Gut Microbiota As Mediator and Moderator Between Hepatitis B Virus and Hepatocellular Carcinoma: A Prospective Study.
CANCER MEDICINE(2024)
关键词
gut-liver axis,gut microbiota,hepatitis B virus,hepatocellular carcinoma,machine learning
AI 理解论文
溯源树
样例
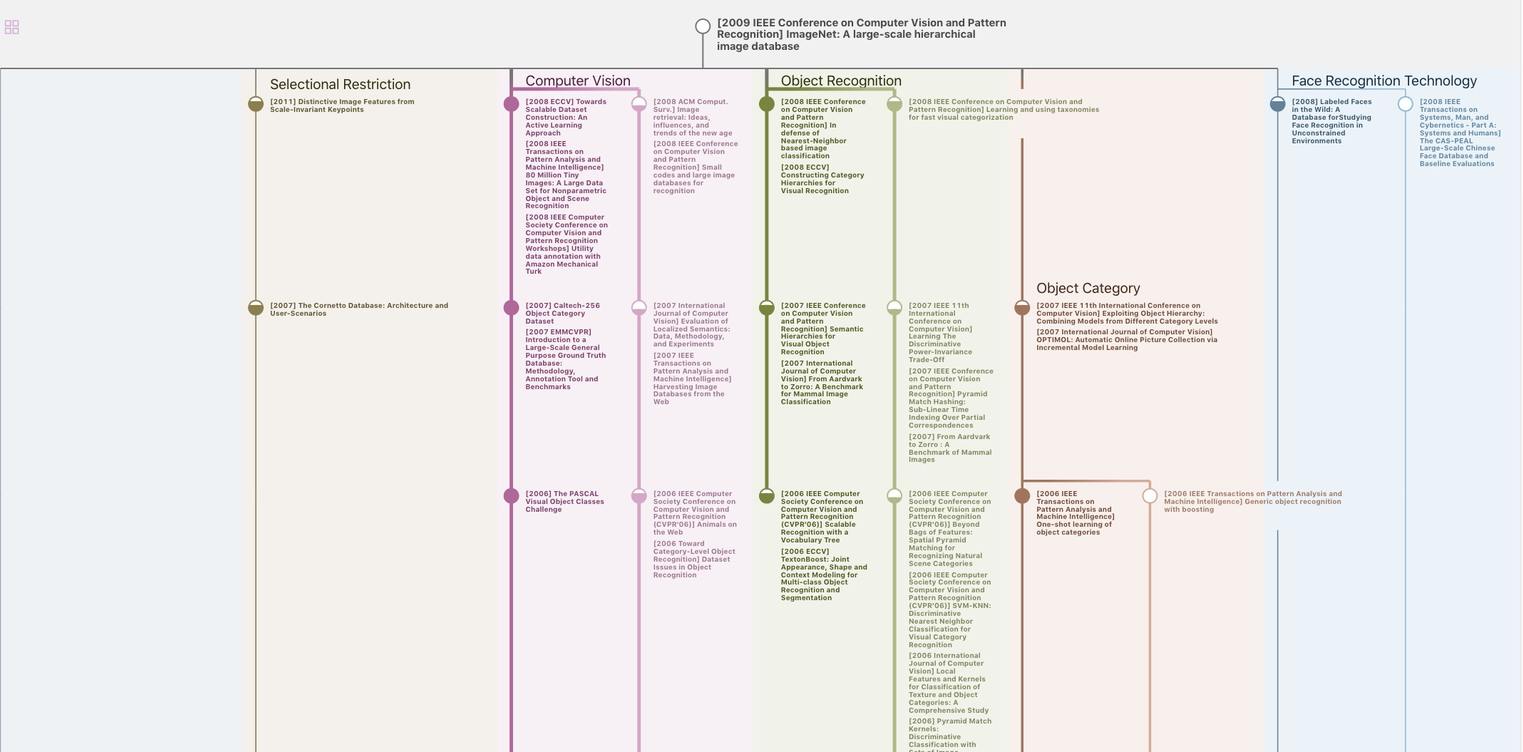
生成溯源树,研究论文发展脉络
Chat Paper
正在生成论文摘要