Iterative Graph Self-Distillation
IEEE TRANSACTIONS ON KNOWLEDGE AND DATA ENGINEERING(2024)
Abstract
Recently, there has been increasing interest in the challenge of how to discriminatively vectorize graphs. To address this, we propose a method called Iterative Graph Self-Distillation (IGSD) which learns graph-level representation in an unsupervised manner through instance discrimination using a self-supervised contrastive learning approach. IGSD involves a teacher-student distillation process that uses graph diffusion augmentations and constructs the teacher model using an exponential moving average of the student model. The intuition behind IGSD is to predict the teacher network representation of the graph pairs under different augmented views. As a natural extension, we also apply IGSD to semi-supervised scenarios by jointly regularizing the network with both supervised and self-supervised contrastive loss. Finally, we show that fine-tuning the IGSD-trained models with self-training can further improve graph representation learning. Empirically, we achieve significant and consistent performance gain on various graph datasets in both unsupervised and semi-supervised settings, which well validates the superiority of IGSD.
MoreTranslated text
Key words
Task analysis,Representation learning,Kernel,Graph neural networks,Iterative methods,Data augmentation,Training,graph representation learning,self-supervised learning
AI Read Science
Must-Reading Tree
Example
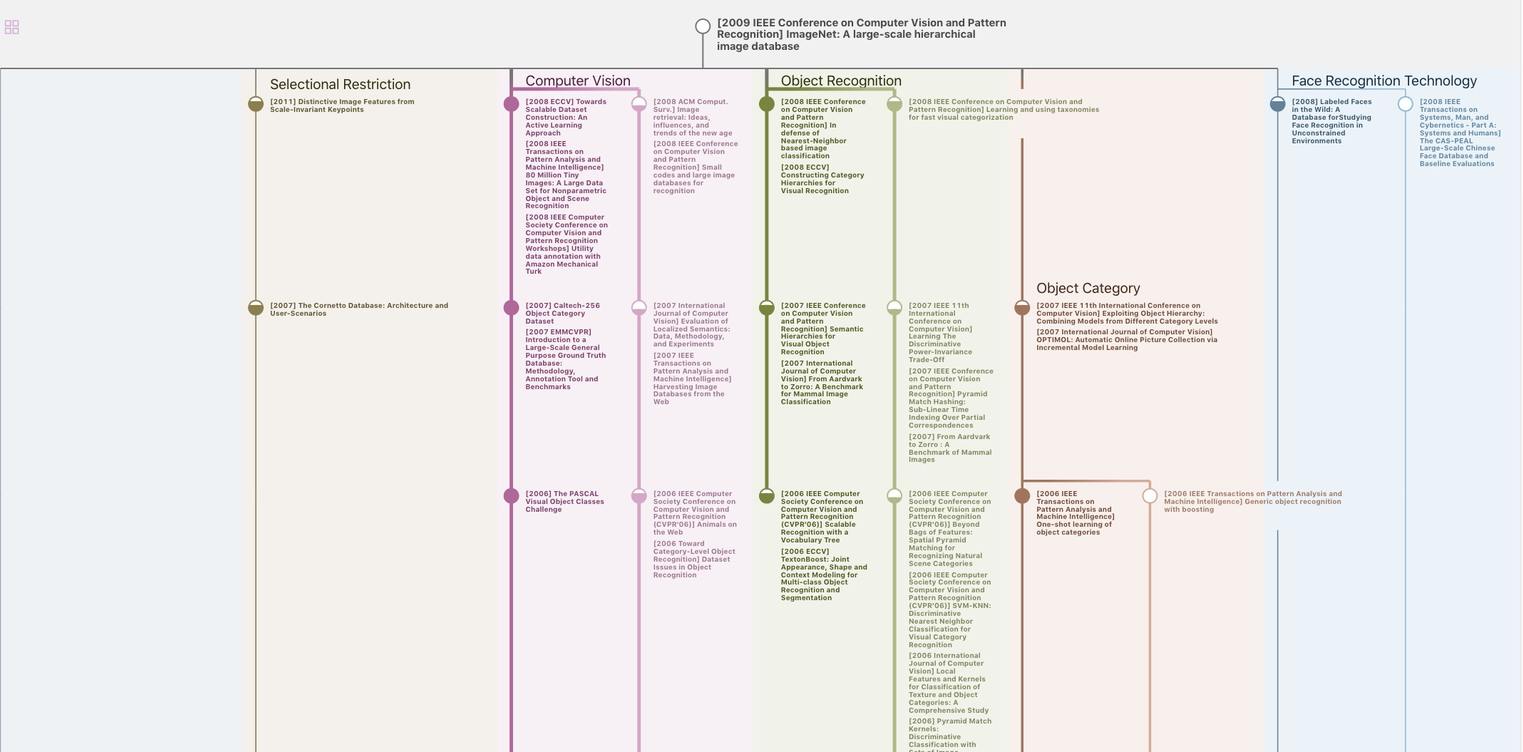
Generate MRT to find the research sequence of this paper
Chat Paper
Summary is being generated by the instructions you defined