering based on Sparse Non-Negative Matrix Factorization (MVC-SNMF). This method leverages the manifold structure of multi-view data and incorporates a sparsity constraint to effectively handle noise while preserving the geometric structure. Furthermore, we introduce a sparsity constraint on the rows of the coefficient matrix to enhance the visibility of clustering structures. To efficiently solve the proposed non-convex optimization problem, we devise an iterative update scheme. Our experimental results on real datasets from Last.fm and Yelp demonstrate the effectiveness of our approach in improving clustering performance, surpassing most existing clustering algorithms. The proposed method not only enhances the clustering accuracy but also exhibits robustness to noise and missing values, making it a promising solution for practical recommendation systems.
更多查看译文
AI 理解论文
溯源树
样例
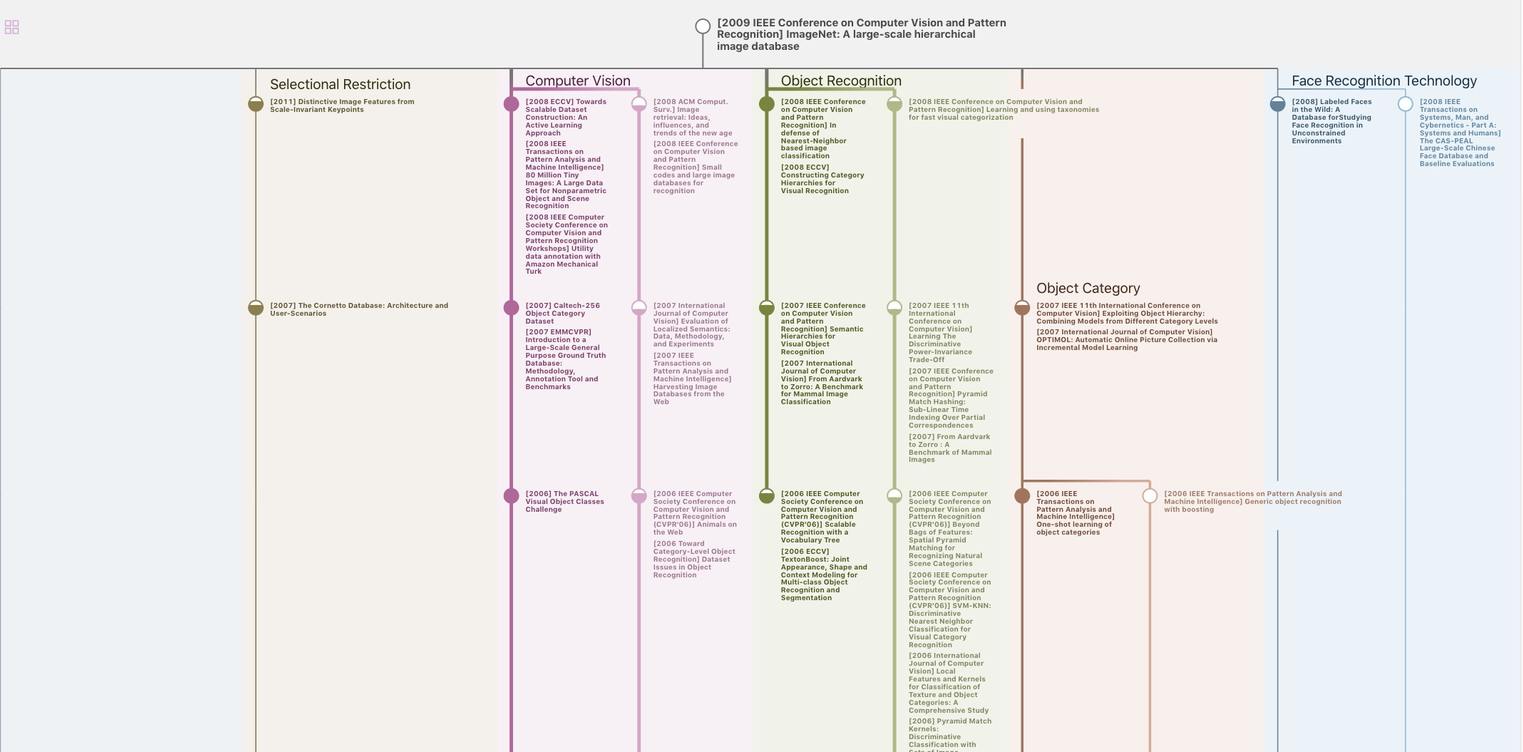
生成溯源树,研究论文发展脉络
Chat Paper
正在生成论文摘要